Shuffle SwishNet-181: COVID-19 diagnostic framework using ECG images
MULTIMEDIA TOOLS AND APPLICATIONS(2023)
摘要
Early and precise detection of COVID-19 holds significant benefits, particularly in facilitating the prompt isolation of infected individuals and helping to control the spread of this disease as vaccinated persons also got infected from COVID-19. The research community has explored various COVID-19 diagnostic tools operated on different imaging modalities like X-rays and CT scans apart from conventional PCR testing which often takes several hours to get the results. Existing studies on ECG-based COVID-19 diagnostics are limited even though this modality is quickly available as compared to CT scans and X-rays. Moreover, our preliminary analysis suggests that ECG images can also be used to study the correlation of COVID-19 with cardio diseases, which is not possible in the case of X-rays and CT scans. Moreover, current ECG-based COVID-19 diagnostics approaches often report an issue of low detection accuracy and focus more on binary classification. To overcome these challenges, we developed an effective COVID-19 diagnostic tool by proposing a novel Shuffle SwishNet-181 deep learning-based model. During the pre-processing, the background is subtracted from the signals and combined these signals in a hexaxial way. Shuffle SwishNet-181 extracts the distinctive deep features and accurately classifies the ECG images into COVID-19, normal, myocardial infarction (MI), abnormal heartbeat patients (HB), and patients who have a history of myocardial infarction (PMI). Moreover, the Score-cam technique is employed to visualize the working of the proposed model by showing the top priority features extracted by the Shuffle SwishNet-181 model. The rigorous experimentation is performed on a publicly available ECG imaging dataset to demonstrate the effectiveness of the COVID-19 diagnostic framework. The proposed model achieved an accuracy of 99% in the case of COVID-19 vs. Normal, 99.4% in the case of COVID-19 vs. MI, 98.8% in the case of COVID-19 vs. HB, and 98.7% in the case of COVID-19 vs. PMI. For multiclass classification, the proposed model achieved 91.6% accuracy. Experimental results show the reliability of the method for binary and multi-class classification of COVID-19. Explainability analysis proved that the proposed model precisely focuses on salient features for classification.
更多查看译文
关键词
COVID-19 detection,Cardiovascular patients,ECG,Shuffle SwishNet-181
AI 理解论文
溯源树
样例
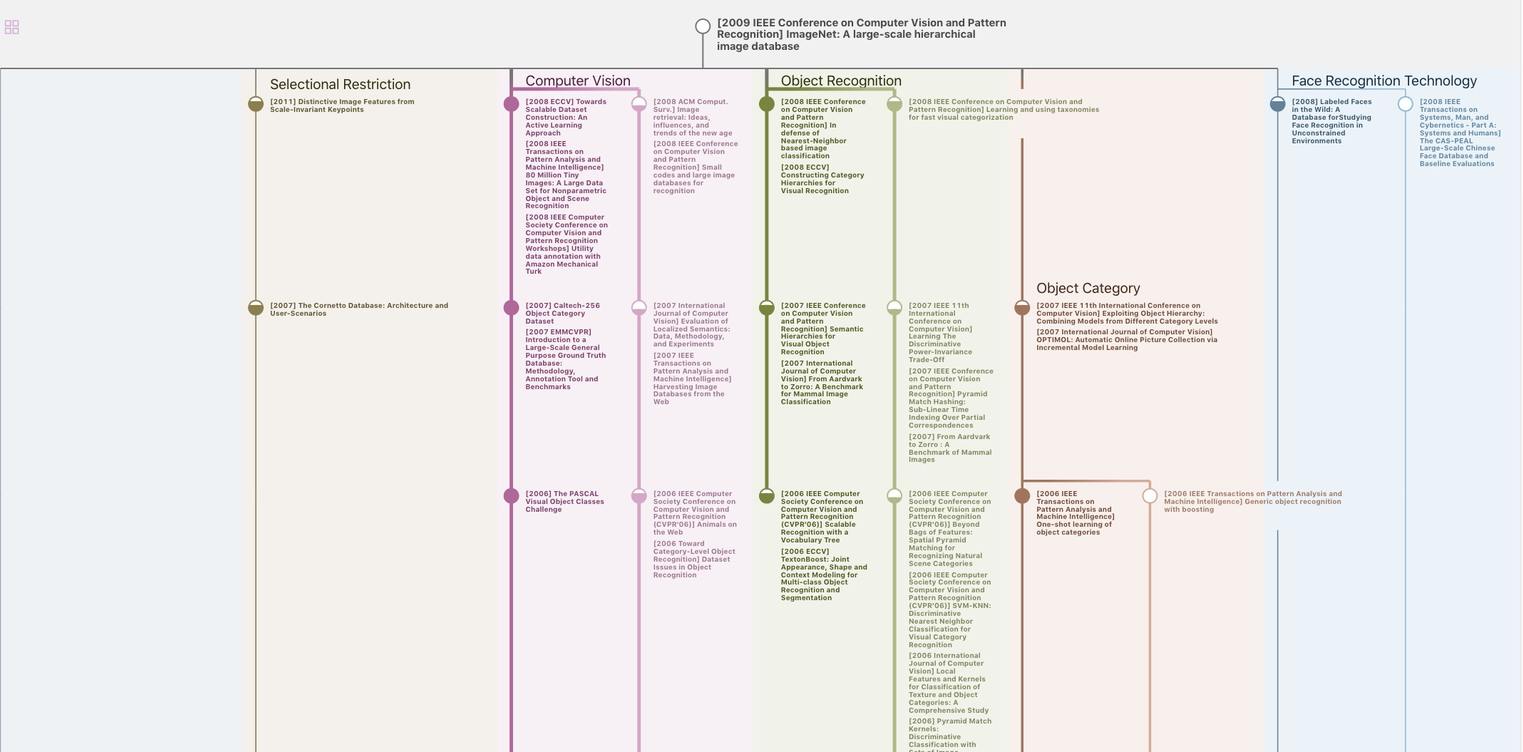
生成溯源树,研究论文发展脉络
Chat Paper
正在生成论文摘要