GAN-IE: Generative Adversarial Network for Information Extraction with Limited Annotated Data.
WISE(2023)
摘要
Extracting valuable information from a large corpus of unstructured data poses a formidable challenge in many applications. Transformer-based architectures e.g. BERT, employing transfer learning techniques, have exhibited promising results across diverse NLP tasks. Nonetheless, the practical implementation of these models presents a significant hurdle given the substantial demand for annotated data during the training phase. In this paper, we present GAN-IE, a novel GAN-based model architecture, designed specifically for information extraction from unstructured textual data while accounting for limited annotated resources. In a generative adversarial setting, GAN-IE leverages BERT’s rich semantic and contextual knowledge obtained from unlabelled data while fine-tuning. Experimental results show that GAN-IE achieves a level of accuracy that surpasses the current state-of-the-art models when trained using a fraction of labelled data ( $$\sim $$ 100–200 annotated samples).
更多查看译文
关键词
generative adversarial network,information extraction
AI 理解论文
溯源树
样例
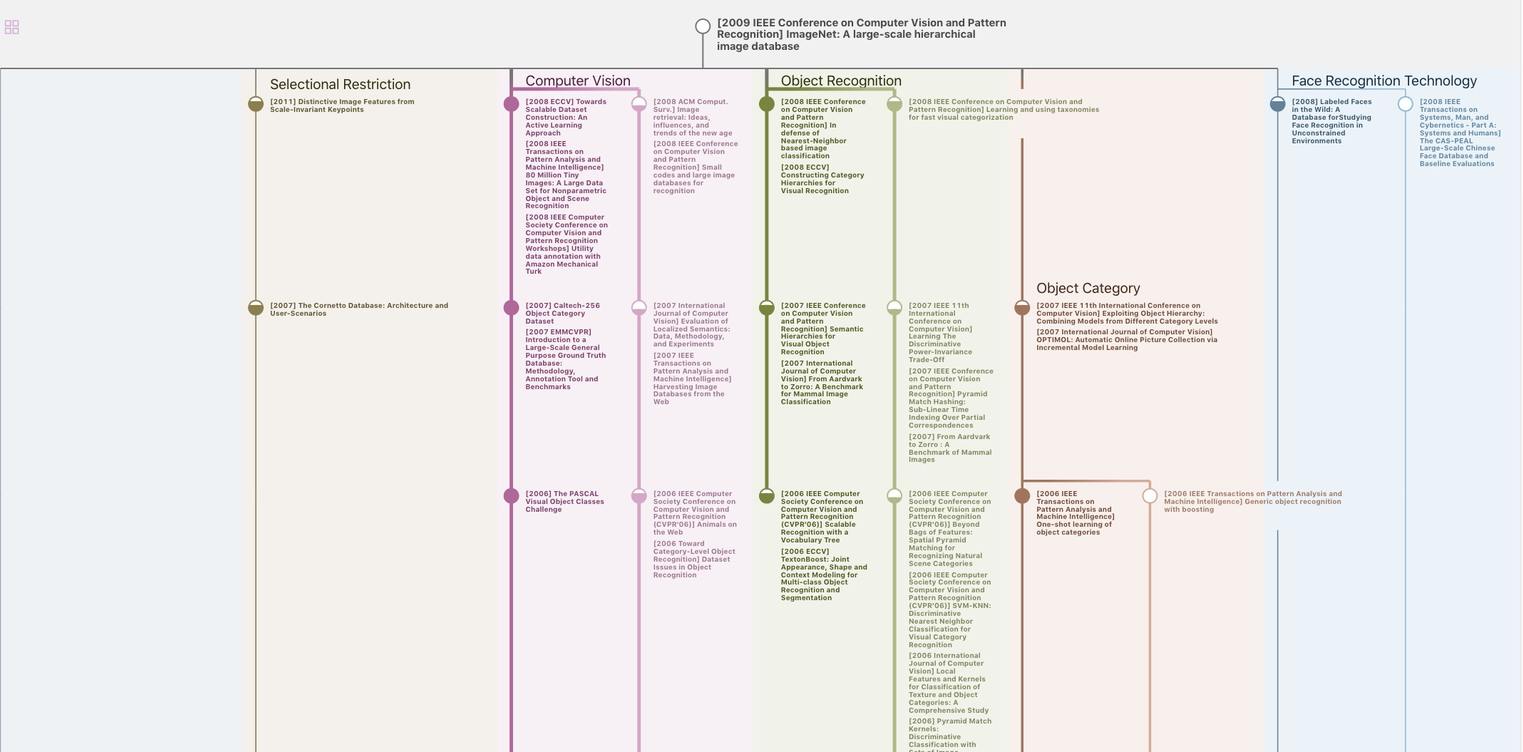
生成溯源树,研究论文发展脉络
Chat Paper
正在生成论文摘要