Artificial CT images can enhance variation of case images in diagnostic radiology skills training
INSIGHTS INTO IMAGING(2023)
摘要
Objectives We sought to investigate if artificial medical images can blend with original ones and whether they adhere to the variable anatomical constraints provided. Methods Artificial images were generated with a generative model trained on publicly available standard and low-dose chest CT images (805 scans; 39,803 2D images), of which 17% contained evidence of pathological formations (lung nodules). The test set (90 scans; 5121 2D images) was used to assess if artificial images (512 × 512 primary and control image sets) blended in with original images, using both quantitative metrics and expert opinion. We further assessed if pathology characteristics in the artificial images can be manipulated. Results Primary and control artificial images attained an average objective similarity of 0.78 ± 0.04 (ranging from 0 [entirely dissimilar] to 1[identical]) and 0.76 ± 0.06, respectively. Five radiologists with experience in chest and thoracic imaging provided a subjective measure of image quality; they rated artificial images as 3.13 ± 0.46 (range of 1 [unrealistic] to 4 [almost indistinguishable to the original image]), close to their rating of the original images (3.73 ± 0.31). Radiologists clearly distinguished images in the control sets (2.32 ± 0.48 and 1.07 ± 0.19). In almost a quarter of the scenarios, they were not able to distinguish primary artificial images from the original ones. Conclusion Artificial images can be generated in a way such that they blend in with original images and adhere to anatomical constraints, which can be manipulated to augment the variability of cases. Critical relevance statement Artificial medical images can be used to enhance the availability and variety of medical training images by creating new but comparable images that can blend in with original images. Key points • Artificial images, similar to original ones, can be created using generative networks. • Pathological features of artificial images can be adjusted through guiding the network. • Artificial images proved viable to augment the depth and broadening of diagnostic training. Graphical Abstract
更多查看译文
关键词
Artificial image,Artificial intelligence,Radiology,Medical image education,Personalized education
AI 理解论文
溯源树
样例
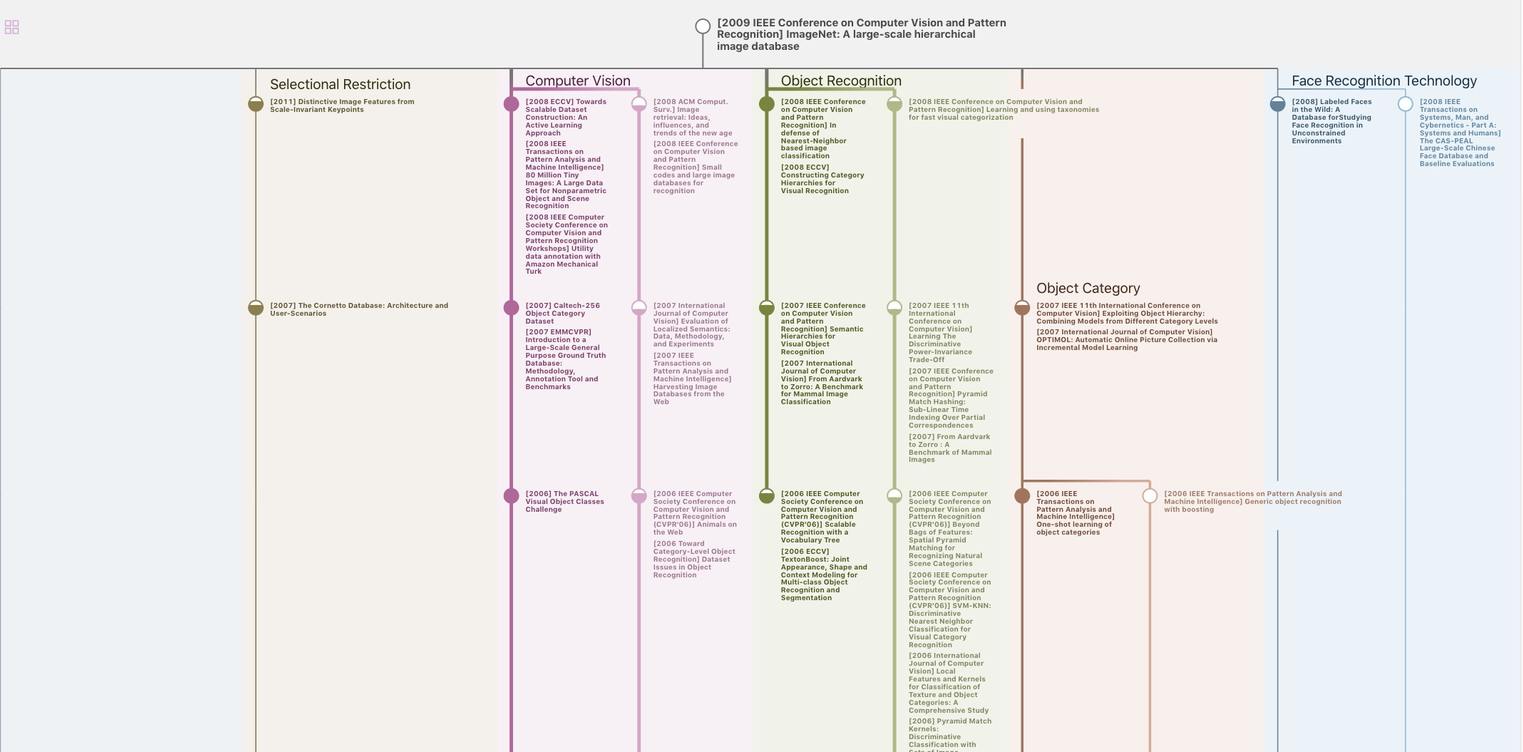
生成溯源树,研究论文发展脉络
Chat Paper
正在生成论文摘要