Incorporating Structural Information into Legal Case Retrieval
ACM TRANSACTIONS ON INFORMATION SYSTEMS(2024)
摘要
Legal case retrieval has received increasing attention in recent years. However, compared to ad hoc retrieval tasks, legal case retrieval has its unique challenges. First, case documents are rather lengthy and contain complex legal structures. Therefore, it is difficult for most existing dense retrieval models to encode an entire document and capture its inherent complex structure information. Most existing methods simply truncate part of the document content to meet the input length limit of PLMs, which will lead to information loss. Additionally, the definition of relevance in the legal domain differs from that in the general domain. Previous semantic-based or lexical-based methods fail to provide a comprehensive understanding of the relevance of legal cases. In this article, we propose a Structured Legal case Retrieval (SLR) framework, which incorporates internal and external structural information to address the above two challenges. Specifically, to avoid the truncation of long legal documents, the internal structural information, which is the organization pattern of legal documents, can be utilized to split a case document into segments. By dividing the document-level semantic matching task into segment-level subtasks, SLR can separately process segments using different methods based on the characteristic of each segment. In this way, the key elements of a case document can be highlighted without losing other content information. Second, toward a better understanding of relevance in the legal domain, we investigate the connections between criminal charges appearing in large-scale case corpus to generate a chargewise relation graph. Then, the similarity between criminal charges can be pre-computed as the external structural information to enhance the recognition of relevant cases. Finally, a learning-to-rank algorithm integrates the features collected from internal and external structures to output the final retrieval results. Experimental results on public legal case retrieval benchmarks demonstrate the superior effectiveness of SLR over existing state-of-the-art baselines, including traditional bag-of-words and neural-based methods. Furthermore, we conduct a case study to visualize how the proposed model focuses on key elements and improves retrieval performance.
更多查看译文
关键词
Legal case retrieval,structural information,relevance
AI 理解论文
溯源树
样例
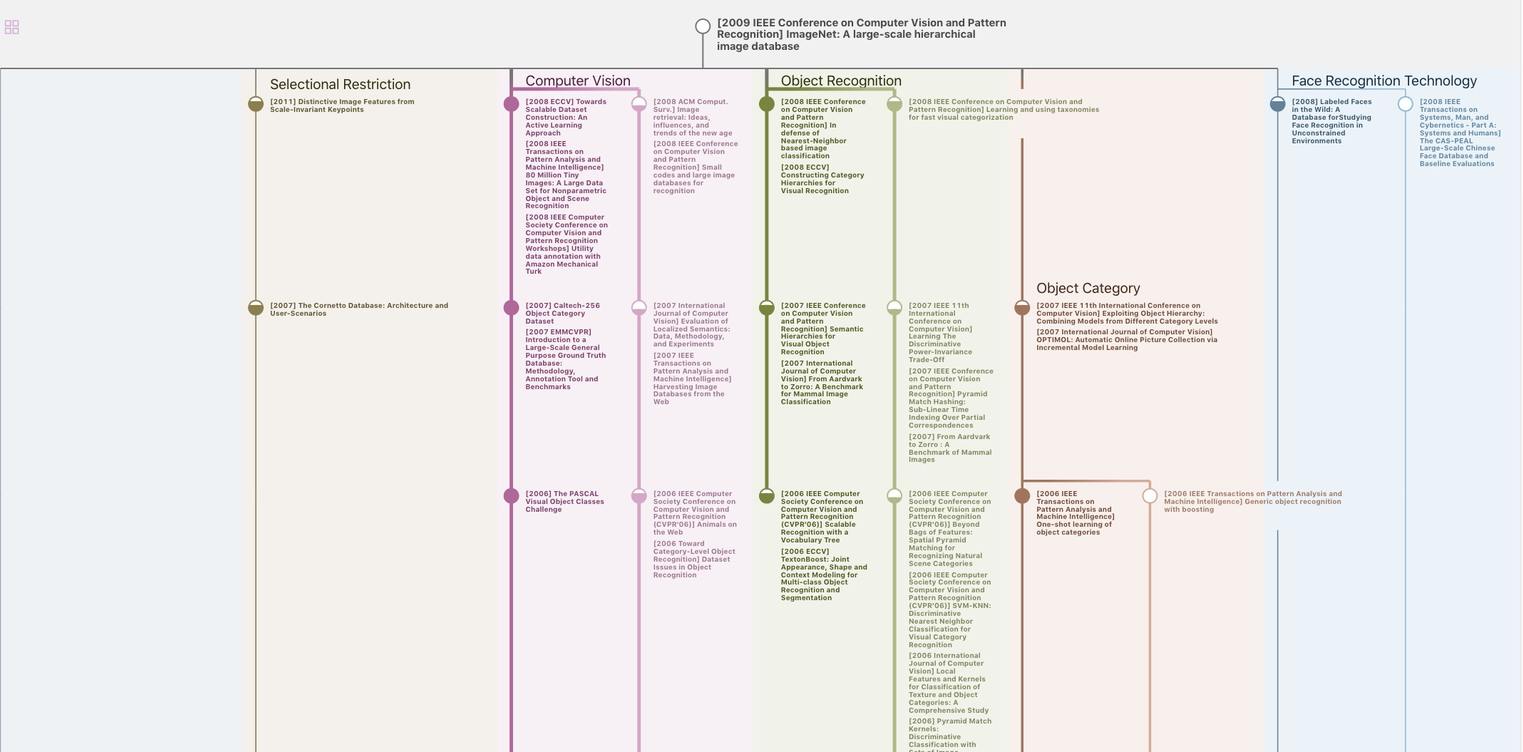
生成溯源树,研究论文发展脉络
Chat Paper
正在生成论文摘要