Incorporating Social-Aware User Preference for Video Recommendation.
WISE(2023)
摘要
Modeling user interest accurately is crucial to recommendation systems. Existing works capture user interest from historical behaviors. Due to the sparsity and noise in user behavior data, behavior based models learn incomplete and sometimes inaccurate preference patterns and easily suffer from the cold user problem. In this work, we propose a social graph enhanced framework for behavior based models, namely Social4Rec. The social graph, involving multiple relation types, is extracted to find users with similar interests. It is challenging due to the trivial and sparse relations in social graph. To address the sparse relations issue, we first propose a Cluster-Calibrate-Merge network (CCM) to discover interest groups satisfying three properties: intrinsic self-organizing patterns through cluster layer, robustness to sparse relations through knowledge distillation of calibrator layer. We then use the averaged user interest representation within each group from CCM to complete each user behavior embedding and obtain relation specific interest aware embedding. To alleviate the trivial relation problem, relation specific interest aware embedding are aggregated among relation types through attention mechanism to obtain the interest aware social embedding for each user. It is combined with user behavior embedding to derive the matching score between the user and item. Both offline and online experiments on our video platform, which is one of the biggest video recommendation platforms with nearly one billion users over the world, demonstrate the superiority of our method, especially for cold users. The codes are available at https://github.com/xuanjixiao/onerec .
更多查看译文
关键词
video recommendation,preference,social-aware
AI 理解论文
溯源树
样例
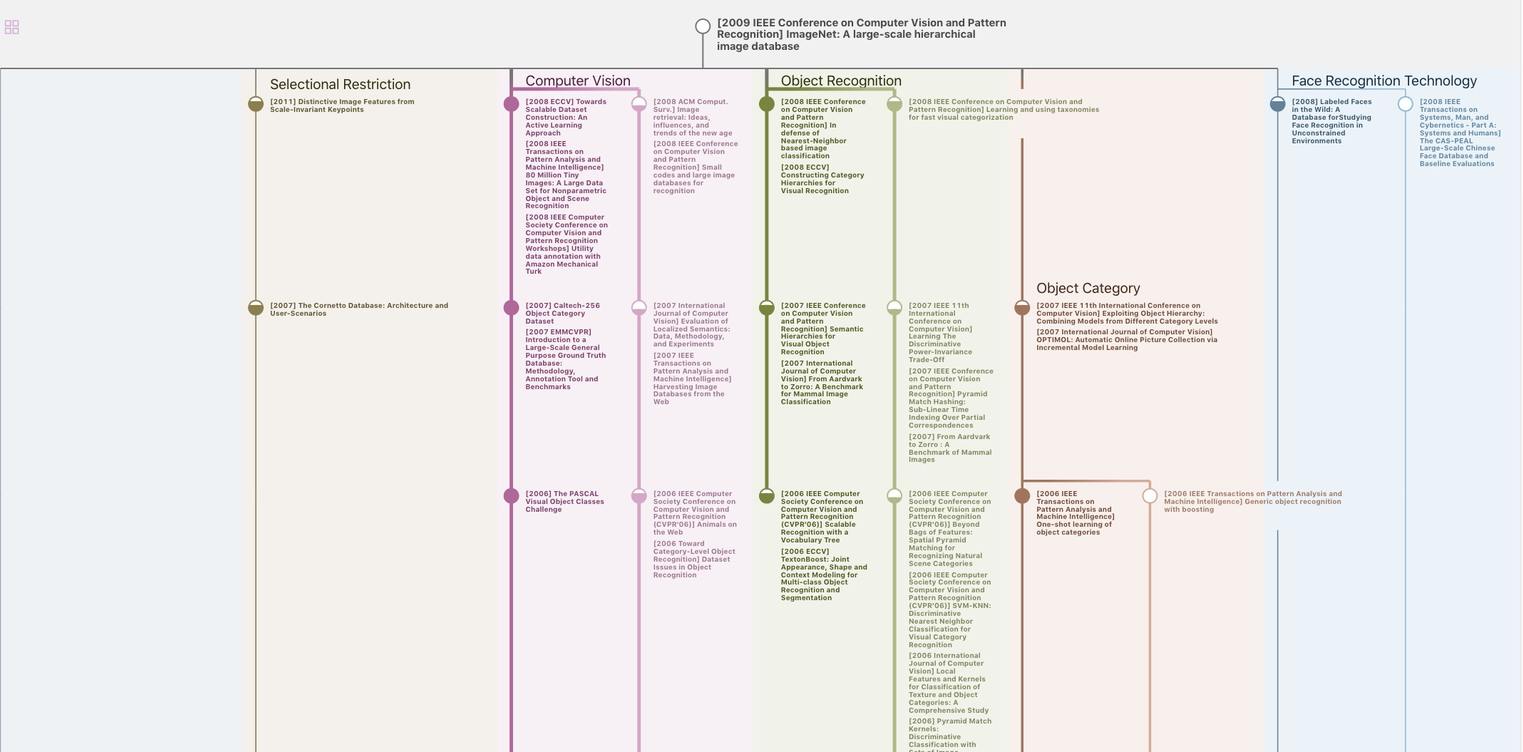
生成溯源树,研究论文发展脉络
Chat Paper
正在生成论文摘要