Implicitly Solved Regularization for Learning-Based Image Registration
MACHINE LEARNING IN MEDICAL IMAGING, MLMI 2023, PT I(2024)
摘要
Deformable image registration is a fundamental step in many medical image analysis tasks and has attracted a large amount of research to develop efficient and accurate unsupervised machine learning approaches. Although much attention has been paid to finding suitable similarity measures, network architectures and training methods, much less research has been devoted to suitable regularization techniques to ensure the plausibility of the learned deformations. In this paper, we propose implicitly solved regularizers for unsupervised and weakly supervised learning of deformable image registration. In place of pure gradient descent with automatic differentiation, we combine efficient implicit solvers for the regularization term with the established gradient-based optimization regarding the network parameters. As a result, our approach is broadly applicable and can be combined with a range of similarity measures and network architectures. Our experiments with state-of-the-art network architectures show that the proposed approach has the potential to increase the smoothness, i.e. the plausibility, of the learned deformations and the registration accuracy measured as dice overlaps. Furthermore, we show that due to efficient GPU implementations of the implicit solvers, this increase in plausibility and accuracy comes at almost no additional cost in terms of computational time.
更多查看译文
关键词
image registration,regularization,implicit solvers
AI 理解论文
溯源树
样例
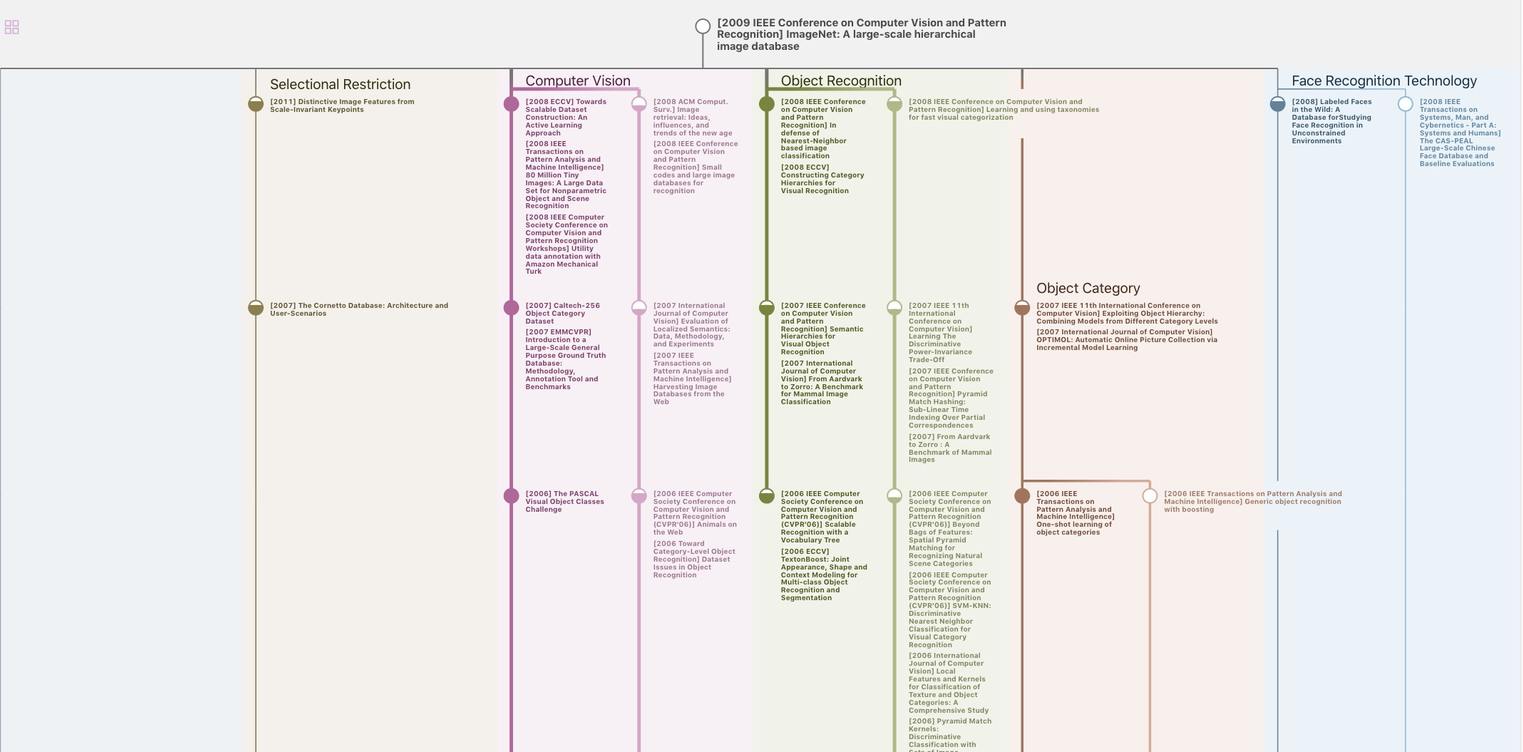
生成溯源树,研究论文发展脉络
Chat Paper
正在生成论文摘要