FairShare: An Incentive-Based Fairness-Aware Data Sharing Framework for Federated Learning.
ICIRA (2)(2023)
摘要
Federated learning protects sensitive data during AI model training, enabling collaboration without sharing raw data. Ensuring fairness and addressing un-shared decisions are crucial for reliable federated learning. This study introduces “FairShare”, an incentive mechanism enhancing reliability in applications like financial fraud detection and chatbot customer service. FairShare has two stages: stage one uses the Vickrey-Clarke-Groves (VCG) auction to estimate clients’ true costs, ensuring truthfulness, individual rationality, and computational efficiency. Stage two employs the Shapley Value method to allocate fair payments, promoting high-quality data usage. FairShare encourages clients to balance local datasets, reducing bias and increasing reliability. Theoretical proofs and experiments demonstrate FairShare’s effectiveness in ensuring fairness and protecting data privacy, leading to reliable outcomes. Experiments confirm the VCG-based mechanism’s truthfulness and Shapley Value’s fairness in payment allocation. In conclusion, FairShare addresses fairness challenges and fosters reliability in federated learning, facilitating dependable human-machine interactions. Implementing FairShare can enhance sensitive data protection, promote fairness, and improve reliability in human-machine interactions, supporting federated learning adoption across sectors.
更多查看译文
关键词
sharing,incentive-based,fairness-aware
AI 理解论文
溯源树
样例
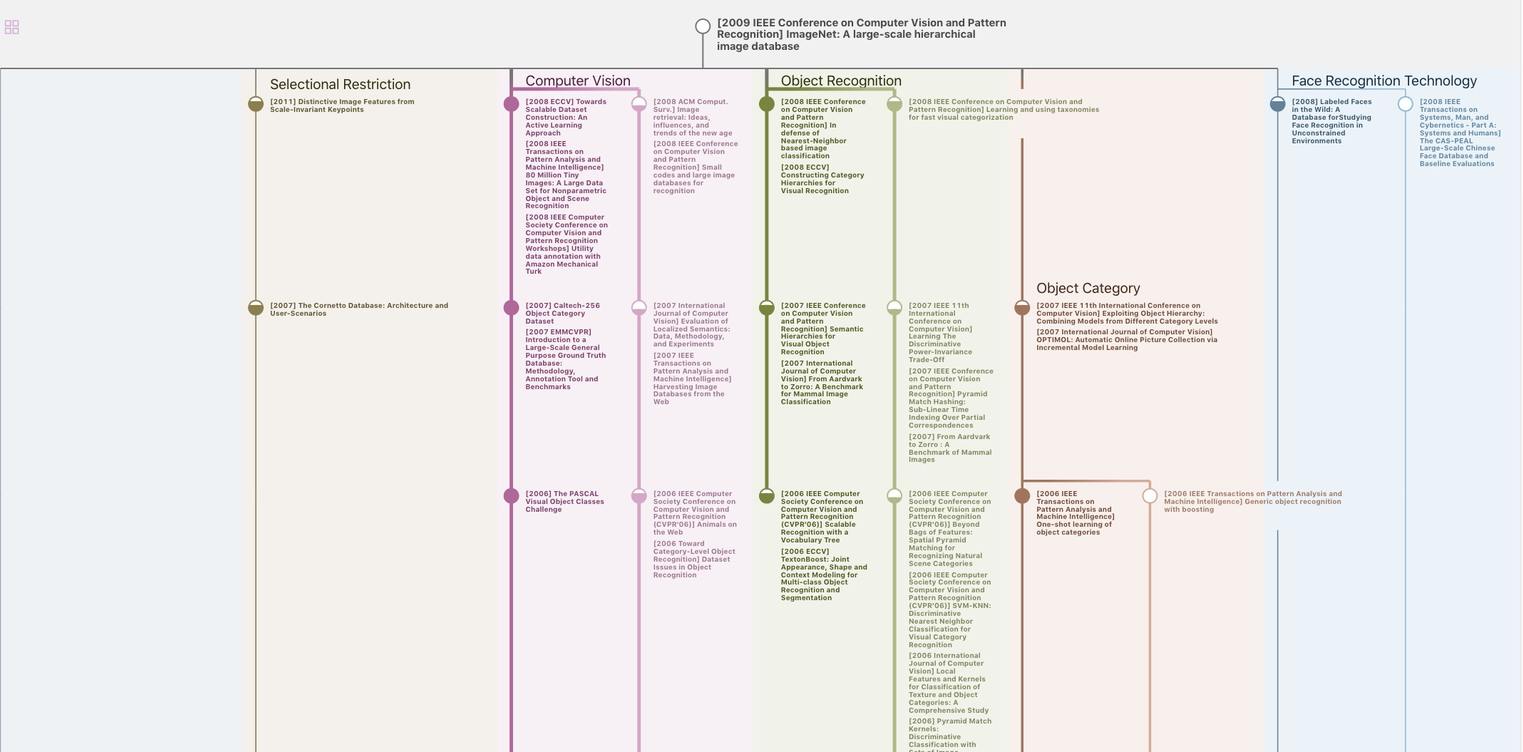
生成溯源树,研究论文发展脉络
Chat Paper
正在生成论文摘要