Forecasting climate transition regulatory and market risk variables with machine learning
2023 IEEE INTERNATIONAL SYMPOSIUM ON TECHNOLOGY AND SOCIETY, ISTAS(2023)
摘要
The financial impacts of the consequences of climate change on organisations are not always clear. Therefore, for many organisations, assessing the potential impact of climate risk remains a challenge. This piece of research is geared towards selecting the best artificial intelligence technique for modelling and forecasting the operational costs related to climate transition risk, such as direct or indirect utility consumption, including the costs of electricity and fossil fuels. These costs can be affected, especially by regulatory and market risks that are part of transition risk. We train 17 deep learning and machine learning models to forecast electricity and diesel consumption indicators, as well as the corresponding prices of these variables. Our results show an average prediction accuracy of 90.36% and emphasise the importance of using such decision support tools to analyse the financial impacts of climate risks within organisations. This calls for organisations to improve data collection and availability, as performing this kind of analysis is promising but currently remains a challenge due to the scarcity of available information.
更多查看译文
关键词
Climate transition risk,climate risk,artificial intelligence,machine learning,deep learning,forecast
AI 理解论文
溯源树
样例
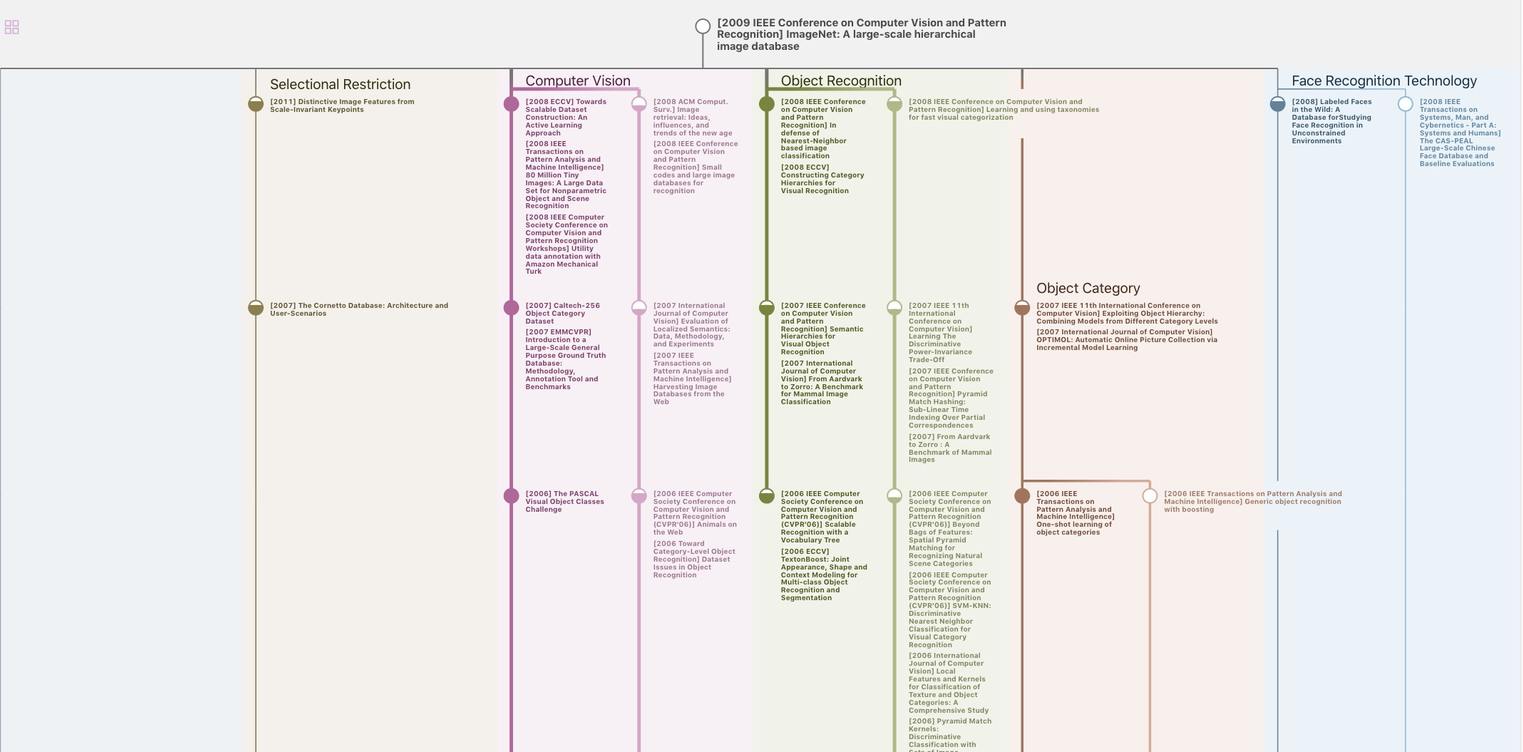
生成溯源树,研究论文发展脉络
Chat Paper
正在生成论文摘要