Double reverse diffusion for realistic garment reconstruction from images
ENGINEERING APPLICATIONS OF ARTIFICIAL INTELLIGENCE(2024)
摘要
Creating realistic digital 3D avatars has been getting more attention thanks to the introduction of new multimedia formats such as augmented and virtual reality. An important factor making avatars realistic is clothes. In this paper, we investigate a new method to reconstruct realistic garments from a set of images and body information. Early methods working on realistic images struggle to faithfully reconstruct the garment details. As deep learning is increasingly applied to geometric data which can conveniently represent garments, we devise a novel deep learning-based solution to the garment reconstruction problem. We offer a new perspective on the reconstruction problem and treat it as a reversion of the smoothing diffusion process. To achieve this goal, we propose to deform the smoothed human mesh into a clothed human via a Double Reverse Diffusion (DReD) process. For the first reverse diffusion, we introduce a novel operator called Graph Long Short-Term Memory (GraphLSTM) which recursively diffuses features to produce a deformed mesh by modeling the relationships between vertices. Then, the output mesh can be repeatedly upsampled and deformed by the above pipeline to obtain finer garment details, which can be seen as another reverse diffusion process. To obtain features for the reverse diffusion, we extract pixel-aligned features transferred from images and explore to incorporate the visibility of garments from the image viewpoints. Through detailed experiments on two public datasets, we demonstrate that DReD synthesizes more realistic wrinkled garments with lower errors and offers faster inference than previous methods.
更多查看译文
关键词
3D garment reconstruction,Deep learning,Mesh processing,Graph diffusion,Graph long short term memory,Double reverse diffusion
AI 理解论文
溯源树
样例
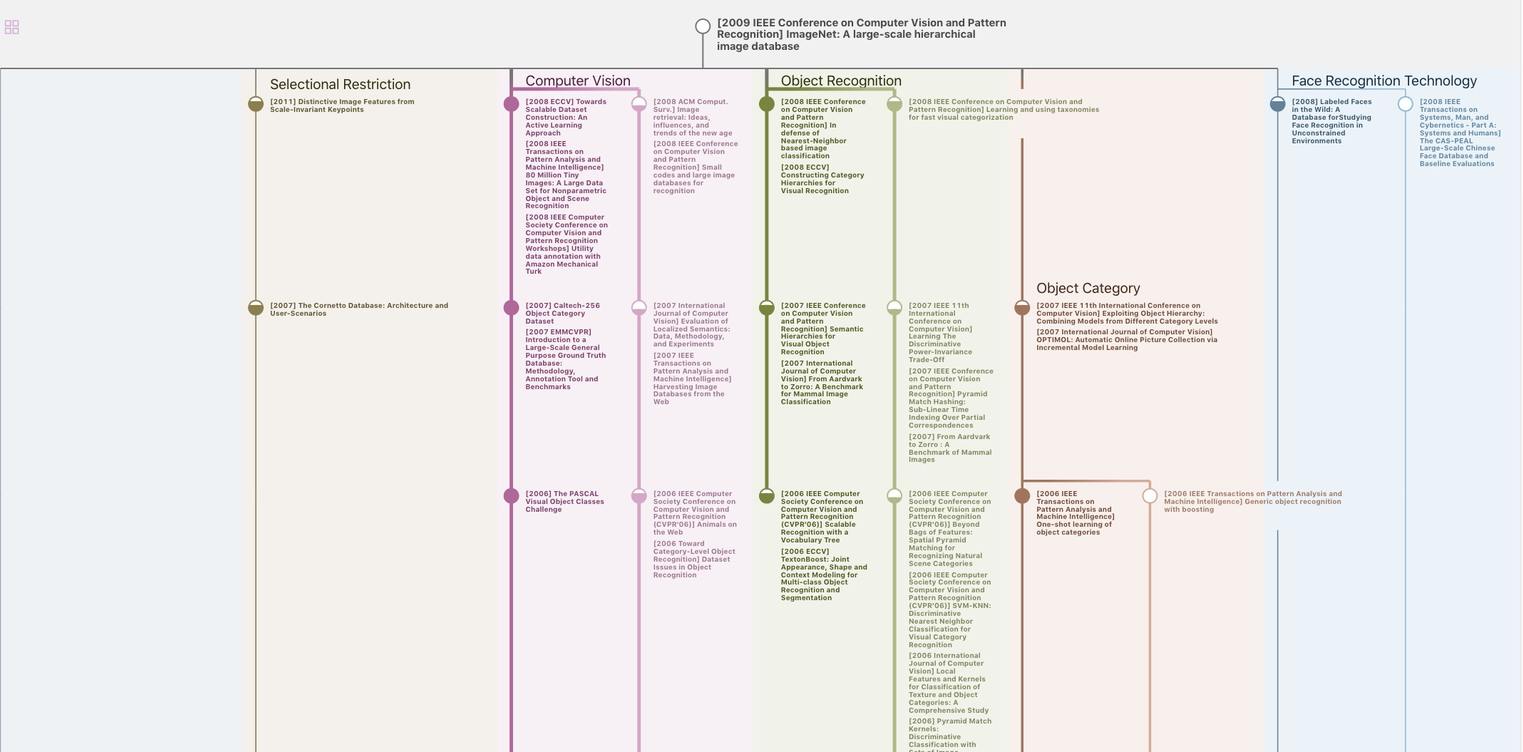
生成溯源树,研究论文发展脉络
Chat Paper
正在生成论文摘要