MAUNext: a lightweight segmentation network for medical images
PHYSICS IN MEDICINE AND BIOLOGY(2023)
摘要
Objective. The primary objective of this study is to enhance medical image segmentation techniques for clinical research by prioritizing accuracy and the number of parameters. Approach. To achieve this objective, a novel codec-based MAUNext approach is devised, focusing on lightweight backbone design and the integration of skip connections utilizing multiscale, attention mechanism, and other strategic components. The approach is composed of three core modules: a multi-scale attentional convolution module for improved accuracy and parameter reduction, a collaborative neighbourhood-attention MLP encoding module to enhance segmentation performance, and a tiny skip-connected cross-layer semantic fusion module to bridge the semantic gap between encoder and decoder. Main results. The study extensively evaluates the MAUNext approach alongside eight state-of-the-art methods on three renowned datasets: Kagglelung, ISIC, and Brain. The experimental outcomes robustly demonstrate that the proposed approach surpasses other methods in terms of both parameter numbers and accuracy. This achievement holds promise for effectively addressing medical image segmentation tasks. Significance. Automated medical image segmentation, particularly in organ and lesion identification, plays a pivotal role in clinical diagnosis and treatment. Manual segmentation is resource-intensive, thus automated methods are highly valuable. The study underscores the clinical significance of automated segmentation by providing an advanced solution through the innovative MAUNext approach. This approach offers substantial improvements in accuracy and efficiency, which can significantly aid clinical decision-making and patient treatment.
更多查看译文
关键词
lightweight network,medical image segmentation,deep learning method
AI 理解论文
溯源树
样例
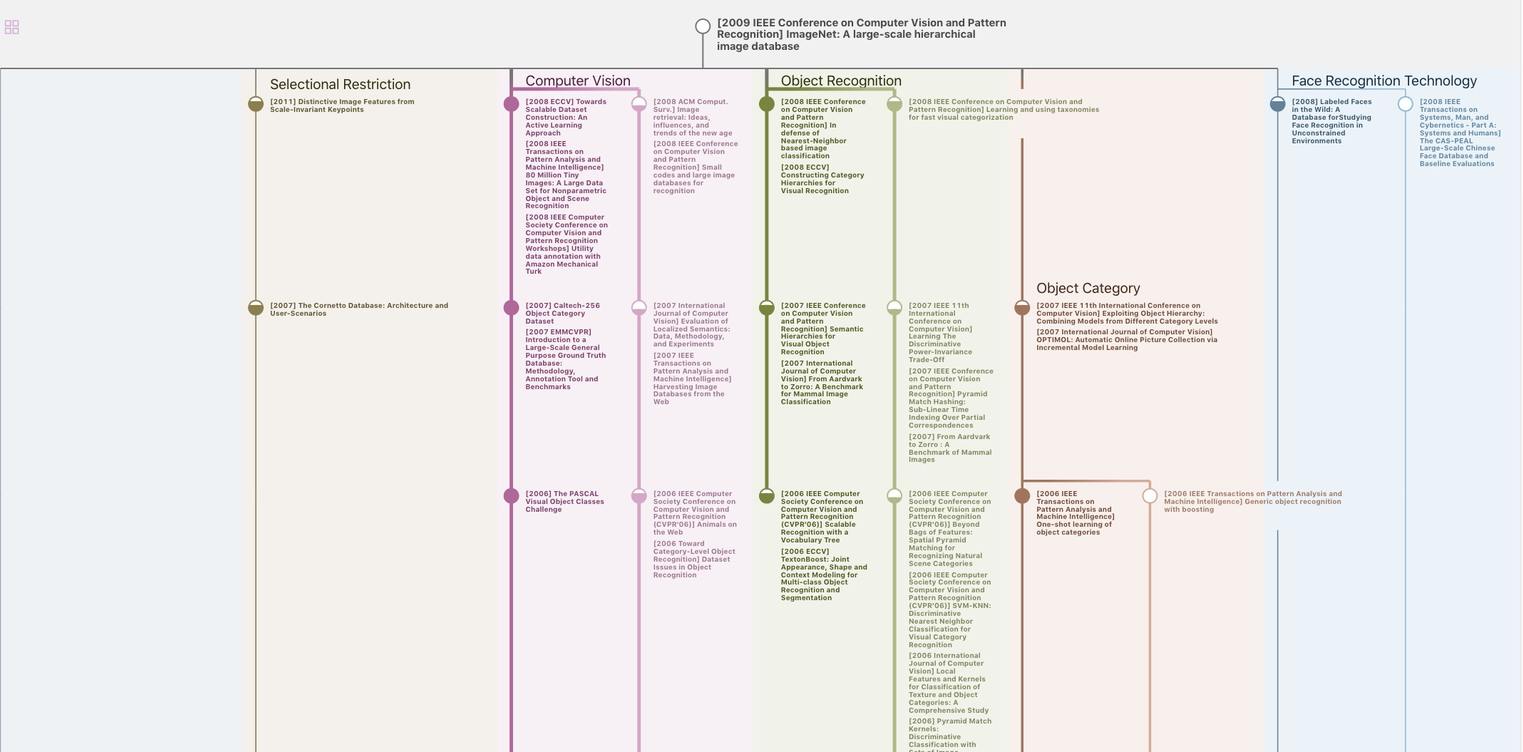
生成溯源树,研究论文发展脉络
Chat Paper
正在生成论文摘要