Efficient Edge Data Management Framework for IIoT via Prediction-Based Data Reduction
IEEE Transactions on Parallel and Distributed Systems(2023)
摘要
Large amounts of time series data are required to support data analysis at the edge in the end-edge-cloud Industrial Internet of Things (IIoT) architecture. Reducing the storage cost is one of the main challenges in edge data management due to the limited storage resource of edge nodes. The state-of-the-art data reduction method has a high time overhead and poor reduction efficiency for unstable data sets. To solve this problem, this study proposes a time-series data management framework that combines data partition and data compression techniques. For the data partition technique, we propose an adaptive selection strategy to integrate the access pattern of the application and the characteristics of the time series data, thereby improving the partition accuracy. For the data compression technique, we propose a compression scheme based on time series data segmentation by using the idea of divide and conquer; we further introduce a change point detection technique to improve the compression efficiency for unstable data sets. Experimental results obtained with three types of real industrial data sets show that our framework is significantly better than the state-of-the-art method in terms of compression ratio and time overhead.
更多查看译文
关键词
Industrial Internet of Things,Image edge detection,Data compression,Computer architecture,Time series analysis,Real-time systems,Streams,Data reduction,edge data management framework,industrial internet of things (IIoT)
AI 理解论文
溯源树
样例
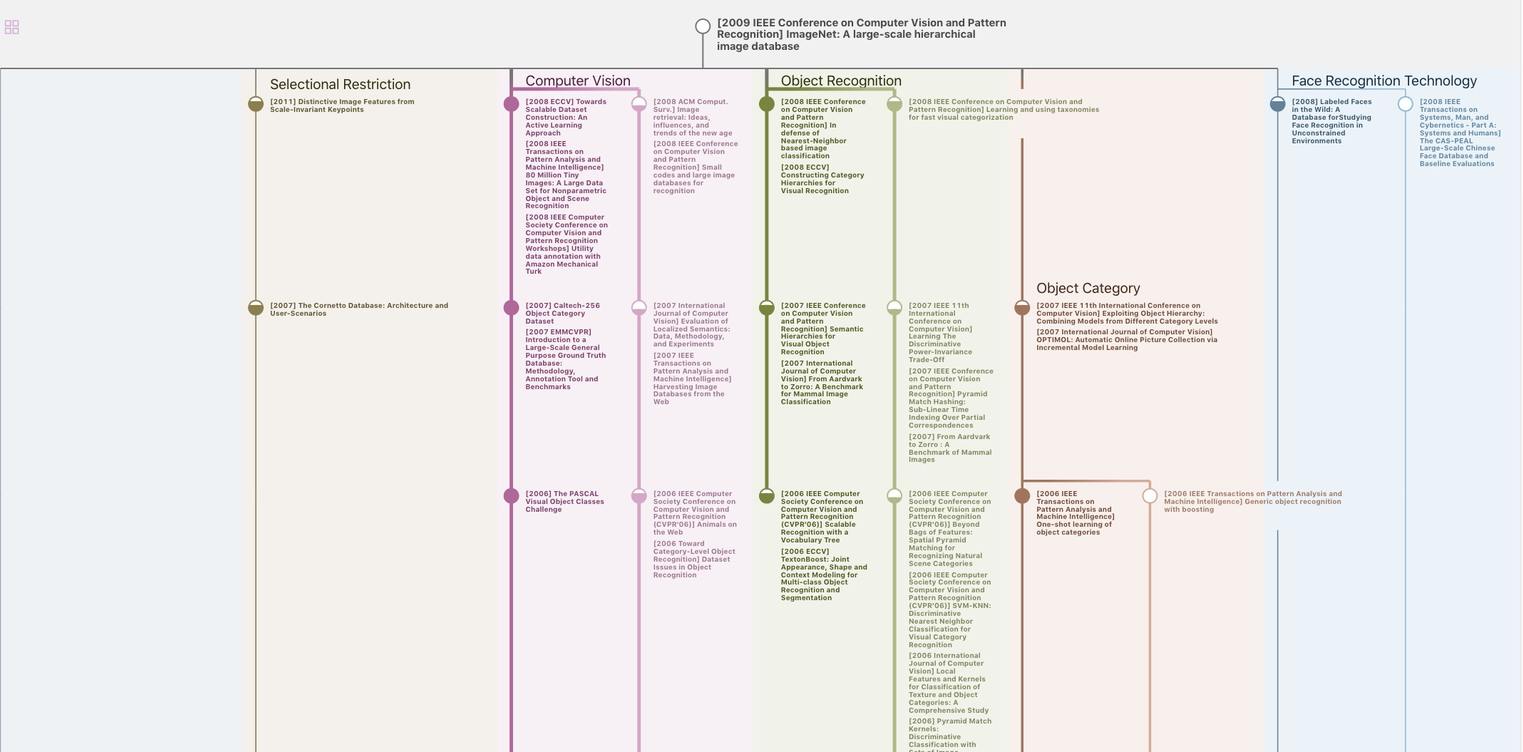
生成溯源树,研究论文发展脉络
Chat Paper
正在生成论文摘要