Measuring Adversarial Datasets
arXiv (Cornell University)(2023)
摘要
In the era of widespread public use of AI systems across various domains, ensuring adversarial robustness has become increasingly vital to maintain safety and prevent undesirable errors. Researchers have curated various adversarial datasets (through perturbations) for capturing model deficiencies that cannot be revealed in standard benchmark datasets. However, little is known about how these adversarial examples differ from the original data points, and there is still no methodology to measure the intended and unintended consequences of those adversarial transformations. In this research, we conducted a systematic survey of existing quantifiable metrics that describe text instances in NLP tasks, among dimensions of difficulty, diversity, and disagreement. We selected several current adversarial effect datasets and compared the distributions between the original and their adversarial counterparts. The results provide valuable insights into what makes these datasets more challenging from a metrics perspective and whether they align with underlying assumptions.
更多查看译文
关键词
adversarial datasets,measuring
AI 理解论文
溯源树
样例
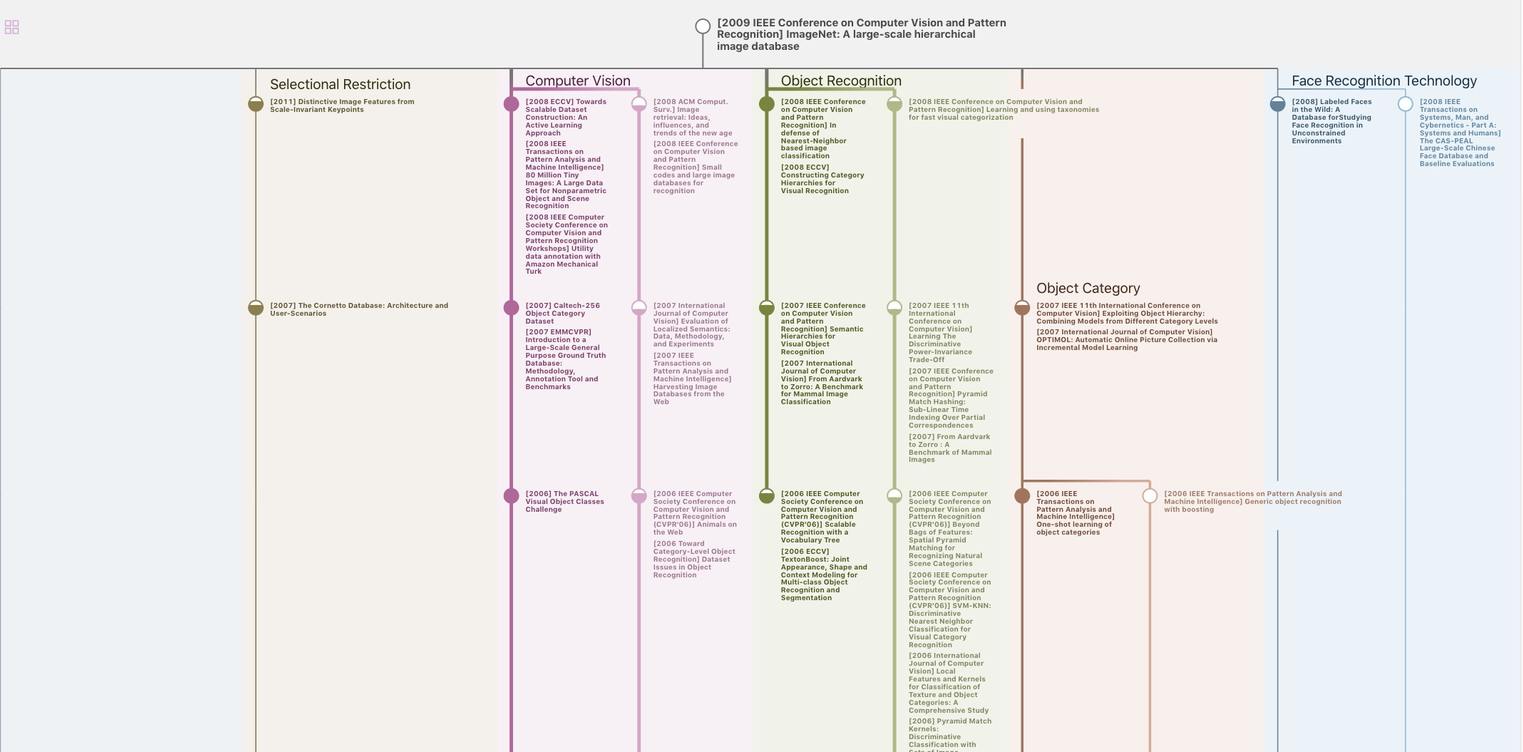
生成溯源树,研究论文发展脉络
Chat Paper
正在生成论文摘要