Ensembles of Classifiers and Quantifiers with Data Fusion for Quantification Learning.
DS(2023)
摘要
Quantification is a supervised Machine Learning task that estimates the class distribution in an unlabeled test set. Quantification has practical applications in various fields, including medical research, environmental monitoring, and quality control. For instance, medical research often estimates the prevalence of a particular disease in a population. Despite being a thriving research area, most existing quantification methods are limited to binary-class problems. Moreover, recent experimental evidence suggests that modern state-of-the-art quantifiers do not perform well for multi-class problems, which are prevalent in quantification. This paper proposes two novel multi-class ensemble quantifiers, FMC-SQ and FMC-MQ, that use data fusion methods at the classifier and quantifier levels. We conducted experiments with 12 state-of-the-art (single and ensemble) quantifiers to evaluate our models on 31 multi-class datasets. Our experimental results indicate that FMC-MQ is the best-performing quantifier outperforming other single and ensemble methods. Also, aggregating quantifier outputs seem to be a more promising research direction than aggregating classification scores for quantification.
更多查看译文
关键词
data fusion,classifiers,quantification,quantifiers,learning
AI 理解论文
溯源树
样例
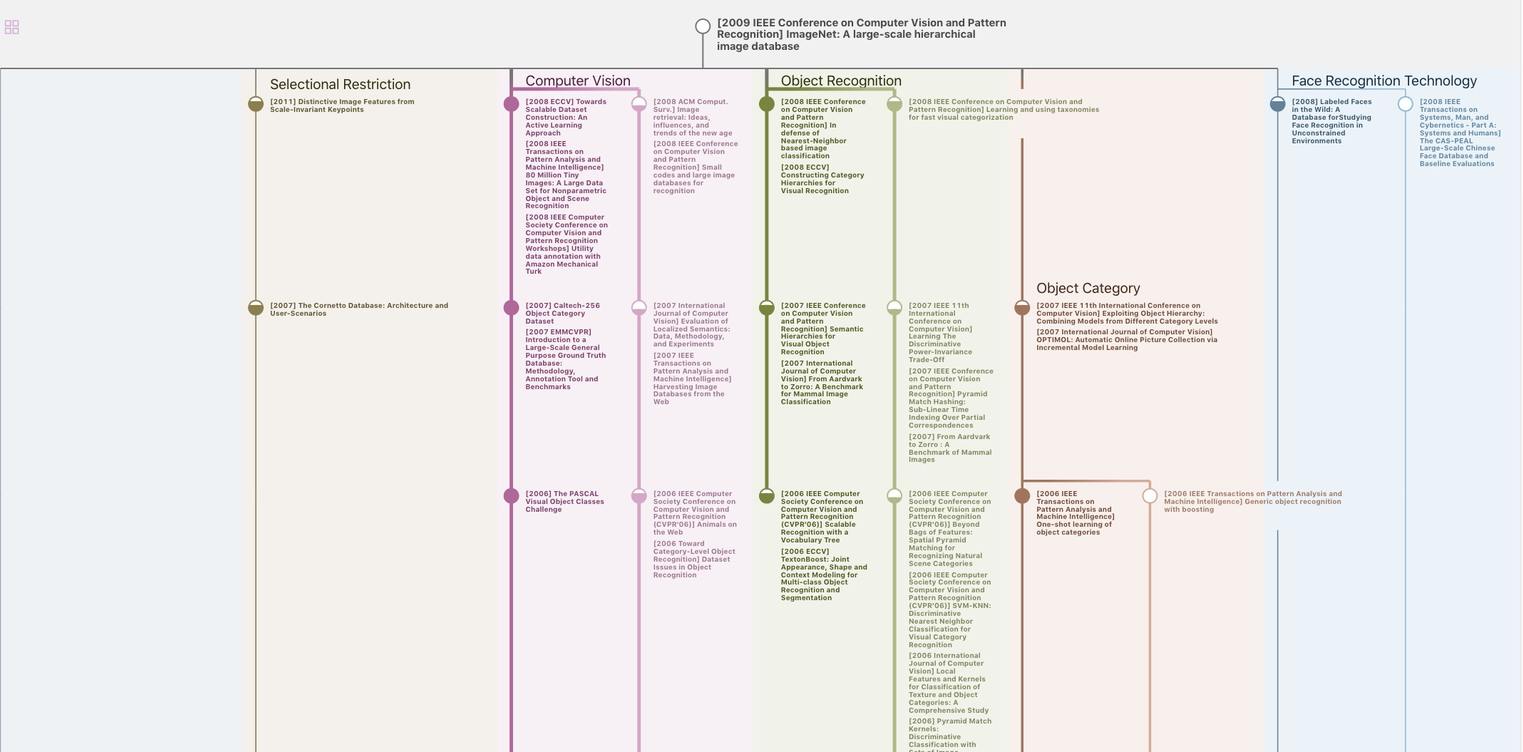
生成溯源树,研究论文发展脉络
Chat Paper
正在生成论文摘要