Remaining Useful Life Estimation for Railway Gearbox Bearings Using Machine Learning
RELIABILITY, SAFETY, AND SECURITY OF RAILWAY SYSTEMS, RSSRAIL 2023(2023)
摘要
Gearbox bearing maintenance is one of the major overhaul cost items for railway electric propulsion systems. They are continuously exposed to challenging working conditions, which compromise their performance and reliability. Various maintenance strategies have been introduced over time to improve the operational efficiency of such components, while lowering the cost of their maintenance. One of these is predictive maintenance, which makes use of previous historical data to estimate a component's remaining useful life (RUL). This paper introduces a machine learning-based method for calculating the RUL of railway gearbox bearings. The method uses unlabeled mechanical vibration signals from gearbox bearings to detect patterns of increased bearing wear and predict the component's residual life span. We combined a data smoothing method, a change point algorithm to set thresholds, and regression models for prediction. The proposed method has been validated using real-world gearbox data provided by our industrial partner, Alstom Transport AB in Sweden. The results are promising, particularly with respect to the predicted failure time. Our model predicted the failure to occur on day 330, while the gearbox bearing's actual lifespan was 337 days. The deviation of just 7 days is a significant result, since an earlier RUL prediction value is usually preferable to avoid unexpected failure during operations. Additionally, we plan to further enhance the prediction model by including more data representing failing bearing patterns.
更多查看译文
关键词
Railway,Gearbox bearing,Predictive maintenance,Remaining useful life,Machine learning
AI 理解论文
溯源树
样例
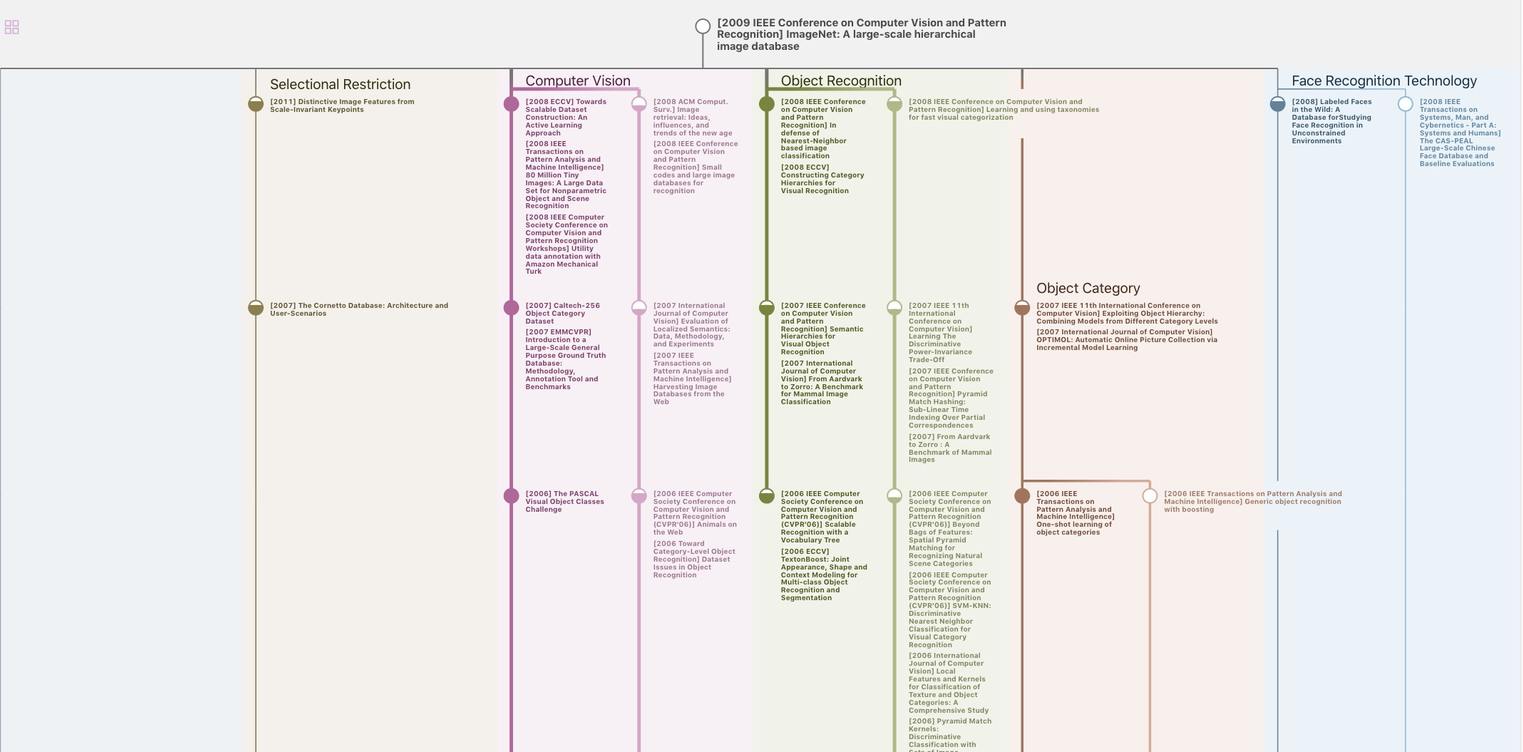
生成溯源树,研究论文发展脉络
Chat Paper
正在生成论文摘要