Temporal Dynamics of Drowsiness Detection Using LSTM-Based Models
ADVANCES IN COMPUTATIONAL INTELLIGENCE, IWANN 2023, PT I(2023)
摘要
Different LSTM-based models were tested for binary drowsiness detection using the ULg Multimodality Drowsiness Database (DROZY). The dataset contains physiological signals and behavioral measures collected from participants during different experimental conditions designed to induce varying levels of drowsiness. The LSTM models were trained using a sequential approach using the inter-beat intervals, where they were exposed to increasing levels of drowsiness over time. The performance of the models was evaluated in terms of accuracy, precision, recall, F1-score, and AUC. The results showed that the stacked bidirectional LSTM model achieved the highest performance with an accuracy of 0.873, precision of 0.825, recall of 0.793, F1-score of 0.808, and AUC of 0.918. These findings suggest that LSTM-based models can learn to capture the temporal dynamics of drowsiness and make accurate predictions based on the current and previous levels of drowsiness.
更多查看译文
关键词
Drowsiness Detection,LSTM,Recurrent Neural Networks,ECG,HRV
AI 理解论文
溯源树
样例
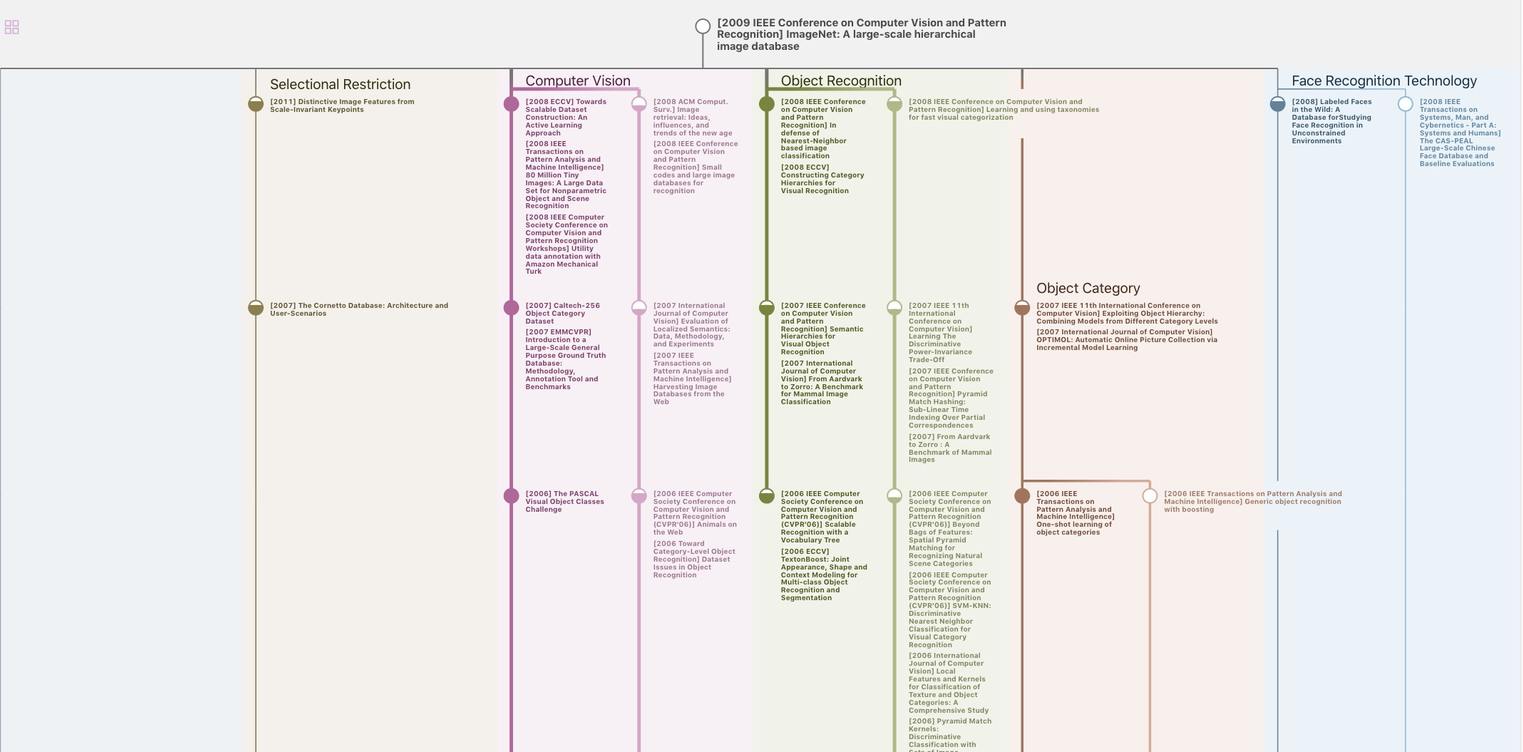
生成溯源树,研究论文发展脉络
Chat Paper
正在生成论文摘要