DC Neural Networks avoid overfitting in one-dimensional nonlinear regression
KNOWLEDGE-BASED SYSTEMS(2024)
摘要
In this paper, we analyze Difference of Convex Neural Networks in the context of one-dimensional nonlinear regression. Specifically, we show the surprising ability of the Difference of Convex Multilayer Perceptron (DC-MLP) to avoid overfitting in nonlinear regression. Otherwise said, DC-MLPs self-regularize (do not require additional regularization techniques). Thus, DC-MLPs could result very useful for practical purposes based on one-dimensional nonlinear regression. It turns out that shallow MLPs with a convex activation (ReLU, softplus, etc.) fall in the class of DC-MLPs. On the other hand, we call SQ-MLP the shallow MLP with a Squashing activation (logistic, hyperbolic tangent, etc.). In the numerical experiments, we show that DC-MLPs used for nonlinear regression avoid overfitting, in contrast with SQ-MLPs. We also compare DC-MLPs and SQ-MLPs from a theoretical point of view.
更多查看译文
关键词
DC neural network,Multilayer perceptron,Nonlinear regression,Overfitting
AI 理解论文
溯源树
样例
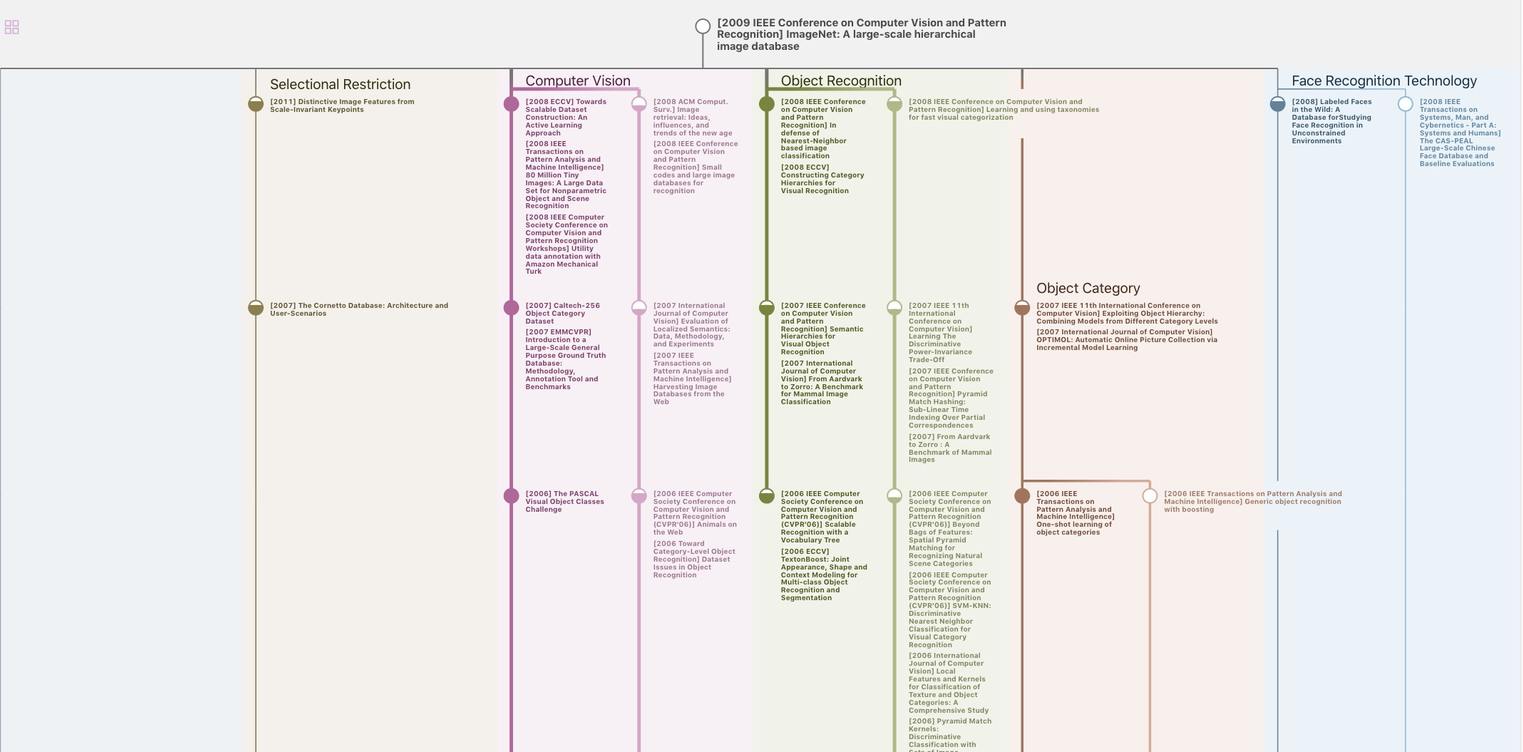
生成溯源树,研究论文发展脉络
Chat Paper
正在生成论文摘要