Dual-Stream Model with Brain Metrics and Images for MRI-Based Fetal Brain Age Estimation
MACHINE LEARNING IN MEDICAL IMAGING, MLMI 2023, PT I(2024)
摘要
The disparity between chronological age and estimated brain age from images is a significant indicator of abnormalities in brain development. However, MRI-based brain age estimation still encounters considerable challenges due to the unpredictable movement of the fetus and maternal abdominal motions, leading to fetal brain MRI scans of extremely low quality. In this work, we propose a novel deep learning-based dual-stream fetal brain age estimation framework, involving brain metrics and images. Given a stack of MRI data, we first locate and segment out brain regions of every slice. Since brain metrics are highly correlated with age, we introduce four brain metrics into the model. To enhance the representational capacity of these metrics in space, we design them as vector-based discrete spatial metrics(DSM). Then we design the 3D-FetalNet and DSM-Encoder to extract visual and metric features respectively. Additionally, we apply the Global and local regression to enable the model to learn various patterns across different age ranges. We evaluate our model on a fetal brain MRI dataset with 238 subjects and reach the age estimation error of 0.75 weeks. Our proposed method achieves state-of-the-art results compared with other models.
更多查看译文
关键词
MRI-based fetal brain age estimation,Brain metrics,Discrete spatial metircs,DSM-Encoder,3D-FetalNet
AI 理解论文
溯源树
样例
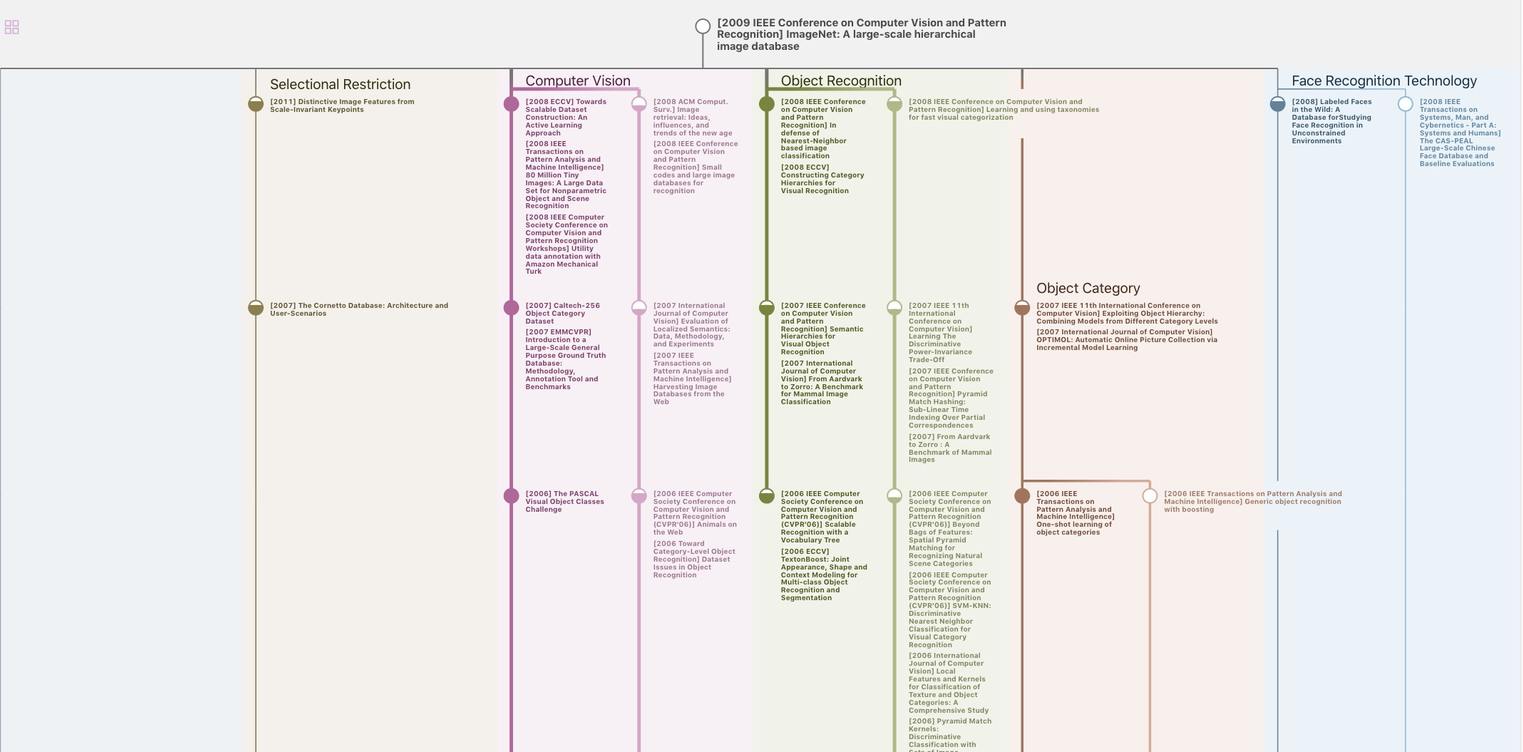
生成溯源树,研究论文发展脉络
Chat Paper
正在生成论文摘要