STOP: Joint send buffer and transmission control for user-perceived deadline guarantee via curriculum guided-deep reinforcement learning
JOURNAL OF NETWORK AND COMPUTER APPLICATIONS(2024)
摘要
Real-time applications with strict user-perceived deadline requirements, such as cloud virtual reality (VR) gaming and high-frequency trading, have experienced substantial growth in recent years. To ensure the user perceived deadline at the transport layer, i.e., the network transmission delay and the waiting delay in the send buffer, transmission control scheme plays an important role. While several delay-sensitive transmission control schemes have been proposed, none of them consider the impact of waiting delay in the send buffer. To address this issue, we propose STOP, a joint send buffer and congestion window (CWND) control approach for guaranteeing user-perceived deadline via curriculum-guided deep reinforcement learning. To obtain optimal joint control policy, STOP utilizes a reinforcement learning-based algorithm to jointly adjust the CWND and send buffer with respect to the send buffer state, the network condition and the deadline requirement. Furthermore, to enhance the generalization capability of reinforcement learning-based algorithm, STOP incorporates curriculum learning during the training phase with a difficulty measurer and training scheduler. Extensive experiments in NS-3 indicate that the STOP scheme achieves an overwhelmingly higher average arrival ratio, i.e., around 95% vs. around 0.2%, compared with current prevalent transmission control scheme such as BBR, Cubic and Vegas.
更多查看译文
关键词
Deadline-aware,Transmission control,Deep reinforcement learning,Send buffer,Curriculum learning,Joint control
AI 理解论文
溯源树
样例
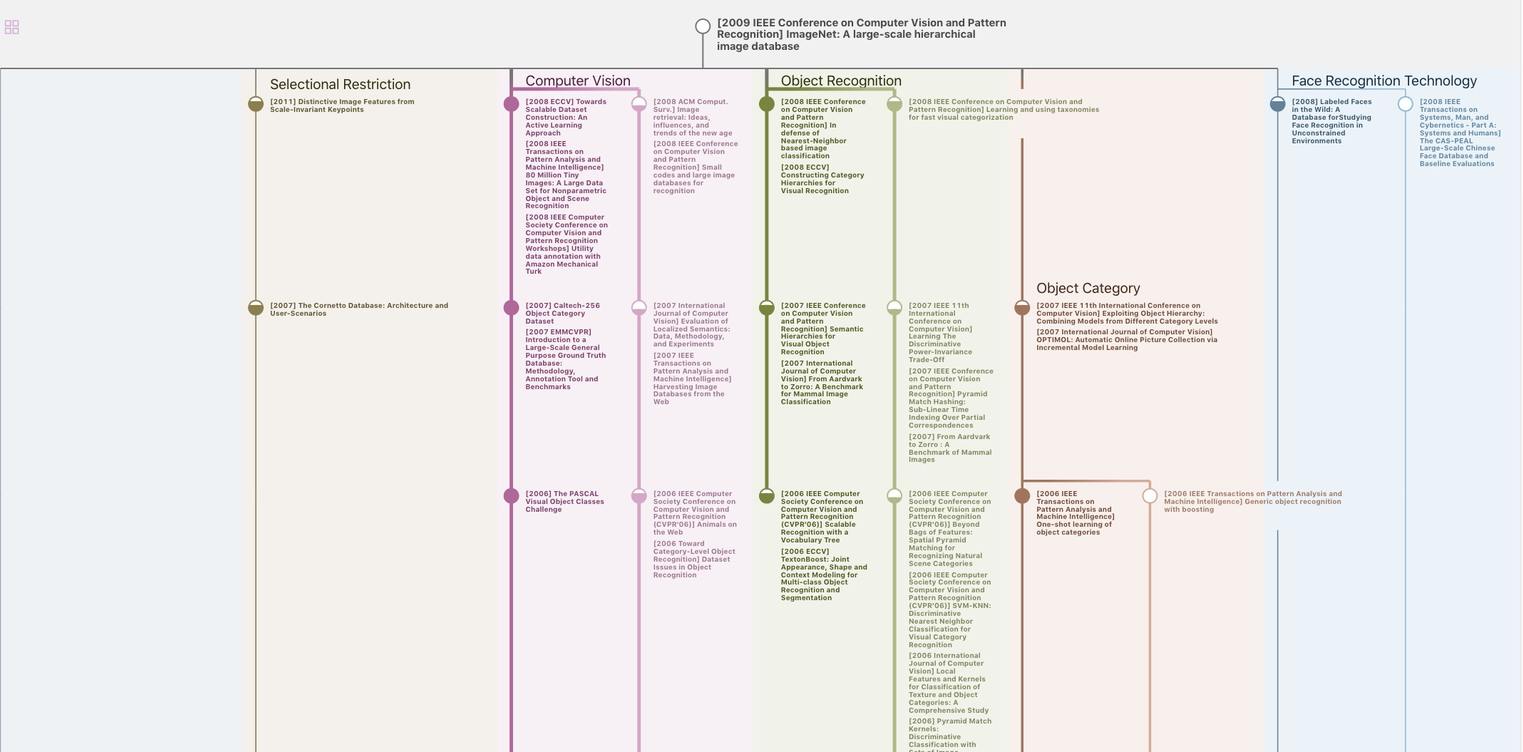
生成溯源树,研究论文发展脉络
Chat Paper
正在生成论文摘要