Improving the out-of-sample generalization ability of data-driven chiller performance models using physics-guided neural network
APPLIED ENERGY(2024)
摘要
Modeling of the chiller performance is essential for the implementation of optimal energy-efficient control strategies in a heating, ventilation, and air conditioning (HVAC) system. Though classical data-driven chiller performance models are widely adopted in the industry, they generally suffer from poor out-of-sample generalization abilities, which refers to the model's capability to extrapolate for new data outside the range of the training dataset. In practice, however, the available chiller operation data for model development are often insufficient or collected from a few limited operating conditions, such that extrapolation is unavoidable after the model is applied for control purposes. To deal with this issue, this paper proposed a physics-guided neural network (PGNN) to model the energy performance of chillers. By adopting a new neural network architecture, modifying the loss function, and adding limited out-of-sample data, the PGNN incorporates domain knowledge into the data-driven model to achieve better out-of-sample generalization performance. Meanwhile, the convexity and monotonicity between the dependent and independent variables in the PGNN are properly addressed. The proposed PGNN is applied to model the chiller serving a high-rise building, and results show that PGNN performs much better in extrapolation than classical models and the multi-layer perceptron model. The research demonstrated the usefulness and effectiveness of the PGNN in modeling HVAC equipment.
更多查看译文
关键词
Chiller model,Generalization ability,Artificial intelligence,Physics-guided neural network,Input convex neural network,Loss function
AI 理解论文
溯源树
样例
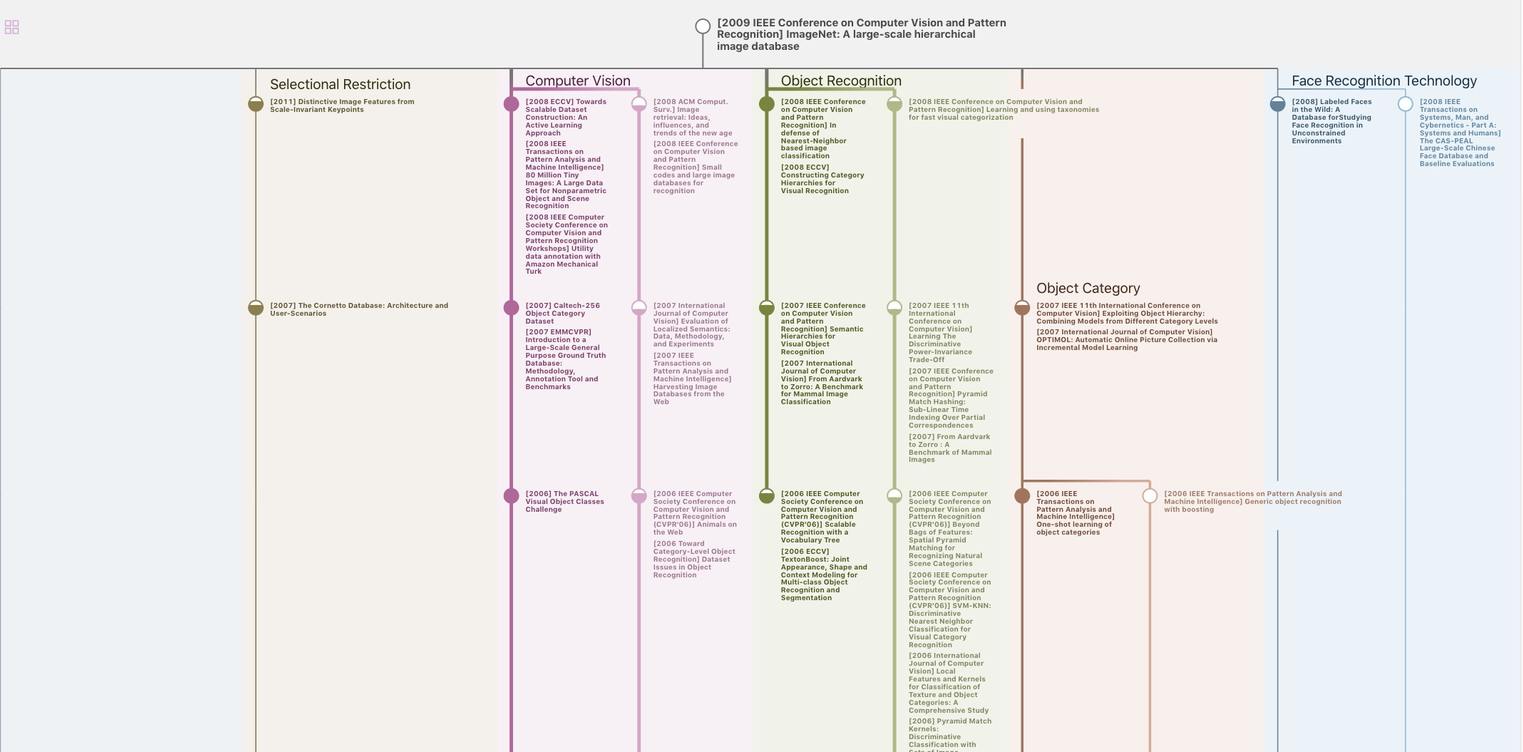
生成溯源树,研究论文发展脉络
Chat Paper
正在生成论文摘要