Analytical framework for the development of water quality virtual sensors in integrated aquaculture systems based on various aquaponics techniques.
ETFA(2023)
摘要
The present research targets to offer preliminary perspectives related to the use of machine learning tools for creating a complex analytical framework which targets to provide decision support for integrated aquaculture systems (IMS) based on aquaponics techniques with different peculiarities. Therefore, virtual sensors for the determination of ammonium chloride (NH
4
Cl) and nitrate (NO
3
), considered the most important chemical compounds during the biological filter activation and aquaponics production cycle, respectively, were developed by using various water quality predictors, in various technical and technological scenarios. Different machine learning-based techniques such as multiple-linear regressions (MLR), XGBoost and Random Forrest (RF) algorithms were used for establishing a high-accuracy prediction framework. The results emphasize that both MLR and XGBoost-based models recorded high prediction accuracy, compared to RF algorithms. The most important predictors differ between the experimental variants, emphasizing the necessity of establishing a multitude of complex prediction frameworks, considering all the technical aspects of IMS.
更多查看译文
关键词
machine learning,XGBoost,random forest,multiple-linear regressions
AI 理解论文
溯源树
样例
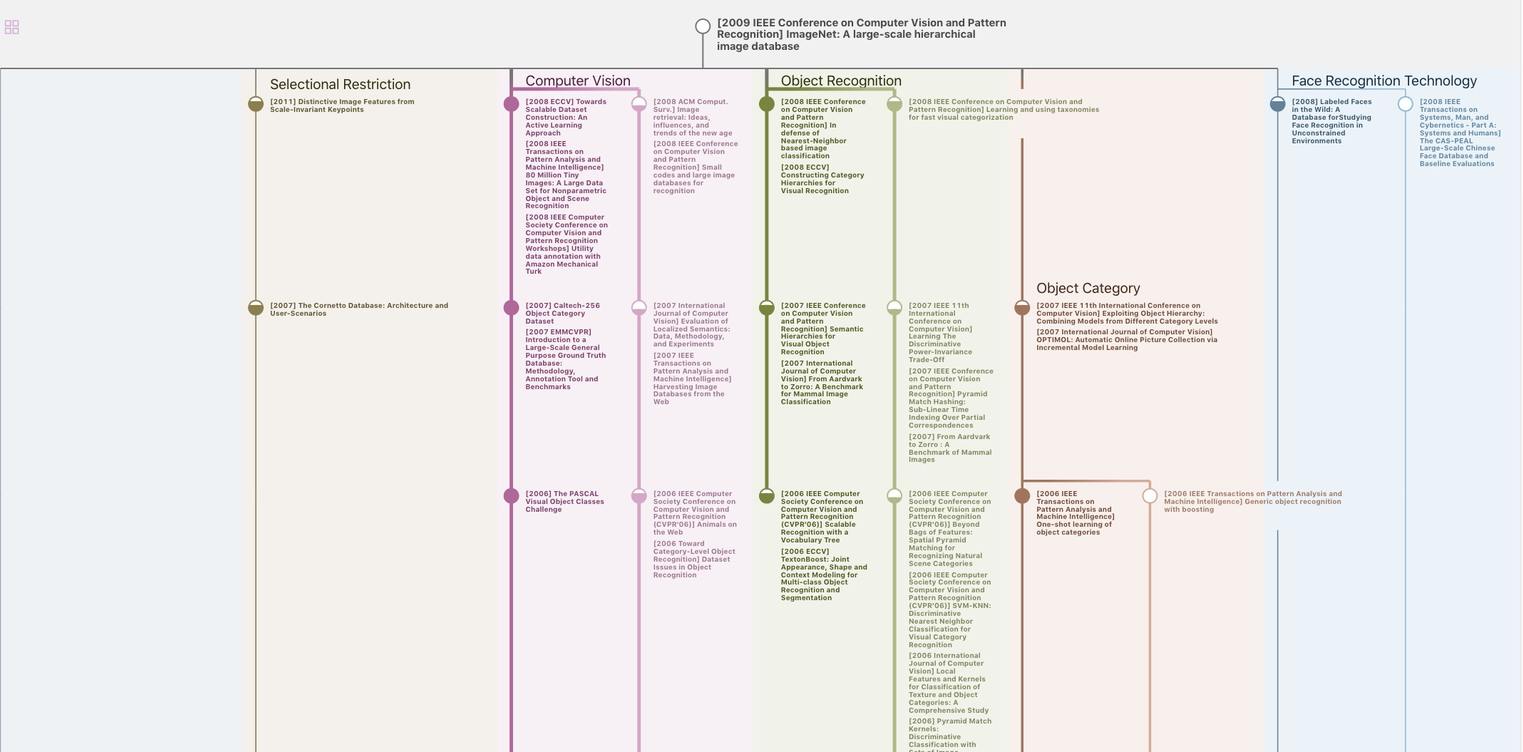
生成溯源树,研究论文发展脉络
Chat Paper
正在生成论文摘要