OnMKD: An Online Mutual Knowledge Distillation Framework for Passage Retrieval.
NLPCC (2)(2023)
摘要
Dense passage retriever recalls a set of relevant passages from a large corpus according to a natural language question. The dual-encoder architecture is prevalent in dense passage retrievers, which is based on large-scale pre-trained language models (PLMs). However, existing PLMs usually have thick structures and bulky parameters, resulting in large memory and time consumption. To overcome the limitation of PLMs, in this paper we apply online distillation to passage retrieval and propose an Online Mutual Knowledge Distillation framework (OnMKD). Specifically, we obtain a lightweight retriever by simultaneously updating two peer networks with the same dual-encoder structure and different initial parameters, named Online Mutual Knowledge Refinement. To further interact with the latent knowledge of intermediate layers, we utilize a novel cross-wise contrastive loss to alternate the representation of questions and passages. Experimental results indicate that our framework outperforms other small baselines with the same number of layers on multiple QA benchmarks. Compared to the heavy PLMs, OnMKD significantly accelerates the inference process and reduces storage requirements with only a slight sacrifice in performance.
更多查看译文
关键词
knowledge
AI 理解论文
溯源树
样例
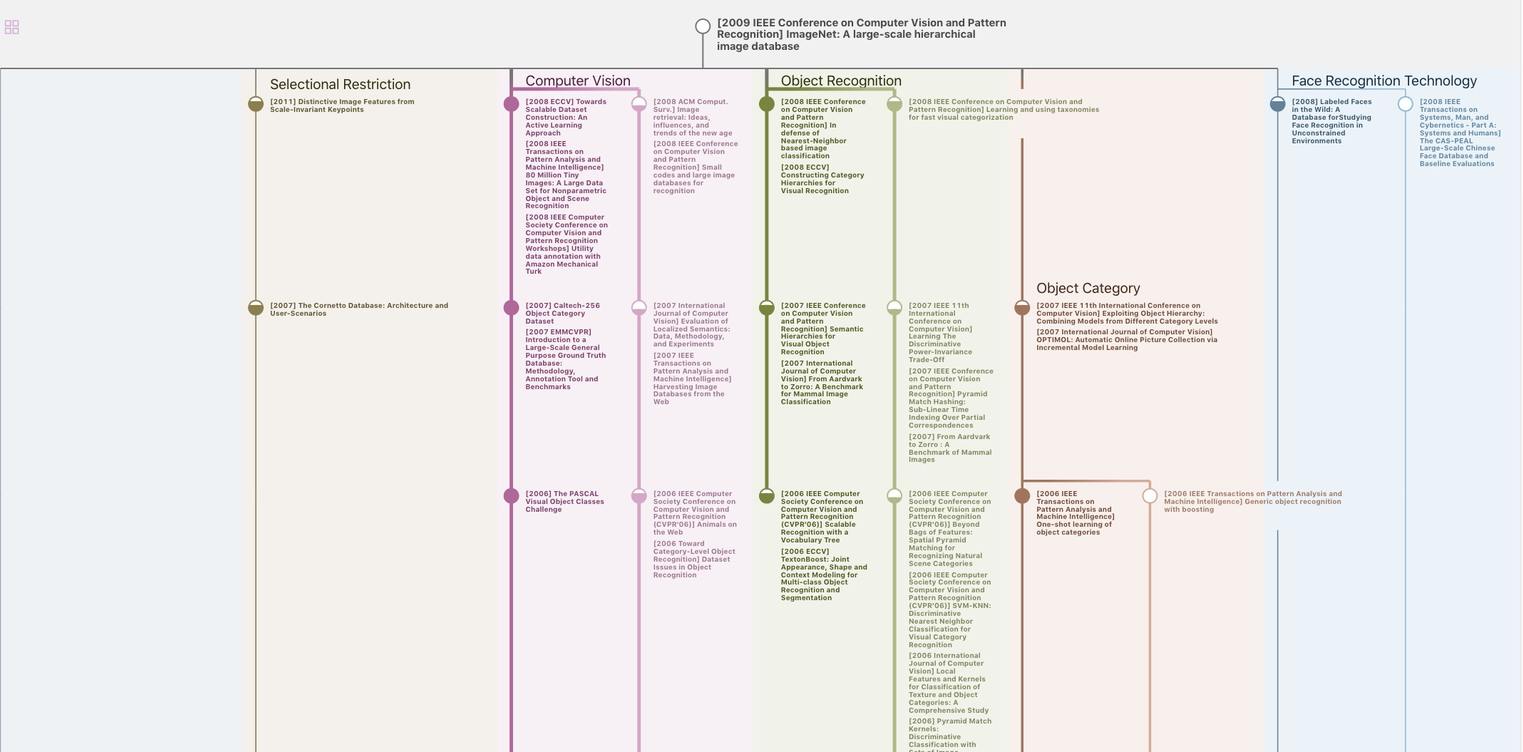
生成溯源树,研究论文发展脉络
Chat Paper
正在生成论文摘要