Systematic Review of risk score prediction models using maternal characteristics with and without biomarkers for the prediction of GDM
medRxiv (Cold Spring Harbor Laboratory)(2023)
摘要
Background GDM is associated with adverse maternal and fetal complications. By the time GDM is diagnosed, continuous exposure to the hyperglycaemic intrauterine environment can adversely affect the fetus. Hence, early pregnancy prediction of GDM is important.
Aim To systematically evaluate whether composite risk score prediction models can accurately predict GDM in early pregnancy.
Method Systematic review of observational studies involving pregnant women of <20 weeks of gestation was carried out. The search involved various databases, grey literature, and reference lists till August 2022. The primary outcome was the predictive performance of the models in terms of the AUC, for <14 weeks and 14-20 weeks of gestation.
Results Sixty-seven articles for <14 weeks and 22 for 14-20 weeks of gestation were included (initial search - 4542). The sample size ranged from 42 to 1,160,933. The studies were from Canada, USA, UK, Europe, Israel, Iran, China, Taiwan, South Korea, South Africa, Australia, Singapore, and Thailand. For <14 weeks, the AUC ranges were 0.59-0.88 and 0.53-0.95, respectively for models that used only maternal characteristics and for those that included biomarkers. For 14-20 weeks these AUCs were 0.68-0.71 and 0.65-0.92. Age, ethnicity, BMI, family history of diabetes, and prior GDM were the 5 most commonly used risk factors. The addition of systolic BP improved performance in some models. Triglycerides, PAPP-A, and lipocalin- 2, combined with maternal characteristics, have the highest predictive performance. AUC varied according to the population studied. Pooled analyses were not done due to high heterogeneity.
Conclusion Accurate GDM risk prediction may be possible if common risk factors are combined with biomarkers. However, more research is needed in populations of high GDM risk. Artificial Intelligence-based risk prediction models that incorporate fetal biometry data may improve accuracy.
### Competing Interest Statement
The authors have declared no competing interest.
### Funding Statement
PS and YW are partly funded by Medical Research Council, UK (MR/R020981/1); DP and SS are funded by Warwick-Novo Nordisk international Doctoral Training Program.
### Author Declarations
I confirm all relevant ethical guidelines have been followed, and any necessary IRB and/or ethics committee approvals have been obtained.
Yes
I confirm that all necessary patient/participant consent has been obtained and the appropriate institutional forms have been archived, and that any patient/participant/sample identifiers included were not known to anyone (e.g., hospital staff, patients or participants themselves) outside the research group so cannot be used to identify individuals.
Yes
I understand that all clinical trials and any other prospective interventional studies must be registered with an ICMJE-approved registry, such as ClinicalTrials.gov. I confirm that any such study reported in the manuscript has been registered and the trial registration ID is provided (note: if posting a prospective study registered retrospectively, please provide a statement in the trial ID field explaining why the study was not registered in advance).
Yes
I have followed all appropriate research reporting guidelines, such as any relevant EQUATOR Network research reporting checklist(s) and other pertinent material, if applicable.
Yes
All data produced in the present study are available upon reasonable request to the authors.
更多查看译文
关键词
risk score prediction models,maternal characteristics,risk score
AI 理解论文
溯源树
样例
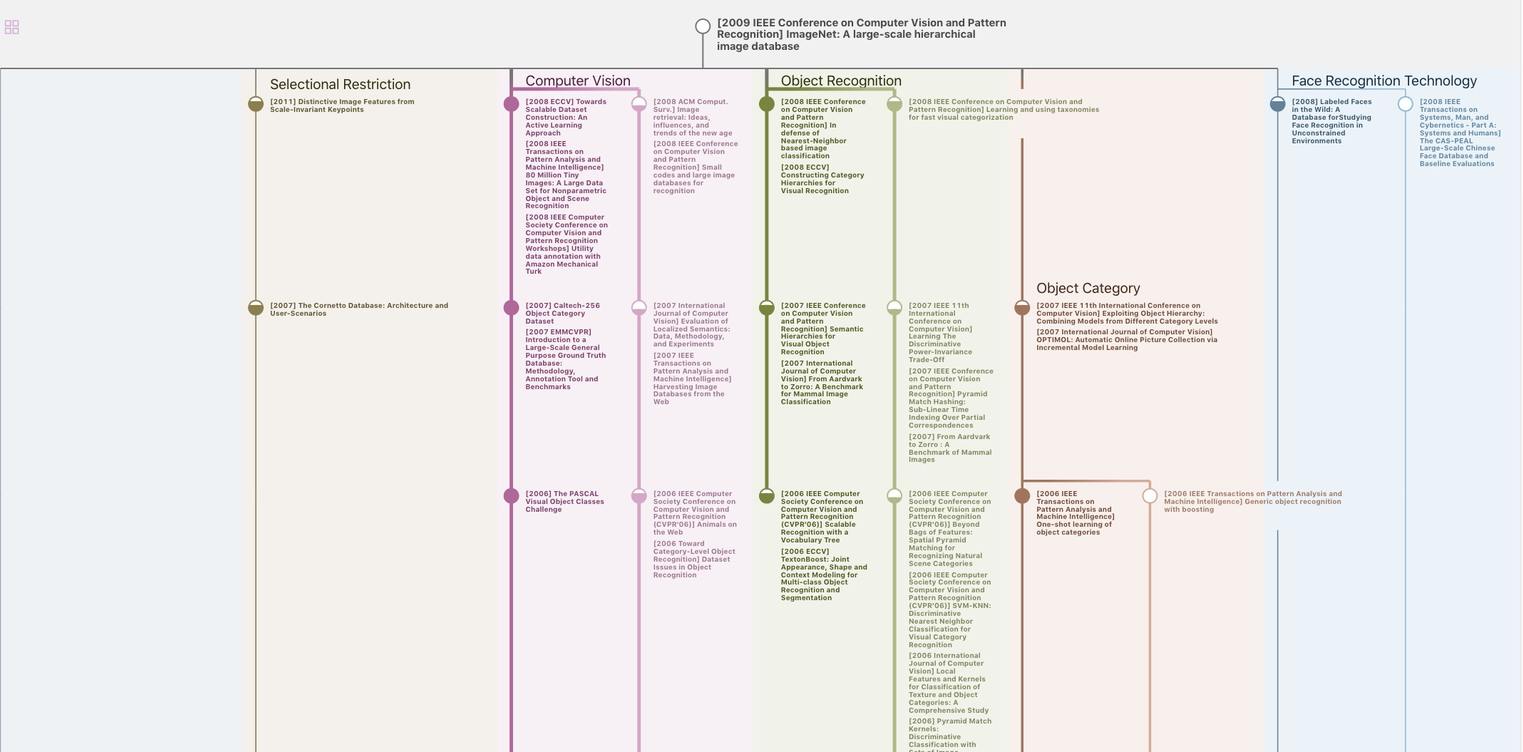
生成溯源树,研究论文发展脉络
Chat Paper
正在生成论文摘要