Efficient Federated Learning with Adaptive Client-Side Hyper-Parameter Optimization.
ICDCS(2023)
摘要
Federated Learning (FL) trains machine learning (ML) models with privacy protection. However, current FL algorithms use the same hyper-parameters for all clients regardless of their statistical or system heterogeneity, leading to slower convergence. Convergence time and communication rounds may be reduced by using appropriate values for hyper-parameters like learning rate and epochs. We present an adaptive client-side hyper-parameter optimization algorithm, FedAdap, that uses metrics gathered during model training to optimize hyper-parameters on each client and can be used in conjunction with any other FL algorithm. Preliminary results show that FedAdap enhances the performance of existing FL algorithms by decreasing convergence time by up to 34% and reducing communication rounds by up to 37% in the case of IID data. Moreover, in non-IID data settings, the convergence time is reduced by up to 82.5% and the number of communication rounds is reduced by up to 77%.
更多查看译文
关键词
Federated Learning,Hyperparameter Optimization,Convergence,Communication cost
AI 理解论文
溯源树
样例
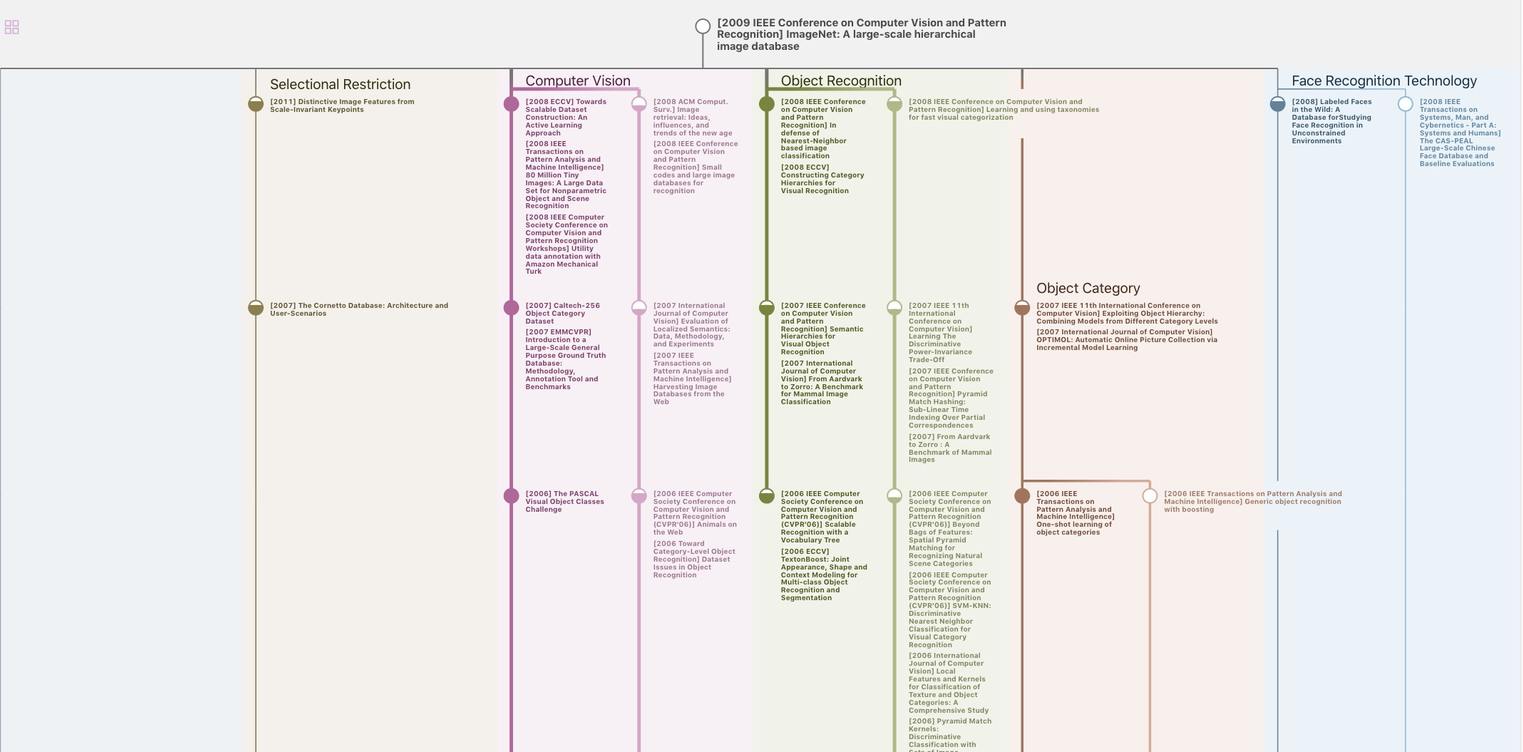
生成溯源树,研究论文发展脉络
Chat Paper
正在生成论文摘要