Evaluation Framework for Poisoning Attacks on Knowledge Graph Embeddings.
NLPCC (1)(2023)
摘要
In the area of knowledge graph embedding data poisoning, attackers are beginning to consider the importance of poisoning sample exposure risk while increasing the toxicity of the poisoning sample. On the other hand, we have found that some researchers incorrectly assess the effectiveness of poisoning attacks without considering the impact of the data-adding operation on model performance. Also, there is currently no definition of the Stealthiness of poisoning attacks. To address this issue, we provide an objective and unified framework for evaluating complex and diverse poisoning strategies. We design a controlled experiment on poisoning attacks to obtain objectively correct poisoning effects, and propose toxicity Dt to evaluate the poisoning performance of poisoning attacks and stealthiness Ds to evaluate the exposure risk of poisoning attacks. In designing the metrics, we fully considered the performance of the control model and the generalizability of the attacked model so that the data poisoning effect can be objectively and correctly evaluated. We compared 12 recently proposed KG attack methods on two different benchmark datasets to verify the objectivity and correctness of our evaluation criteria and to analyze the impact of the generalizability of the attacked model.
更多查看译文
关键词
knowledge graph embeddings,poisoning attacks
AI 理解论文
溯源树
样例
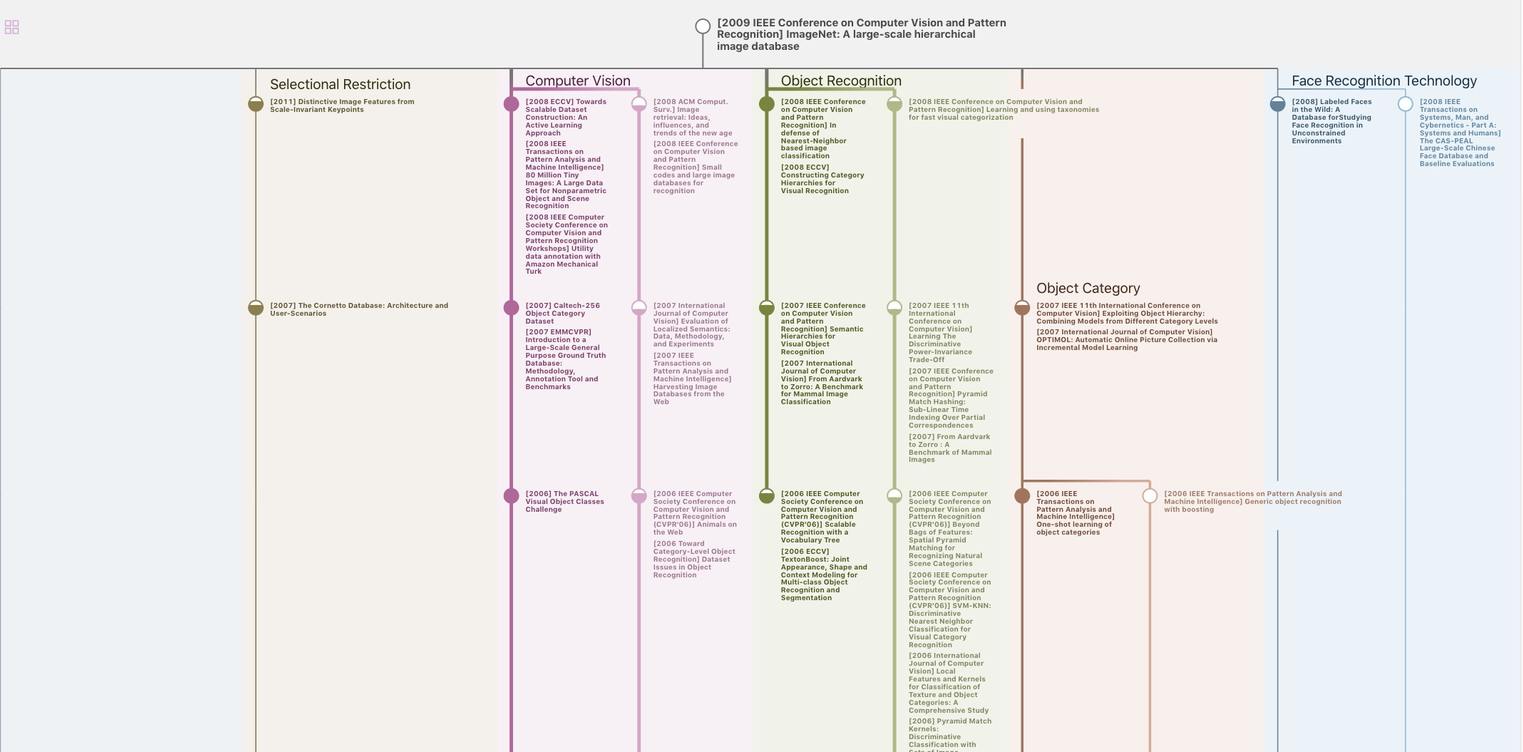
生成溯源树,研究论文发展脉络
Chat Paper
正在生成论文摘要