Normative Aging for an Individual's Full Brain MRI Using Style GANs to Detect Localized Neurodegeneration
MACHINE LEARNING IN MEDICAL IMAGING, MLMI 2023, PT II(2024)
摘要
In older adults, changes in brain structure can be used to identify and predict the risk of neurodegenerative disorders and dementias. Traditional 'brainAge' methods seek to identify differences between chronological age and biological brain age predicted from MRI that can indicate deviations from normative aging trajectories. These methods provide one metric for the entire brain and lack anatomical specificity and interpretability. By predicting an individual's healthy brain at a specific age, one may be able to identify regional deviations and abnormalities in a true brain scan relative to healthy aging. This study aims to address the problem of domain transfer in estimating age-related brain differences. We develop a fully unsupervised generative adversarial network (GAN) with cycle consistency reconstruction losses, trained on 4,000 cross-sectional brain MRI data from UK Biobank participants aged 60 to 80. By converting the individual anatomic information from their T1-weighted MRI as "content" and adding the "style" information related to age and sex from a reference group, we demonstrate that brain MRIs for healthy males and females at any given age can be predicted from one cross-sectional scan. Pairedwith a full brain T1w harmonizationmethod, this new MRI can also be generated for any image from any scanner. Results in the ADNI cohort showed that without relying on longitudinal data from the participants, our style-encoding domain transfer model might successfully predict cognitively normal follow-up brain MRIs. We demonstrate how variations from the expected structure are a sign of a potential risk for neurodegenerative diseases.
更多查看译文
关键词
domain transfer,GAN,aging patterns,brain MRI
AI 理解论文
溯源树
样例
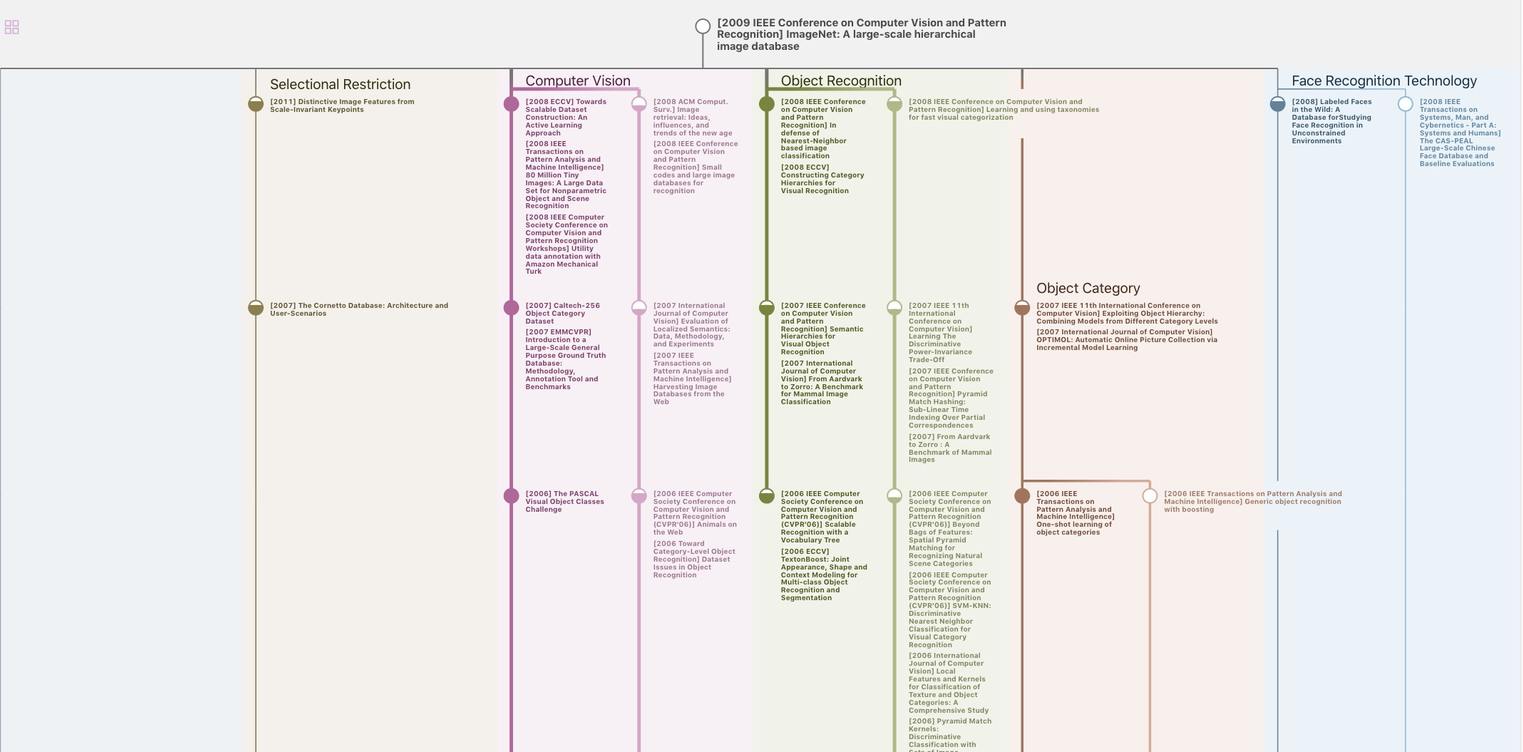
生成溯源树,研究论文发展脉络
Chat Paper
正在生成论文摘要