An Alzheimer’s disease classification model using transfer learning Densenet with embedded healthcare decision support system
Decision Analytics Journal(2023)
摘要
Training a Convolutional Neural Network (CNN) from scratch is time-consuming and expensive. In this study, we propose implementing the DenseNet architecture for classification of AD in three classes. Our approach leverages transfer learning architectures as the base model and showcases superior performance on the MRI dataset compared to other techniques. We use a variety of methodologies to provide a thorough study of our model. We first create a performance baseline without data augmentation, addressing the difficulties in classifying Alzheimer’s disease (AD) caused by high-dimensional MRI brain scans. The improved model performance obtained through data augmentation is then highlighted, demonstrating its effectiveness in handling sparse data and assisting in generalization. We also investigate the impact of omitting particular transformations and modifying dataset split ratios, providing more insights into the behavior of the model. Through comprehensive evaluation, we demonstrate that our proposed system model achieves an accuracy of 96.5% and an impressive AUC of 99%, surpassing previous methods. This study mainly highlights the effectiveness of DenseNet architecture, current study limitations and future recommendation. Moreover, incorporating a healthcare decision support system further aid in providing valuable insights for AD diagnosis and decision-making in clinical settings.
更多查看译文
关键词
disease classification model,healthcare decision support system,alzheimers,healthcare decision
AI 理解论文
溯源树
样例
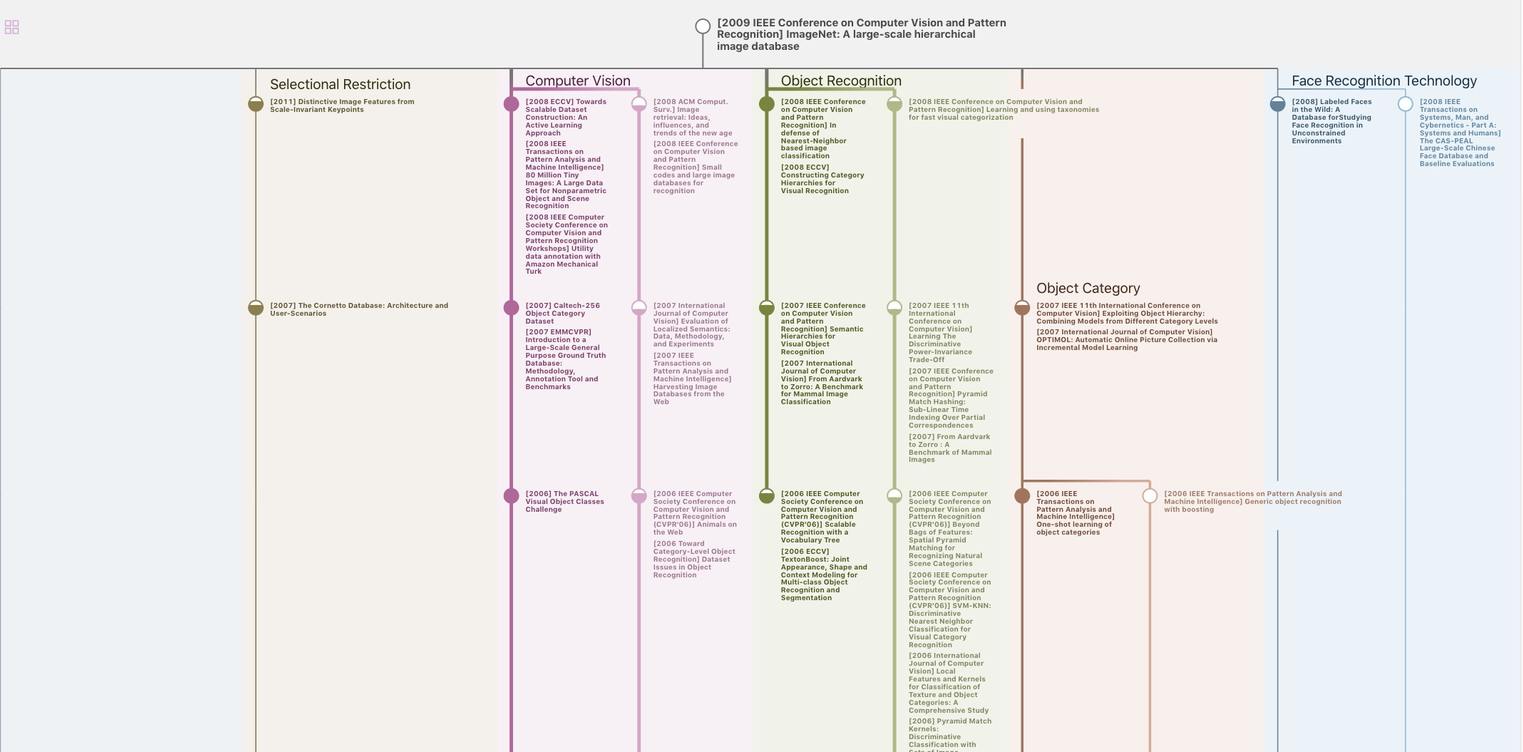
生成溯源树,研究论文发展脉络
Chat Paper
正在生成论文摘要