Solving seepage equation using physics-informed residual network without labeled data
COMPUTER METHODS IN APPLIED MECHANICS AND ENGINEERING(2024)
摘要
Physics-informed neural network (PINN) is an innovative universal function approximator which adds physical constraints to neural network to make the fitting results satisfy the physical laws better. In this paper, a physics-informed residual network (PIResNet) is proposed to solve the single-phase seepage equation without labeled data. The loss function is constructed by summarizing the residuals of the discretized seepage equation based on the finite volume method (FVM), and the boundary conditions are embedded in the PDE residuals in a "hard constraint'' way. The PIResNet is simple in network structure, fast in convergence and easy to optimize. Furthermore, the convergence of the residual structure used in this paper is proved and the theoretical analysis of time complexity illustrates the computational efficiency of PIResNet. The correctness of the proposed method is proved and the experiments display that the solution time of PIResNet is reduced by more than six times compared to physics-informed convolutional neural networks (PICNN).
更多查看译文
关键词
Seepage equation,Darcy flow,Physics information,Residual network,Reservoir simulation
AI 理解论文
溯源树
样例
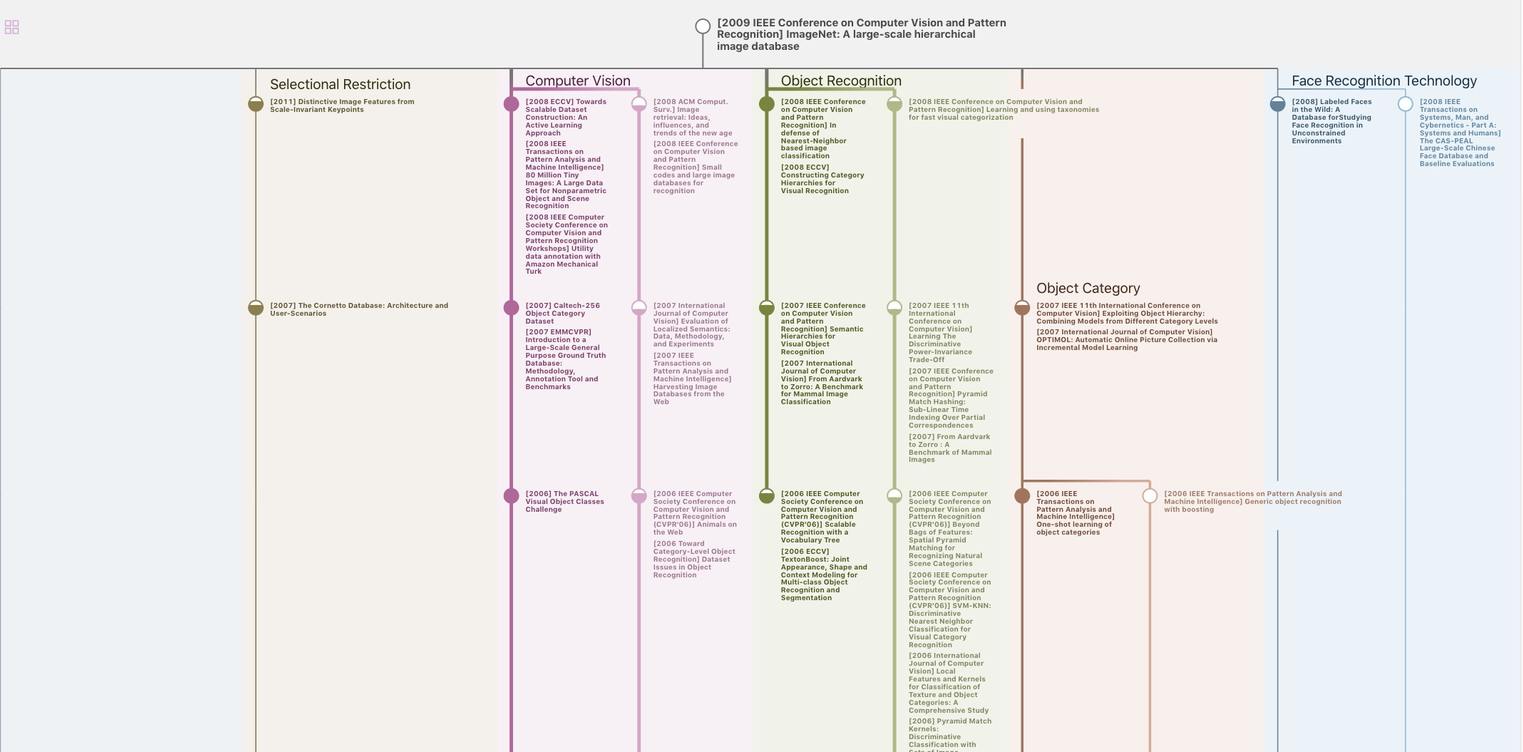
生成溯源树,研究论文发展脉络
Chat Paper
正在生成论文摘要