Evaluating Explanations of an Alzheimer's Disease 18F-FDG Brain PET Black-Box Classifier.
xAI (1)(2023)
摘要
eXplainable Artificial Intelligence (XAI) has been increasingly applied to interpret Deep Neural Networks (DNN) in medical imaging applications, but a general consensus about the best interpretation strategy is missing. This is also due to the absence of a validated framework to assess the quality of the explanations/interpretations produced by different XAI methods. This work aims to quantify the ability of interpretation techniques of producing good explanations and non-misleading representations of what a black-box model has learned. We selected a DNN which classifies 18F-FDG PET images according to the cognitive decline in Alzheimer’s disease, and we applied two different interpretability methods commonly employed in bioimaging: attribution maps (Backpropagation, GradCAM++, Layerwise Relevance Propagation), and latent space interpretation (t-SNE, UMAP, TriMAP, PaCMAP). We evaluated the interpretations using different literature frameworks: evaluation of attribution maps with imaging biomarkers and region perturbation, and preservation of data local and global structure of the latent space. Results suggested that we are not able to observe a clear relationship between the PET signal and attribution maps, highlighting the importance of not assuming that XAI explanations should reflect the human’s reasoning. Layerwise Relevance Propagation best explains the classifier’s decisions according to the region-perturbation evaluation, confirming literature results. Finally, the UMAP and the TriMAP embedding respectively reported the best result for the preservation of the local and the global data structure, which is, to the best of our knowledge, the first systematic assessment in the medical imaging domain, and in line with theoretical background of the methods employed.
更多查看译文
关键词
alzheimers,brain,pet,f-fdg,black-box
AI 理解论文
溯源树
样例
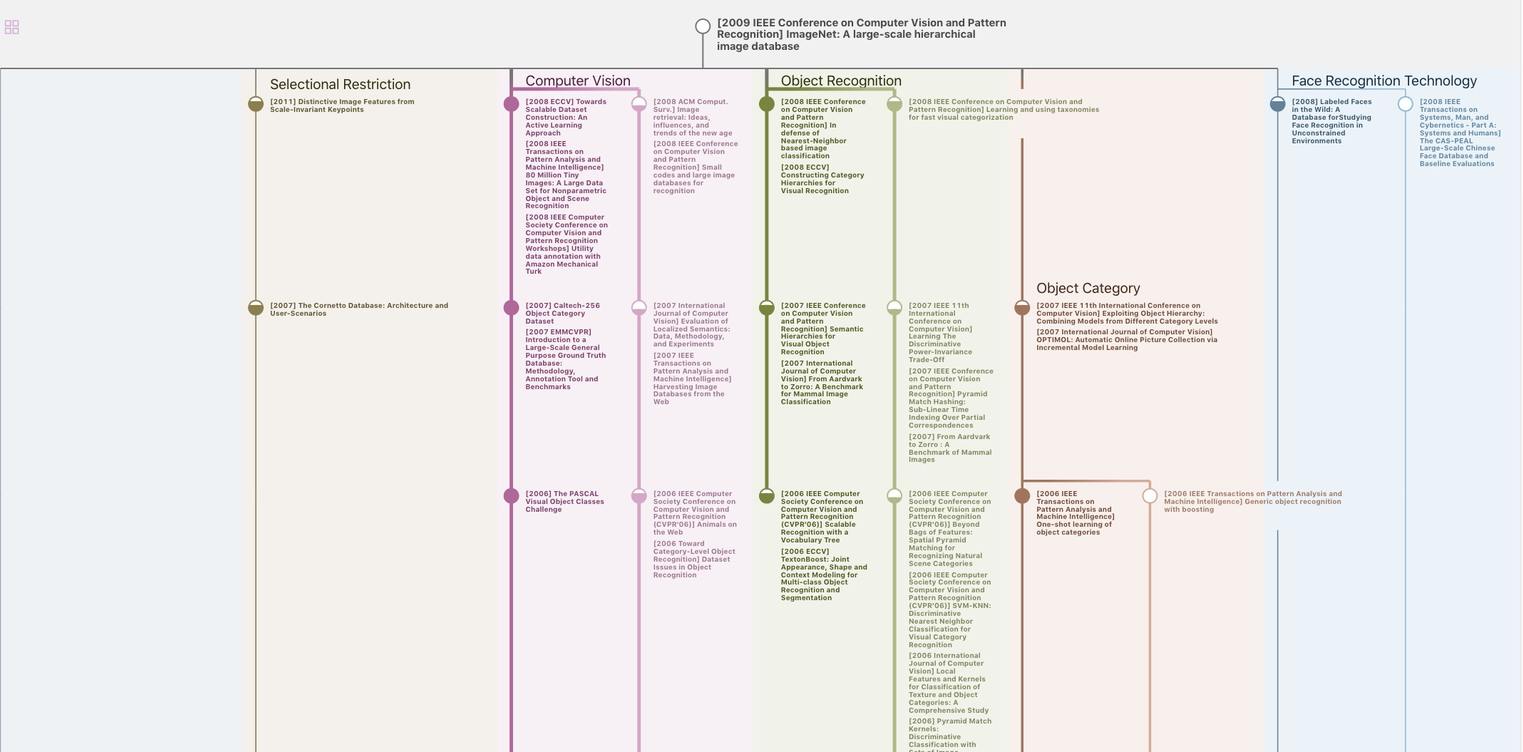
生成溯源树,研究论文发展脉络
Chat Paper
正在生成论文摘要