MEDKD: Enhancing Medical Image Classification with Multiple Expert Decoupled Knowledge Distillation for Long-Tail Data
MACHINE LEARNING IN MEDICAL IMAGING, MLMI 2023, PT II(2024)
摘要
Medical image classification is a challenging task, particularly when dealing with long-tailed datasets where rare diseases are underrepresented. The imbalanced class distribution in such datasets poses significant challenges in accurately classifying minority classes. Existing methods for alleviating the long-tail problem in medical image classification suffer from limitations such as noise introduction, loss of crucial information, and the need for manual tuning and additional computational resources. In this study, we propose a novel framework called Multiple Expert Decoupled Knowledge Distillation (MEDKD) to tackle the imbalanced class distribution in medical image classification. The knowledge distillation of multiple teacher models can significantly alleviate the class imbalance by partitioning the dataset into several subsets. However, current frameworks of this kind have not yet explored the integration of more advanced distillation methods. Our framework incorporating TCKD and NCKD concepts to improve classification performance. Through comprehensive experiments on publicly available datasets, we evaluate the performance of MEDKD and compare it with state-of-the-art methods. Our results demonstrate remarkable accuracy improvements achieved by the proposed method, highlighting its effectiveness in alleviating the challenges of medical image classification with long-tailed datasets.
更多查看译文
关键词
Long-tailed Classification,Knowledge Distillation,Multiple Expert
AI 理解论文
溯源树
样例
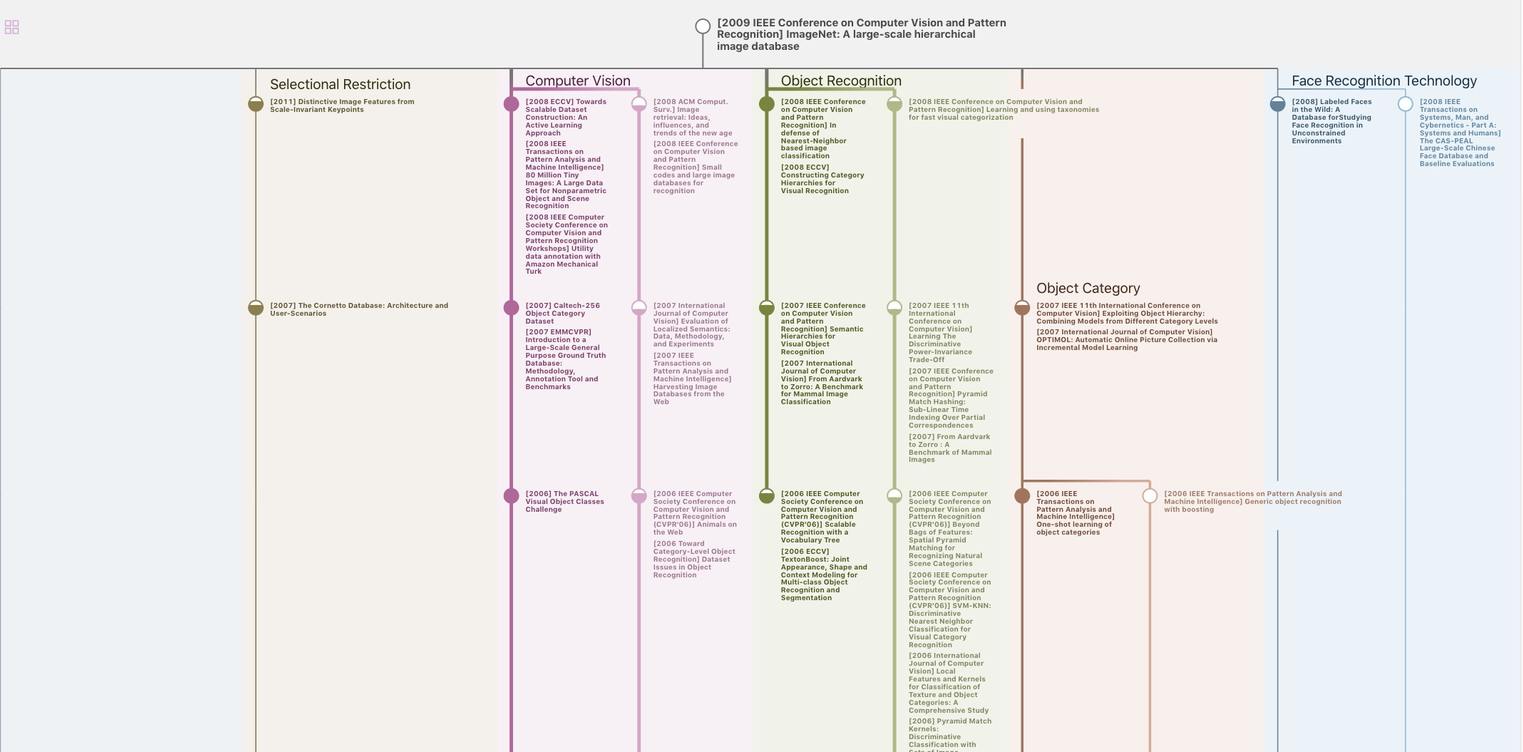
生成溯源树,研究论文发展脉络
Chat Paper
正在生成论文摘要