Lorenz Zonoids for Trustworthy AI.
xAI (1)(2023)
摘要
Machine learning models are boosting Artificial Intelligence (AI) applications in many domains, such as finance, health care and automotive. This is mainly due to their advantage, in terms of predictive accuracy, with respect to “classic” statistical learning models. However, although complex machine learning models may reach high predictive performance, their predictions are not explainable and have an intrinsic black-box nature. Accuracy and explainability are not the only desirable characteristics of a machine learning model. The recently proposed European regulation on Artificial Intelligence, the AI Act, attempts to regulate the use of AI by means of a set of requirements of trustworthiness for high risk applications, to be embedded in a risk management model. We propose to map the requirements established for high-risk applications in the AI Act in four main variables: Sustainability, Accuracy, Fairness and Explainability, which need a set of metrics that can establish not only whether but also how much the requirements are satisfied over time. To the best of our knowledge, there exists no such set of metrics, yet. In this paper, we aim to fill this gap, and propose a set of four integrated metrics, aimed at measuring Sustainability, Accuracy, Fairness and Explainability (S.A.F.E. in brief), which have the advantage, with respect to the available metrics, of being all based on one unifying statistical tool: the Lorenz curve. The Lorenz curve is a well known robust statistical tool, which has been employed, along with the related Gini index to measure income and wealth inequalities. It thus appears as a natural methodology on which to build an integrated set of trustworthy AI measurement metrics.
更多查看译文
关键词
lorenz zonoids,ai
AI 理解论文
溯源树
样例
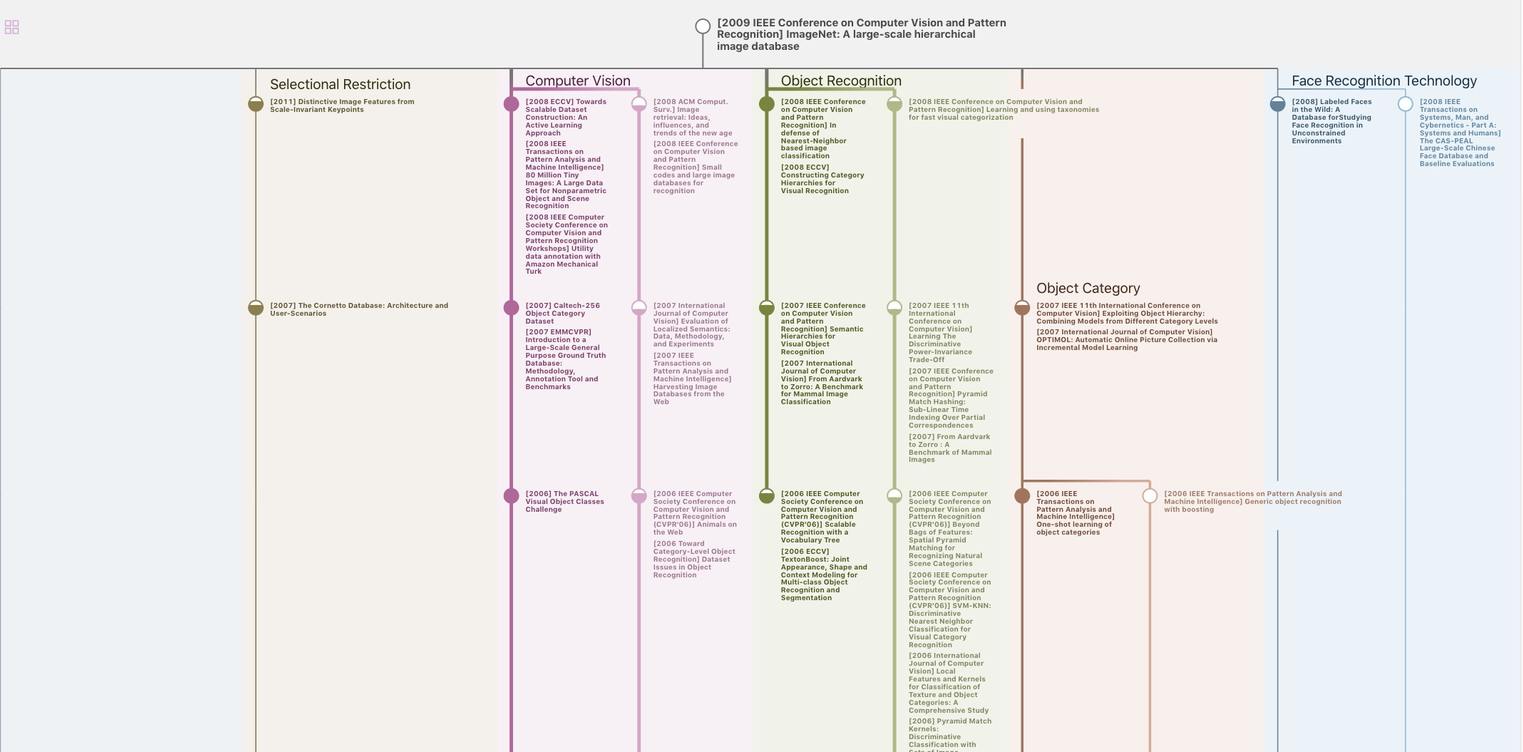
生成溯源树,研究论文发展脉络
Chat Paper
正在生成论文摘要