Joint Recurrent Actor-Critic Model for Partially Observable Control.
ICAC(2023)
摘要
In many real-world robotic control tasks, limited sensors with noise could affect the perceptual abilities of agents. Attempts to solve these partially observable (PO) control tasks using value-based deep reinforcement learning (RL) with recurrent neural networks (RNN) often suffer from two prominent problems: recurrent update inefficiency and overestimation bias of value approximation. In this paper, we propose an off-policy model-free deep RL algorithm, named joint recurrent actor-critic (JRAC), to tackle these two problems simultaneously. First, our algorithm develops the stored encoded state with an episode mask to implement step-based experience replay for efficient recurrent update. Then a pair of critics with independent LSTM parts is introduced to reduce overestimation. We further design a joint actor-critic architecture by sharing the RNN for the actor and the critic, which improves computational efficiency and accelerates the training process without causing intolerable instabilities. Our experiments show that the proposed algorithm can effectively deal with partial observability and performs well on several complicated PO control tasks.
更多查看译文
关键词
Reinforcement learning,partially observable control,experience replay,robotic control
AI 理解论文
溯源树
样例
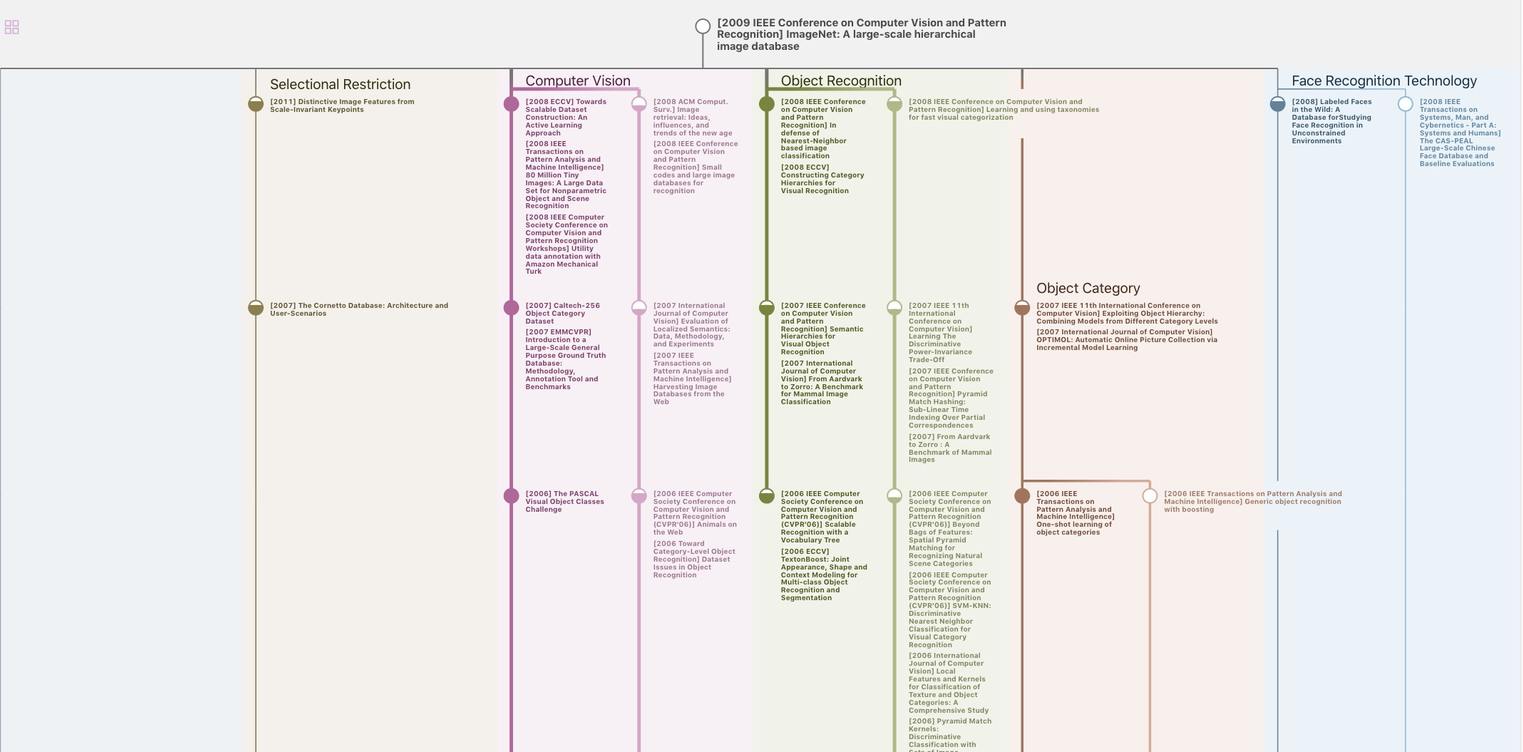
生成溯源树,研究论文发展脉络
Chat Paper
正在生成论文摘要