Tailoring Large Language Models to Radiology: A Preliminary Approach to LLM Adaptation for a Highly Specialized Domain
MACHINE LEARNING IN MEDICAL IMAGING, MLMI 2023, PT I(2024)
摘要
In this preliminary work, we present a domain fine-tuned LLM model for radiology, an experimental large language model adapted for radiology. This model, created through an exploratory application of instruction tuning on a comprehensive dataset of radiological information, demonstrates promising performance when compared with broader language models such as StableLM, Dolly, and LLaMA. This model exhibits initial versatility in applications related to radiological diagnosis, research, and communication. Our work contributes an early but encouraging step towards the evolution of clinical NLP by implementing a large language model that is local and domain-specific, conforming to stringent privacy norms like HIPAA. The hypothesis of creating customized, large-scale language models catering to distinct requirements of various medical specialties, presents a thought-provoking direction. The blending of conversational prowess and specific domain knowledge in these models kindles hope for future enhancements in healthcare AI. While it is still in its early stages, the potential of generative large language models is intriguing and worthy of further exploration. The demonstration code of our domain fine-tuned LLM model for radiology can be accessed at https://anonymous.4open.science/r/radiology-llm-demo-C3E2/.
更多查看译文
关键词
Large Language Models,Natural Language Processing,Radiology
AI 理解论文
溯源树
样例
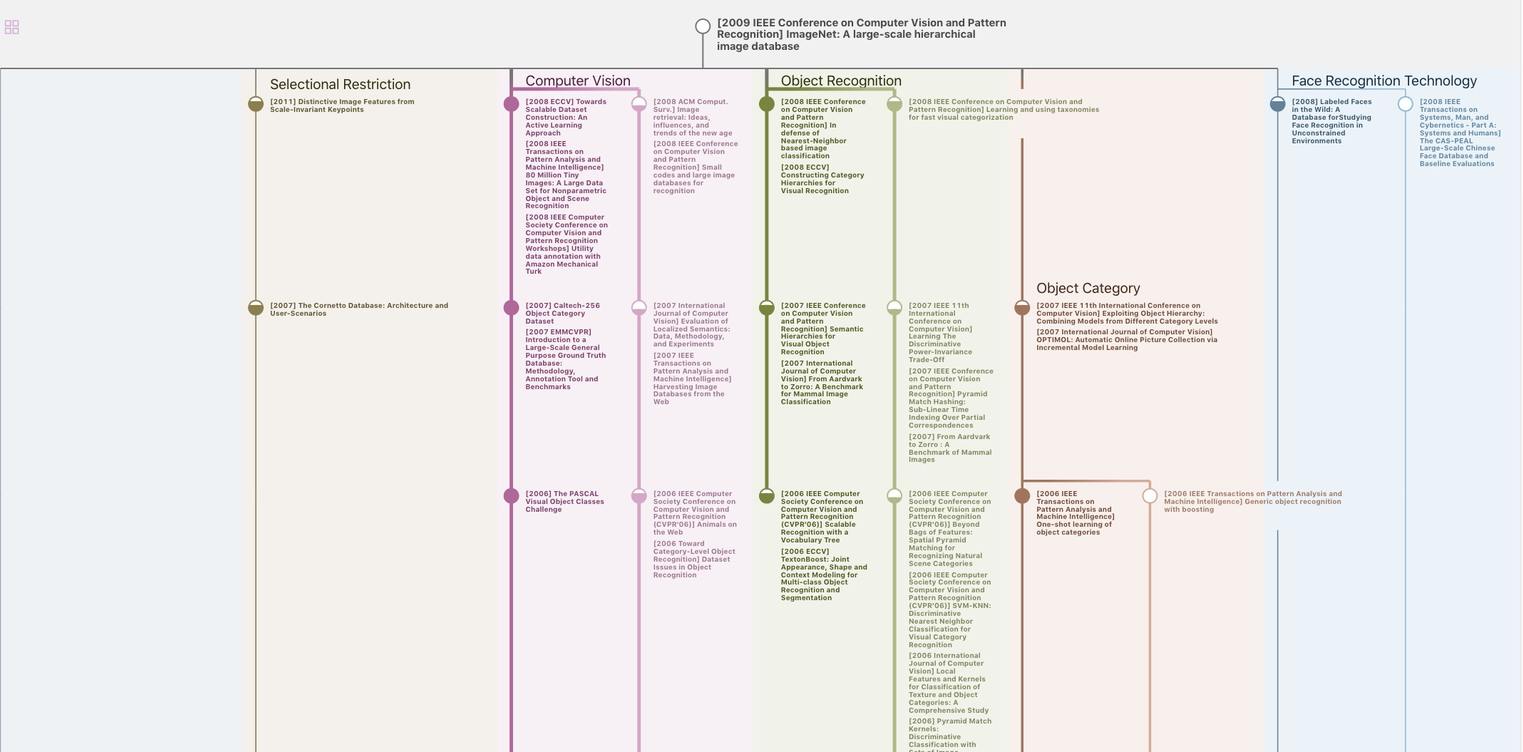
生成溯源树,研究论文发展脉络
Chat Paper
正在生成论文摘要