Image Captioning With Controllable and Adaptive Length Levels
IEEE TRANSACTIONS ON PATTERN ANALYSIS AND MACHINE INTELLIGENCE(2024)
摘要
Image captioning is a core challenge in computer vision, attracting significant attention. Traditional methods prioritize caption quality, often overlooking style control. Our research enhances method controllability, enabling descriptions of varying detail. By integrating a length level embedding into current models, they can produce detailed or concise captions, increasing diversity. We introduce a length-level reranking transformer to correlate image and text complexity, optimizing caption length for informativeness without redundancy. Additionally, with caption length increase, computational complexity grows due to the autoregressive (AR) design of existing methods. To address this, our non-autoregressive (NAR) model maintains constant complexity regardless of caption length. We've developed a training approach that includes refinement sequence training and sequence-level knowledge distillation to close the performance gap between NAR and AR models. In testing, our models set new standards for caption quality on the MS COCO dataset and offer enhanced controllability and diversity. Our NAR model excels over AR models in these aspects and shows greater efficiency with longer captions. With advanced training techniques, our NAR's caption quality rivals that of leading AR models.
更多查看译文
关键词
Length-controllable image captioning,non-autoregressive image captioning,length level reranking,refinement-enhanced sequence training
AI 理解论文
溯源树
样例
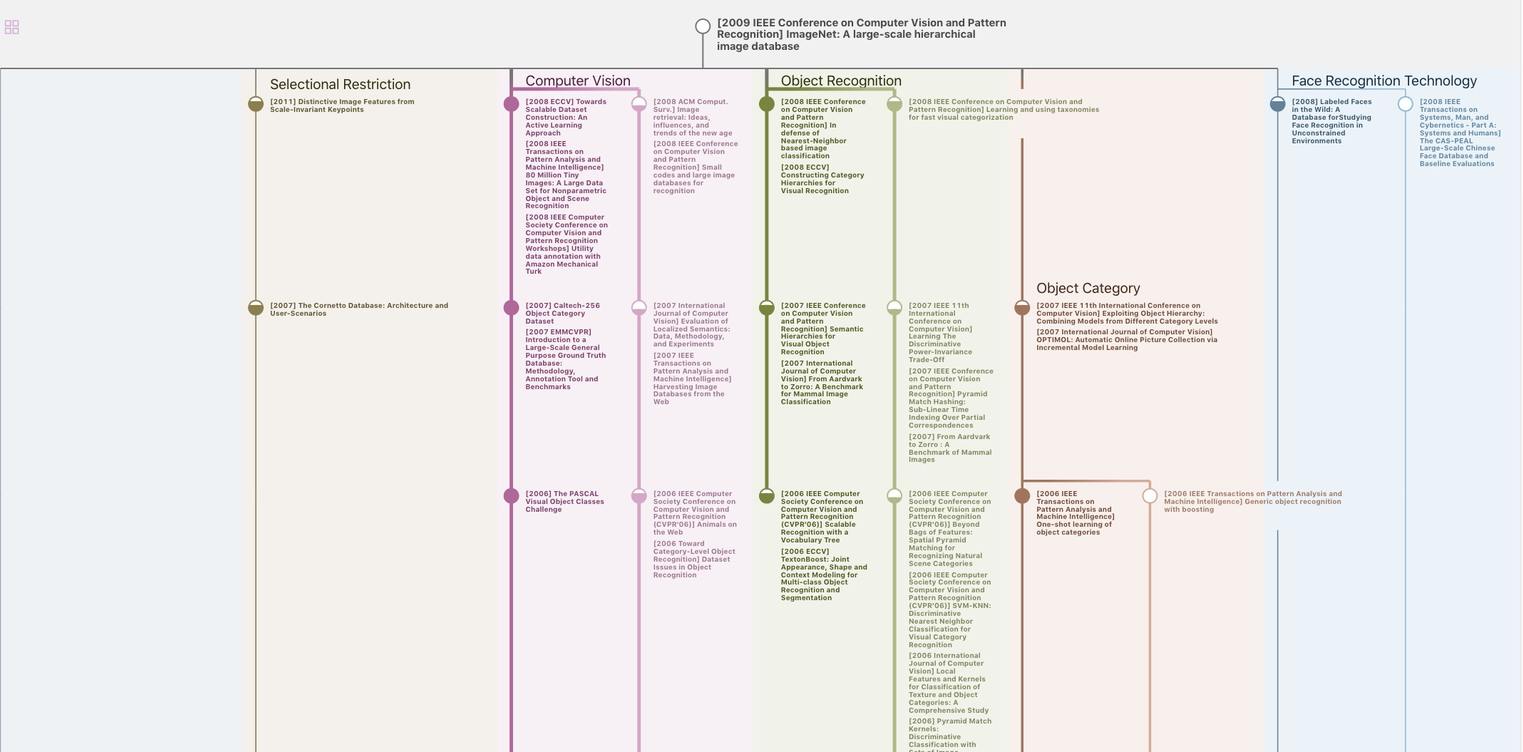
生成溯源树,研究论文发展脉络
Chat Paper
正在生成论文摘要