An Improved Method for Constructing Domain-Agnostic Knowledge Graphs
DATABASE SYSTEMS FOR ADVANCED APPLICATIONS. DASFAA 2023 INTERNATIONAL WORKSHOPS, BDMS 2023, BDQM 2023, GDMA 2023, BUNDLERS 2023(2023)
摘要
Texts contain a vast amount of useful information. Converting unstructured text into structured data enables machines to better understand and mine useful information within texts. Knowledge graphs provide a natural method for data representation and can be applied in downstream tasks. However, existing knowledge graph construction (KGC) methods are mostly domain-specific, which heavily rely on specific external knowledge bases. In this paper, we focus on the domain-agnostic knowledge graph construction task to decouple the reliance on external knowledge. For this purpose, we propose a new method for constructing knowledge graphs from texts, which improves the accuracy of the baseline method Seq2KG. Specifically, we employ a state-of-the-art coreference resolution module in the pre-pocessing stage to provide more priori information for subsequent network learning. Moreover, we introduce a self-attention layer to dynamically model the weights of tokens, thereby enhancing the token representation in the sequence. Our experiments on three public datasets demonstrate that our new model outperforms Seq2KG in both triple extraction and entity typing accuracy.
更多查看译文
关键词
Knowledge Graph,Self-Attention,Coreference Resolution
AI 理解论文
溯源树
样例
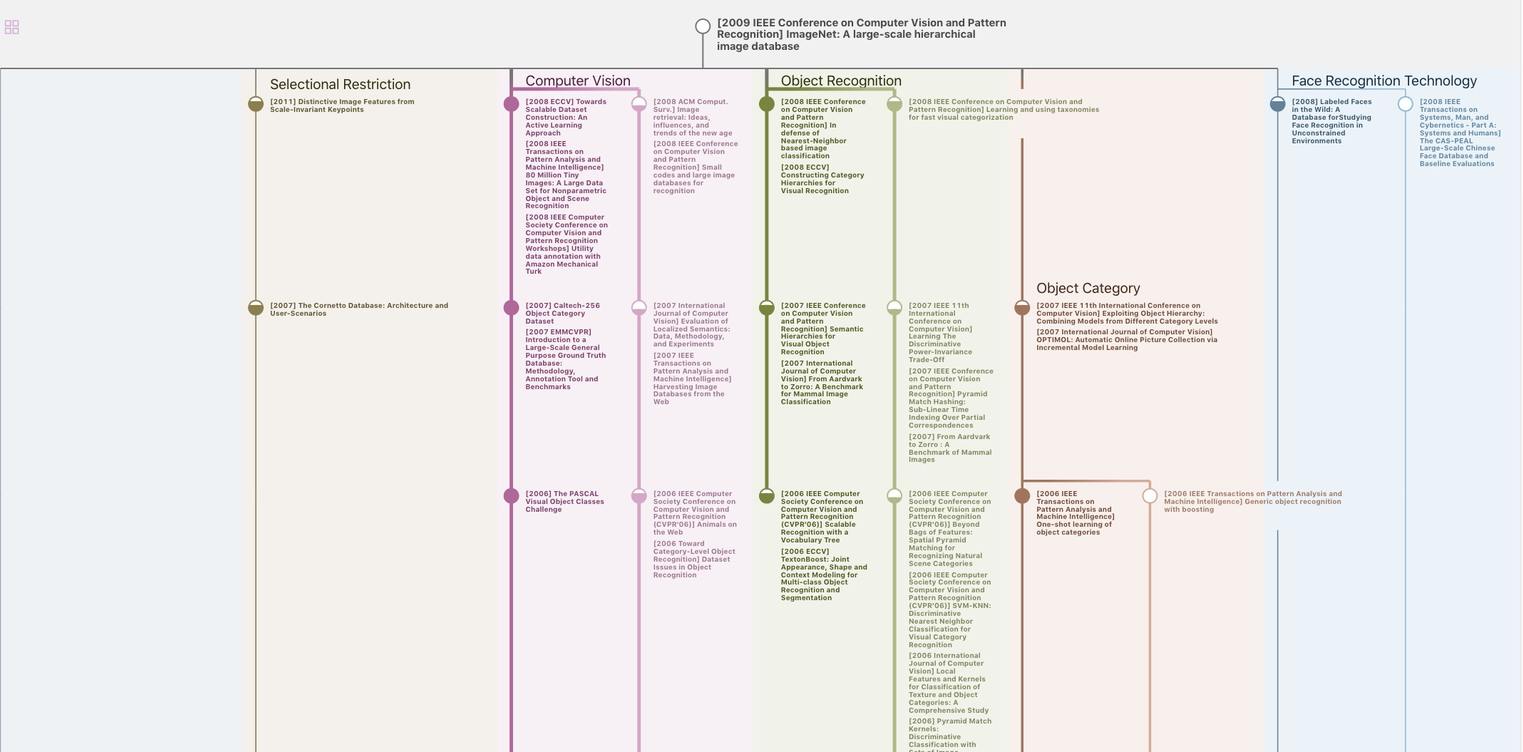
生成溯源树,研究论文发展脉络
Chat Paper
正在生成论文摘要