Towards Unified Modality Understanding for Alzheimer's Disease Diagnosis Using Incomplete Multi-modality Data
MACHINE LEARNING IN MEDICAL IMAGING, MLMI 2023, PT II(2024)
摘要
Multi-modal neuroimaging data, e.g., magnetic resonance imaging (MRI) and positron emission tomography (PET), has greatly advanced computer-aided diagnosis of Alzheimer's disease (AD) and its prodromal stage, i.e., mild cognitive impairment (MCI). However, incomplete multi-modality data often limits the diagnostic performance of deep learning-based methods, as only partial data can be used for training neural networks, and meanwhile it is challenging to synthesize missing scans (e.g., PET) with meaningful patterns associated with AD. To this end, we propose a novel unified modality understanding network to directly extract discriminative features from incomplete multi-modal data for AD diagnosis. Specifically, the incomplete multi-modal neuroimages are first branched into the corresponding encoders to extract modality-specific features and a Transformer is then applied to adaptively fuse the incomplete multi-modal features for AD diagnosis. To alleviate the potential problem of domain shift due to incomplete multi-modal input, the cross-modality contrastive learning strategy is further leveraged to align the incomplete multi-modal features into a unified embedding space. On the other hand, the proposed network also employs inter-modality and intra-modality attention weights for achieving local- to-local and local-to-global attention consistency so as to better transfer the diagnostic knowledge from one modality to another. Meanwhile, we leverage multi-instance attention rectification to rectify the localization of AD-related atrophic area. Extensive experiments on ADNI datasets with 1,950 subjects demonstrate the superior performance of the proposed methods for AD diagnosis and MCI conversion prediction.
更多查看译文
关键词
Alzheimer's Disease,Unified Modality Understanding,Cross-modality Contrastive Learning,Attention Consistency,Multi-Instance
AI 理解论文
溯源树
样例
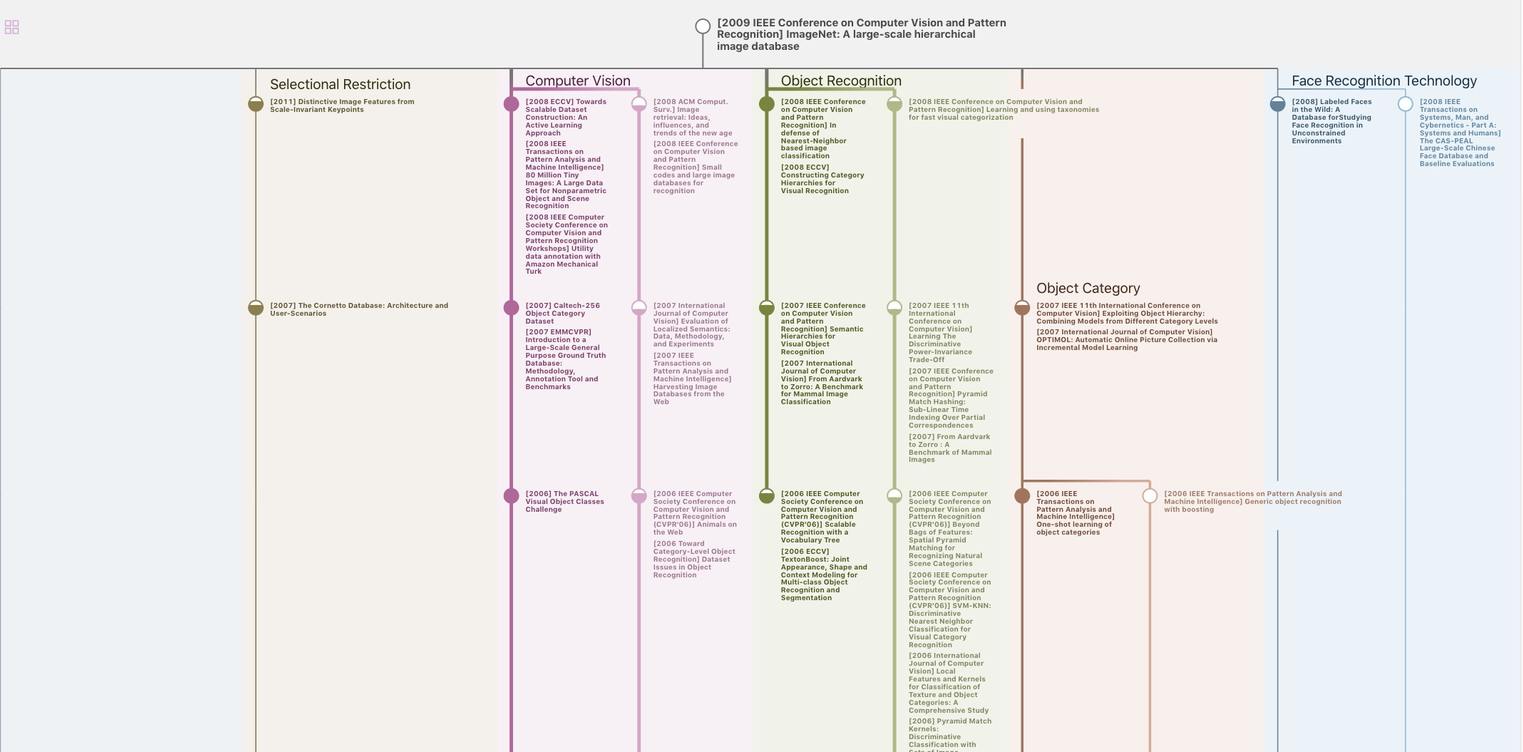
生成溯源树,研究论文发展脉络
Chat Paper
正在生成论文摘要