CBSiMT: Mitigating Hallucination in Simultaneous Machine Translation with Weighted Prefix-to-Prefix Training.
CoRR(2023)
摘要
Simultaneous machine translation (SiMT) is a challenging task that requires starting translation before the full source sentence is available. Prefix-to-prefix framework is often applied to SiMT, which learns to predict target tokens using only a partial source prefix. However, due to the word order difference between languages, misaligned prefix pairs would make SiMT models suffer from serious hallucination problems, i.e. target outputs that are unfaithful to source inputs. Such problems can not only produce target tokens that are not supported by the source prefix, but also hinder generating the correct translation by receiving more source words. In this work, we propose a Confidence-Based Simultaneous Machine Translation (CBSiMT) framework, which uses model confidence to perceive hallucination tokens and mitigates their negative impact with weighted prefix-to-prefix training. Specifically, token-level and sentence-level weights are calculated based on model confidence and acted on the loss function. We explicitly quantify the faithfulness of the generated target tokens using the token-level weight, and employ the sentence-level weight to alleviate the disturbance of sentence pairs with serious word order differences on the model. Experimental results on MuST-C English-to-Chinese and WMT15 German-to-English SiMT tasks demonstrate that our method can consistently improve translation quality at most latency regimes, with up to 2 BLEU scores improvement at low latency.
更多查看译文
关键词
simultaneous machine translation,machine translation,mitigating hallucination,prefix-to-prefix
AI 理解论文
溯源树
样例
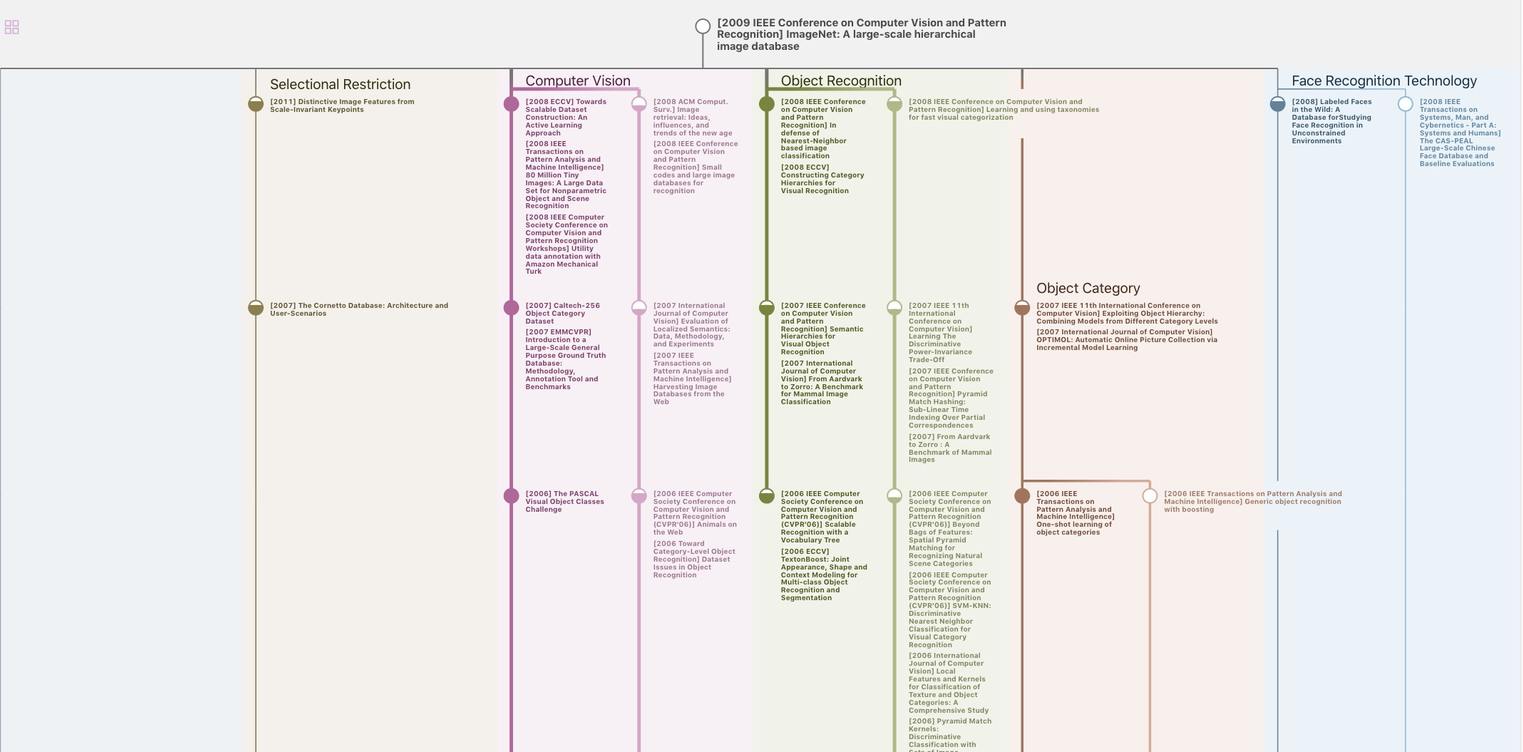
生成溯源树,研究论文发展脉络
Chat Paper
正在生成论文摘要