Embedded Temporal Feature Selection for Time Series Forecasting Using Deep Learning
ADVANCES IN COMPUTATIONAL INTELLIGENCE, IWANN 2023, PT II(2023)
摘要
Traditional time series forecasting models often use all available variables, including potentially irrelevant or noisy features, which can lead to overfitting and poor performance. Feature selection can help address this issue by selecting the most informative variables in the temporal and feature dimensions. However, selecting the right features can be challenging for time series models. Embedded feature selection has been a popular approach, but many techniques do not include it in their design, including deep learning methods, which can lead to less efficient and effective feature selection. This paper presents a deep learning-based method for time series forecasting that incorporates feature selection to improve model efficacy and interpretability. The proposed method uses a multidimensional layer to remove irrelevant features along the temporal dimension. The resulting model is compared to several feature selection methods and experimental results demonstrate that the proposed approach can improve forecasting accuracy while reducing model complexity.
更多查看译文
关键词
feature selection,embedded,neural network,time series,forecasting
AI 理解论文
溯源树
样例
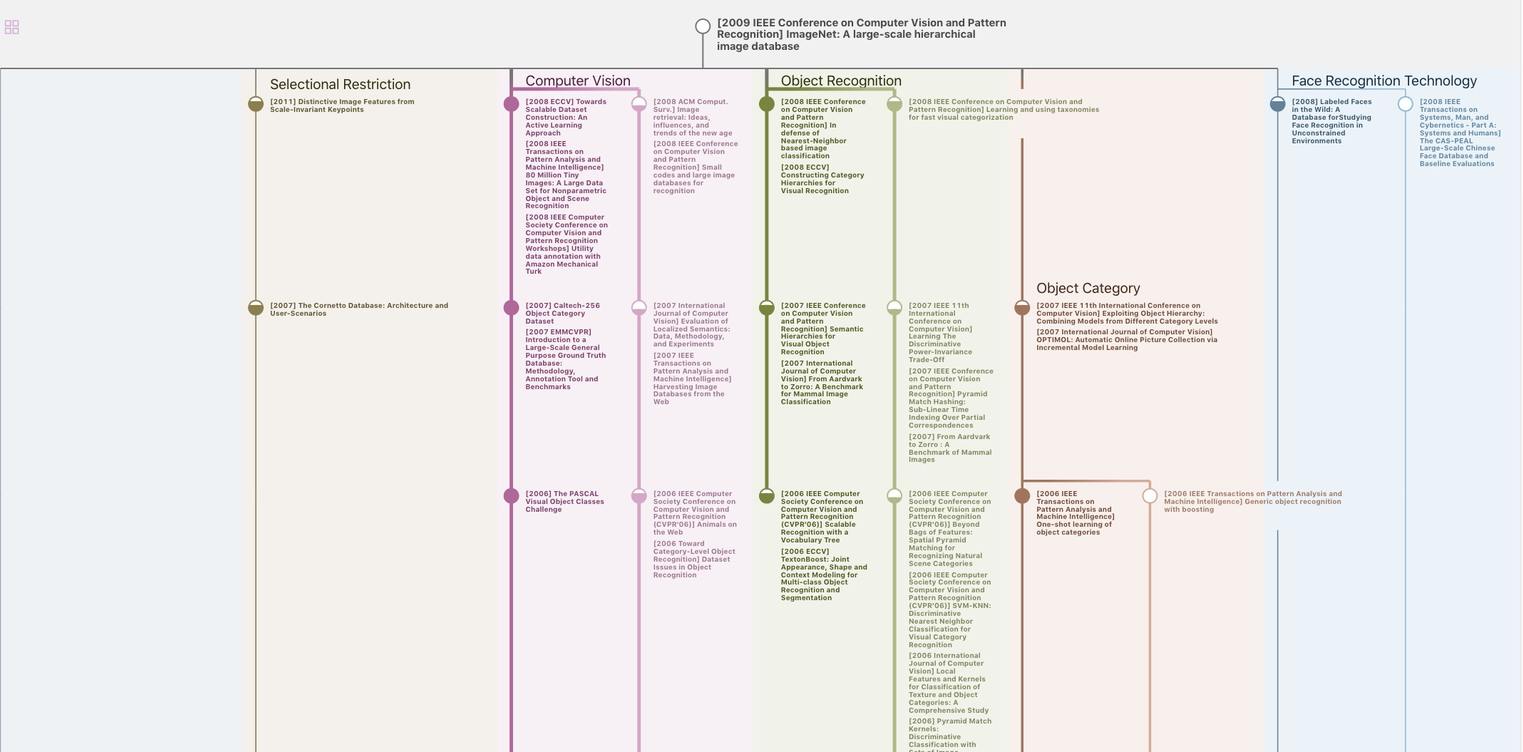
生成溯源树,研究论文发展脉络
Chat Paper
正在生成论文摘要