Localization model and rank-based features selection approach for the classification of GGO and consolidation stages of COVID-19
EXPERT SYSTEMS WITH APPLICATIONS(2024)
摘要
Diseases like Middle East respiratory syndrome (MERS) and severe acute respiratory syndrome (SARS) have been caused by a large family virus known as Coronavirus. High-resolution computed tomography (HRCT) chest is used as a useful tool for the triage and stratification of COVID-19-suspected patients having respiratory symptoms until they are confirmed by polymerase chain reaction (PCR) test. To further expedite the reporting of HRCT chest in a short time, computer-aided detection algorithms can be more useful. In this work, a method is proposed that consists of three phases including COVID-19 classification, localization, and segmentation. The COVID-19-ResNet-50 model is designed for COVID-19 classification using HRCT slices. The features are obtained from a pre-trained ResNet-50 model, and the process of identifying the most pertinent features is carried out using the Wilcoxon Separability Criteria (WSC). The linear discriminant (LD) classifier is used to classify COVID19 providing an accuracy of 0.99. To localize the COVID-19-infected region, the classified HRCT slices are fed as input to an open neural network exchange (ONNX) that is a backbone of the Tiny-You Look at Once (YOLO)-v2 model. The proposed COVID-19-YOLOv2 model localized the COVID-19 lesions with 0.948 intersections over union (IoU). A three-dimensional semantic neural network comprising 10 selected layers is proposed whose training based on the best-selected hyperparameters is performed, it accurately segments the localized infected regions having an 0.98 IoU. The proposed method is accessed on national and international datasets that provide improved performance as compared to the most recent works published in this domain.
更多查看译文
关键词
YOLOv2,ResNet50,Linear discriminant (LD),Separability criteria,HRCT
AI 理解论文
溯源树
样例
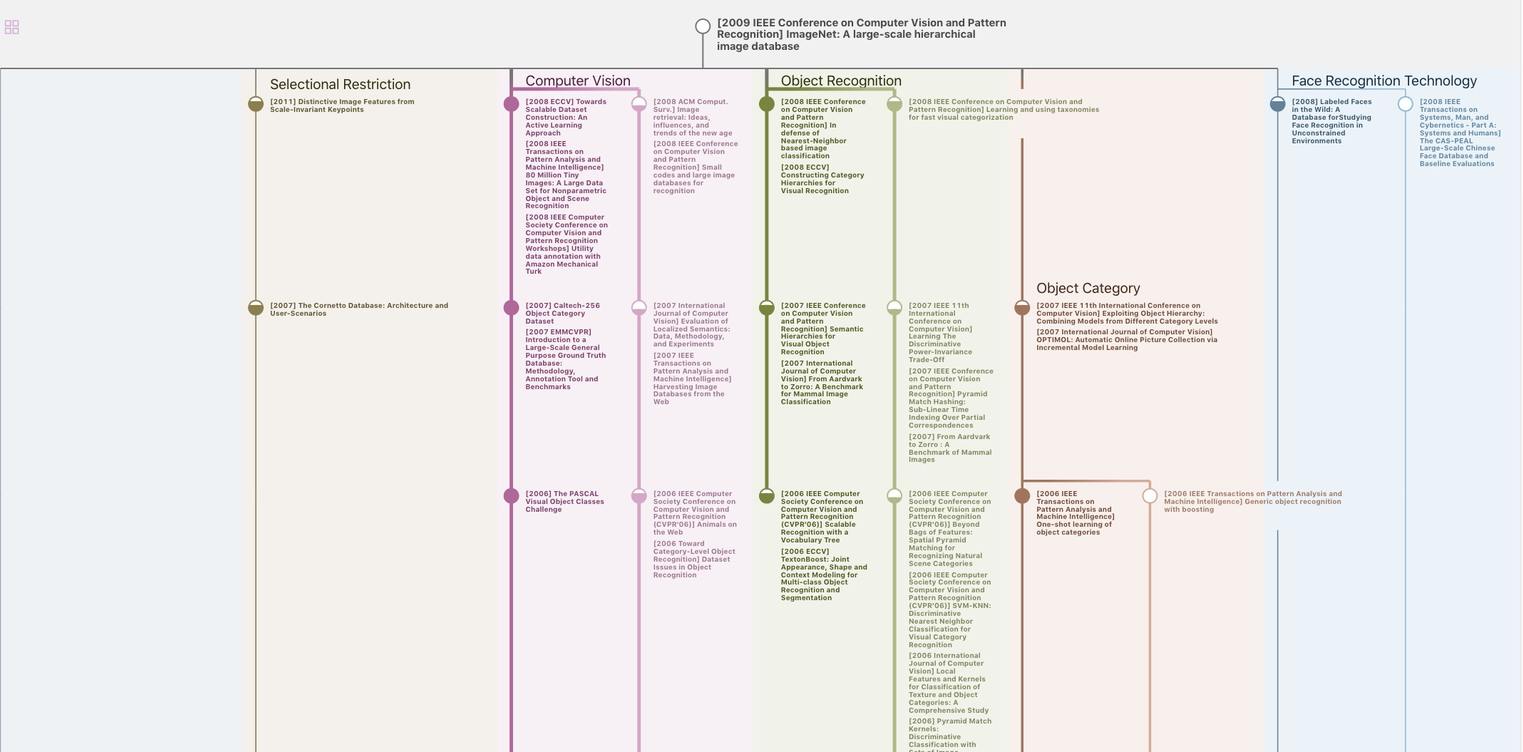
生成溯源树,研究论文发展脉络
Chat Paper
正在生成论文摘要