A Universal Approach for Post-correcting Time Series Forecasts: Reducing Long-Term Errors in Multistep Scenarios.
DS(2023)
摘要
Time series forecasting is an important problem with various applications in different domains. Improving forecast performance has been the center of investigation in the last decades. Several research studies have shown that old statistical method, such as ARIMA, are still state-of-the-art in many domains and applications. However, one of the main limitations of these methods is their low performance in longer horizons in multistep scenarios. We attack this problem from an entirely new perspective. We propose a new universal post-correction approach that can be applied to fix the problematic forecasts of any forecasting model, including ARIMA. The idea is intuitive: We query the last window of observations plus the given forecast, searching for similar “shapes” in the history, and using the future shape of the nearest neighbor, we post-correct the estimates. To ensure that post-correction is adequate, we train a meta-model on the successfulness of post-corrections on the training set. Our experiments on three diverse time series datasets show that the proposed method effectively improves forecasts for 30 steps ahead and beyond.
更多查看译文
关键词
errors,post-correcting,long-term
AI 理解论文
溯源树
样例
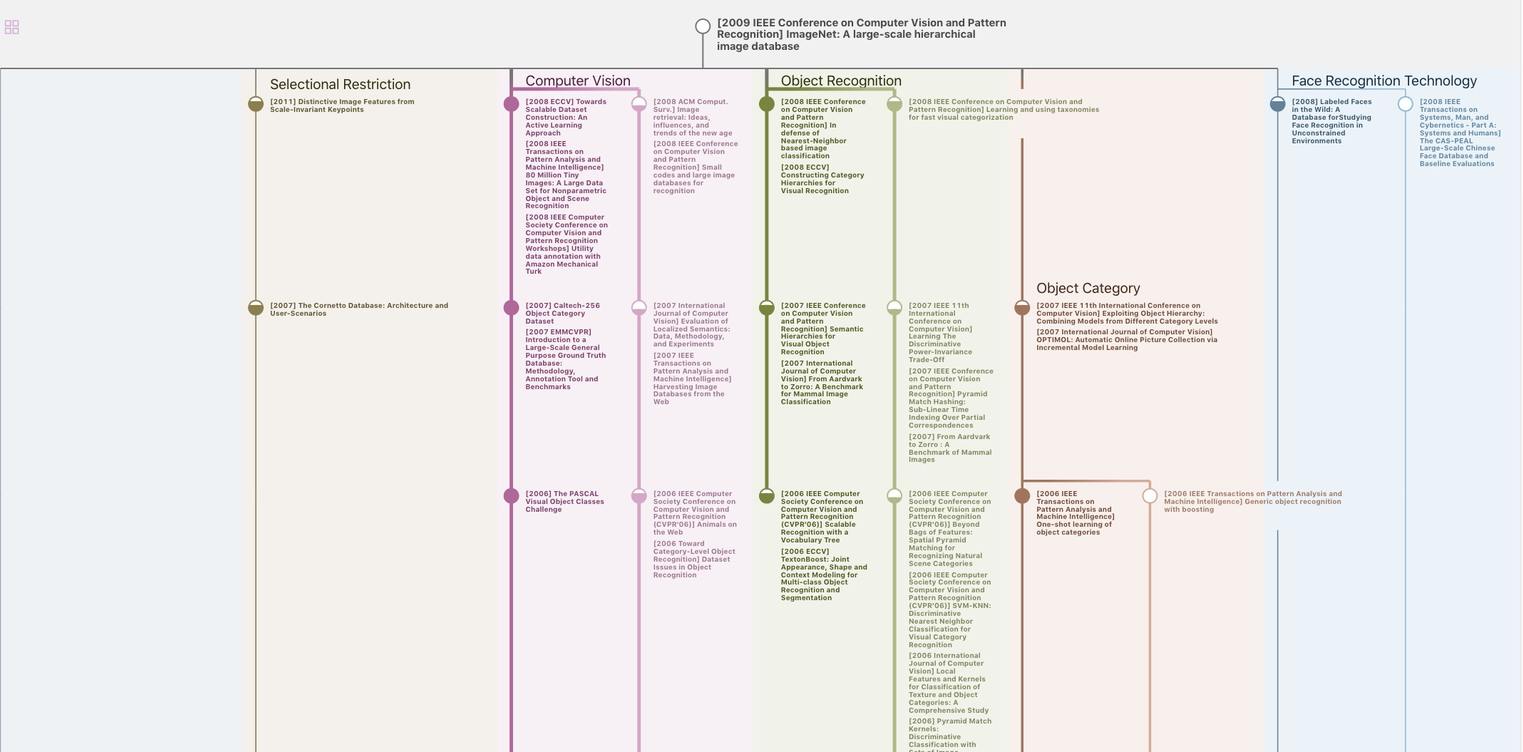
生成溯源树,研究论文发展脉络
Chat Paper
正在生成论文摘要