A Privacy-Preserving Evolutionary Computation Framework for Feature Selection.
WISE(2023)
摘要
Feature selection is a crucial process in data science that involves selecting the most effective subset of features. Evolutionary computation (EC) is one of the most commonly-used feature selection techniques and has demonstrated good performance, which can help find the suitable feature subset based on training data and fitness information. However, in real-world scenarios, the exact fitness information and privacy-protected data cannot be directly accessed due to privacy and security issues, which leads to a great optimization challenge. To solve such privacy-preserving feature selection problems efficiently, this paper proposes a novel EC-based feature selection framework that balances data privacy and optimization efficiency, together with three contributions. First, based on the rank-based cryptographic function that returns the rank of solutions rather than the exact fitness information, this paper proposes a new fitness function to guide the EC algorithm to approach the global optimum without knowing the exact fitness information and the dataset, thereby preserving data privacy. Second, by integrating the proposed method and EC algorithms, this paper develops a new differential evolution and particle swarm optimization algorithms for efficient feature selection. Finally, experiments are conducted on public datasets, which demonstrate that the proposed method can maintain feature selection efficiency while preserving data privacy.
更多查看译文
关键词
feature selection,privacy-preserving
AI 理解论文
溯源树
样例
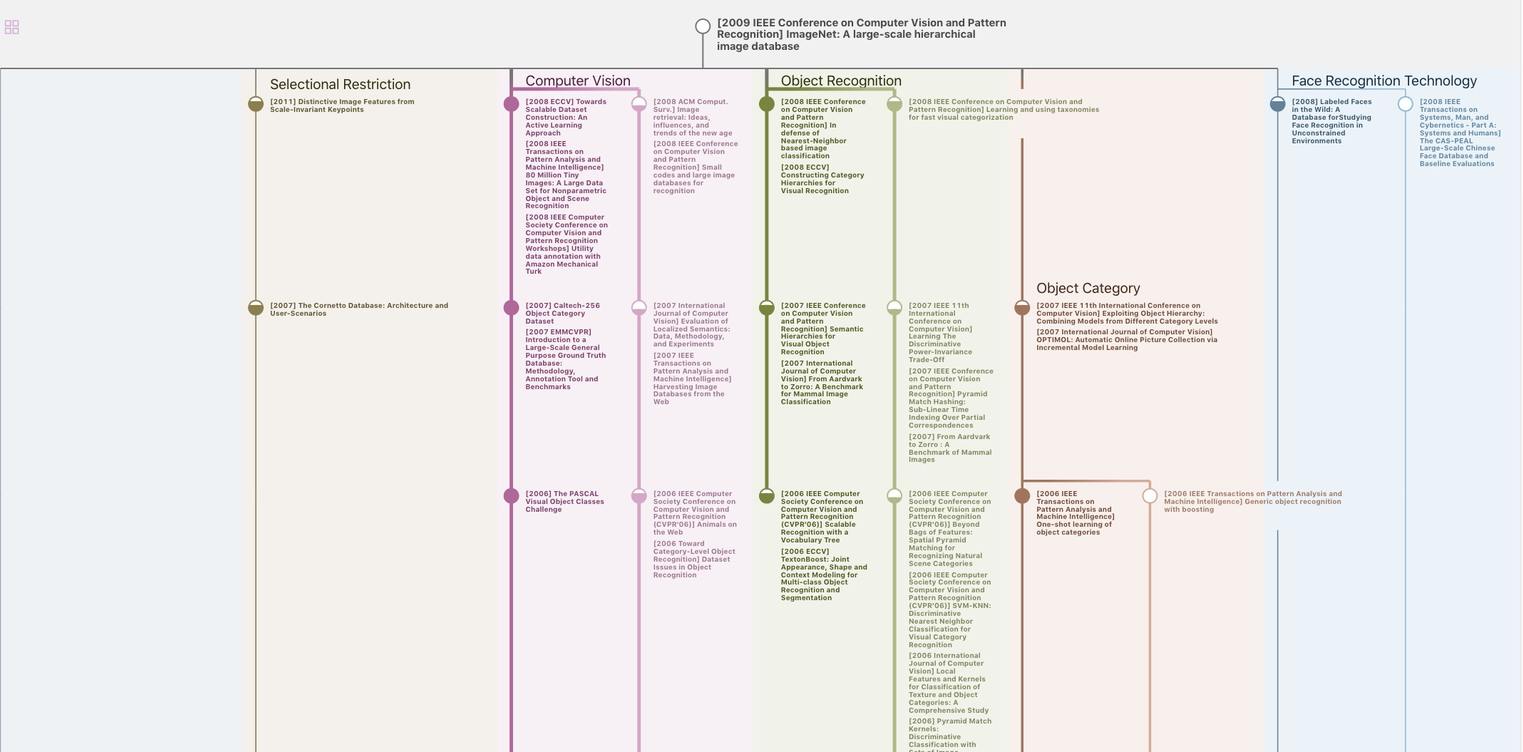
生成溯源树,研究论文发展脉络
Chat Paper
正在生成论文摘要