Data-Driven Prediction of Polymer Intrinsic Viscosity with Incomplete Time Series Data.
ICAC(2023)
摘要
Prediction of polymer intrinsic viscosity is very important for stabilizing the quality of polyester fibers. Data-driven prediction has been applied to solve engineering problems. However, incomplete data are all too frequent to a phenomenon in the workshop due to signal interference, human error, and other reasons. This paper proposes a data-driven approach for polymer intrinsic viscosity prediction with incomplete series data. First, a time series data generative adversarial network (TSDGAN) is presented to fill in the missing data, in which Attention LSTM serves as the generator and CNN as the discriminator. Next, An Informer model is introduced for polymer intrinsic viscosity prediction with the generated time series data. According to the experimental results under different missing rates, the proposed approach achieves better results than traditional and some state-of-the-art time-series prediction methods.
更多查看译文
关键词
Polymer Intrinsic Viscosity prediction,Incomplete data,GAN,Informer
AI 理解论文
溯源树
样例
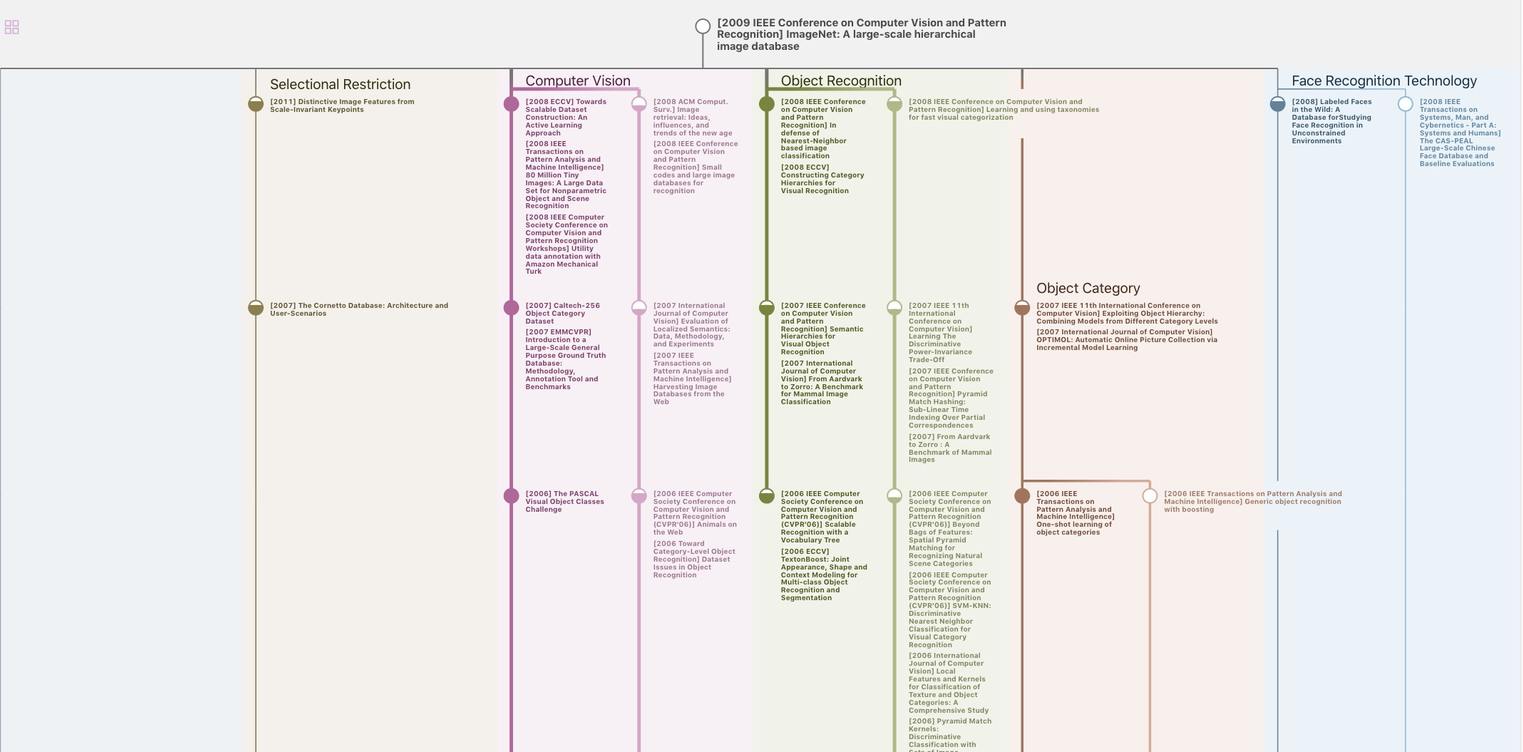
生成溯源树,研究论文发展脉络
Chat Paper
正在生成论文摘要