DA-DAN: A Dual Adversarial Domain Adaption Network for Unsupervised Non-overlapping Cross-domain Recommendation
ACM TRANSACTIONS ON INFORMATION SYSTEMS(2024)
摘要
Unsupervised Non-overlapping Cross-domain Recommendation (UNCR) is the task that recommends source domain items to the target domain users, which is more challenging as the users are non-overlapped, and its learning process is unsupervised. UNCR is still unsolved due to the following: (1) Previous studies need extra auxiliary information to learn transferable features when aligning two domains, which is unrealistic and hard to obtain due to privacy concerns. (2) Since the adoption of the shared network, existing works cannot well eliminate the domain-specific features in the common feature space, which may incorporate domain noise and harm the cross-domain recommendation. In this work, we propose a domain adaption-based method, namely DA-DAN, to address the above challenges. Specifically, to let DA-DAN be free of auxiliary information, we learn users' preferences by only exploring their sequential patterns, and propose an improved self-attention layer to model them. To well eliminate the domain-specific features from the common feature space, we resort to a dual generative adversarial network with a multi-target adversarial loss, where two generators and discriminators are leveraged to model each domain separately. Experimental results on three real-world datasets demonstrate the advantage of DA-DAN compared with the state-of-the-art recommendation baselines. Moreover, our source codes have been publicly released.(1)
更多查看译文
关键词
Cross-domain recommendation,sequential recommendation,unsupervised cross-domain recommendation
AI 理解论文
溯源树
样例
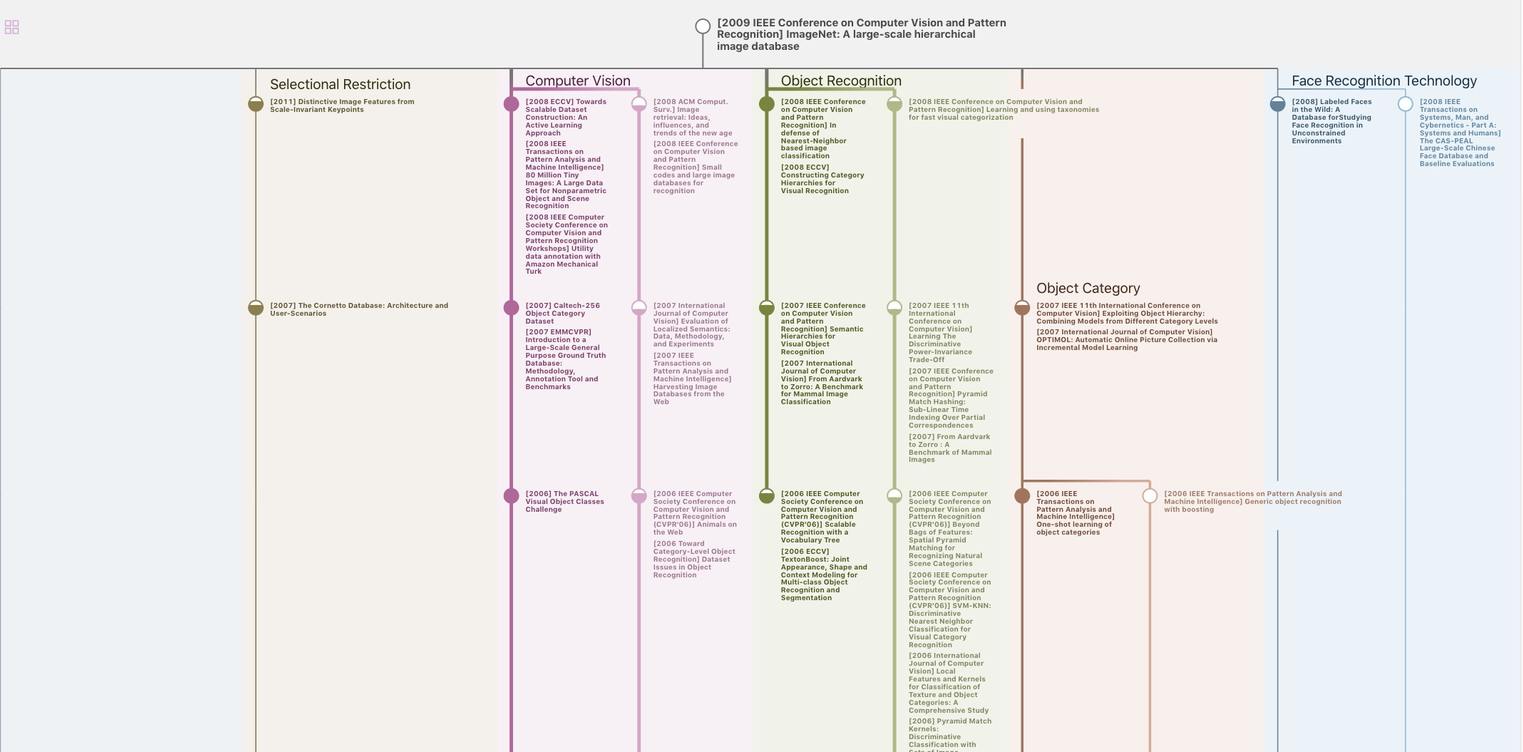
生成溯源树,研究论文发展脉络
Chat Paper
正在生成论文摘要