CCMix: Curriculum of Class-Wise Mixup for Long-Tailed Medical Image Classification
MACHINE LEARNING IN MEDICAL IMAGING, MLMI 2023, PT II(2024)
摘要
Deep learning-based methods have been widely used for medical image classification. However, in clinical practice, rare diseases are usually underrepresented with limited labeled data, which result in long-tailed medical datasets and significantly degrade the performance of deep classification networks. Previous strategies employ re-sampling or reweighting techniques to alleviate this issue by increasing the influence of underrepresented classes and reducing the influence of overrepresented ones. Still, poor performance may occur due to overfitting of the tail classes. Further, Mixup is employed to introduce additional information into model training. Despite considerable improvements, the significant noise in medical images means that random batch mixing may introduce ambiguity into training, thereby impair the performance. This observation motivates us to develop a fine-grained mixing approach. In this paper we present Curriculum of Class-wise Mixup (CCMix), a novel method for addressing the challenge of long-tailed distributions. CCMix leverages a novel curriculum that takes into account both the degree of mixing and the class-wise performance to identify the ideal Mixup proportions of different classes. Our method's simplicity enables its effortless integration with existing long-tailed recognition techniques. Comprehensive experiments on two long-tailed medical image classification datasets demonstrate that our method, requiring no modifications to the framework structure or algorithmic details, achieves state-of-the-art results across diverse long-tailed classification benchmarks. The source code is available at https://github.com/sirileeee/CCMix.
更多查看译文
关键词
Long-tailed Learning,Medical Image Classification,Mixup
AI 理解论文
溯源树
样例
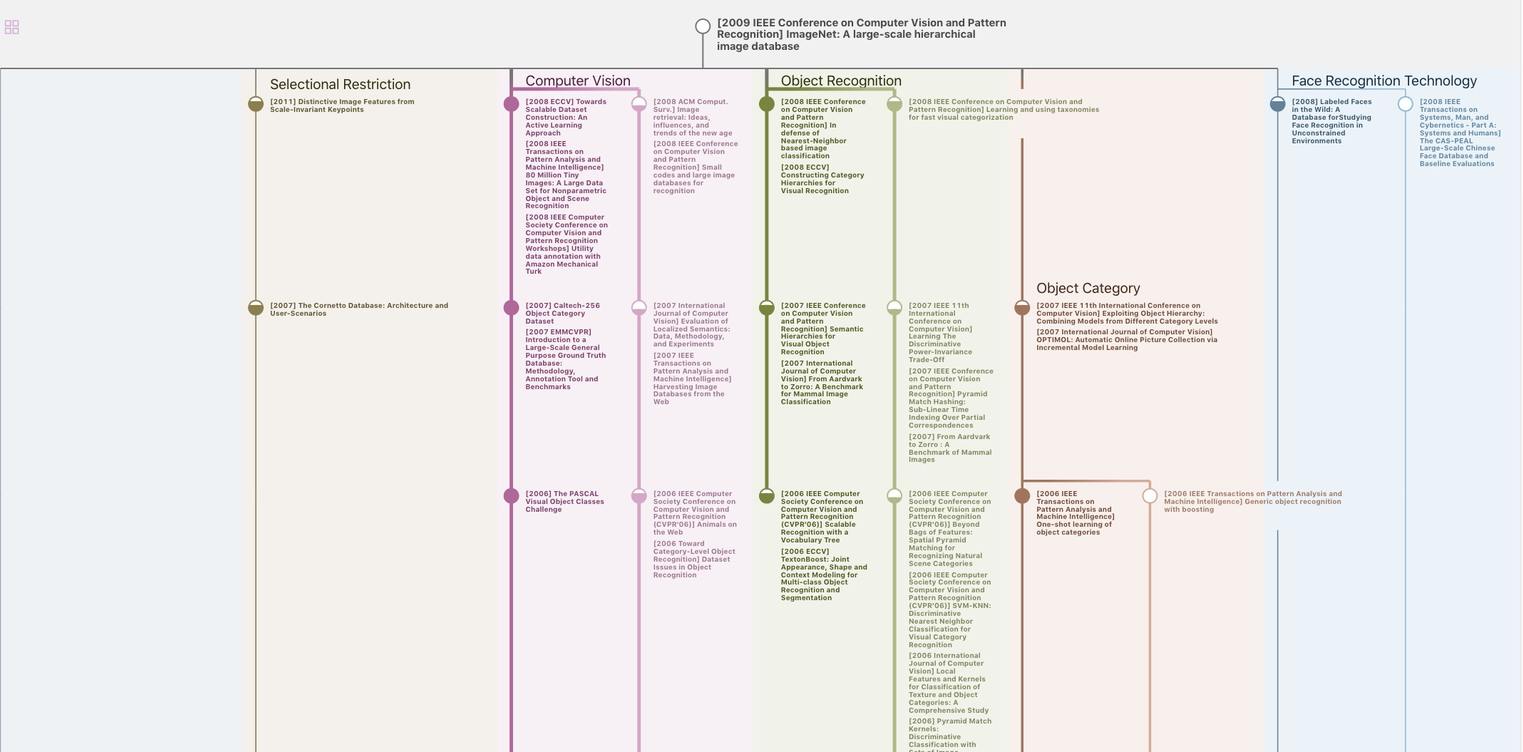
生成溯源树,研究论文发展脉络
Chat Paper
正在生成论文摘要