FedDM: Data and Model Heterogeneity-Aware Federated Learning via Dynamic Weight Sharing.
ICDCS(2023)
摘要
Federated Learning (FL) plays an indispensable role in edge computing systems. Prevalent FL methods mainly address challenges involved in heterogeneous data distribution across devices. Model heterogeneity, however, has seldom been put under scrutiny. In practice, different devices (e.g., PCs and smartphones) generally have disparate computation and communication resources, necessitating neural network models with varying parameter sizes. Therefore, we propose FedDM, a novel data and model heterogeneity-aware FL system, which improves the FL system's accuracy while reducing edge devices' computation and communication costs for heterogeneous model training. FedDM features: 1) dynamic weight sharing scheme that handles model heterogeneity by dynamically selecting parts of the large model to share with smaller ones; 2) tree-structured layer-wise client cooperation scheme that handles data heterogeneity by allowing clients with similar data distribution to share some network layers. We implement FedDM and evaluate it using five public datasets with different tasks.
更多查看译文
关键词
Federated Learning, Data Heterogeneity, Model Heterogeneity, Parameter Sharing
AI 理解论文
溯源树
样例
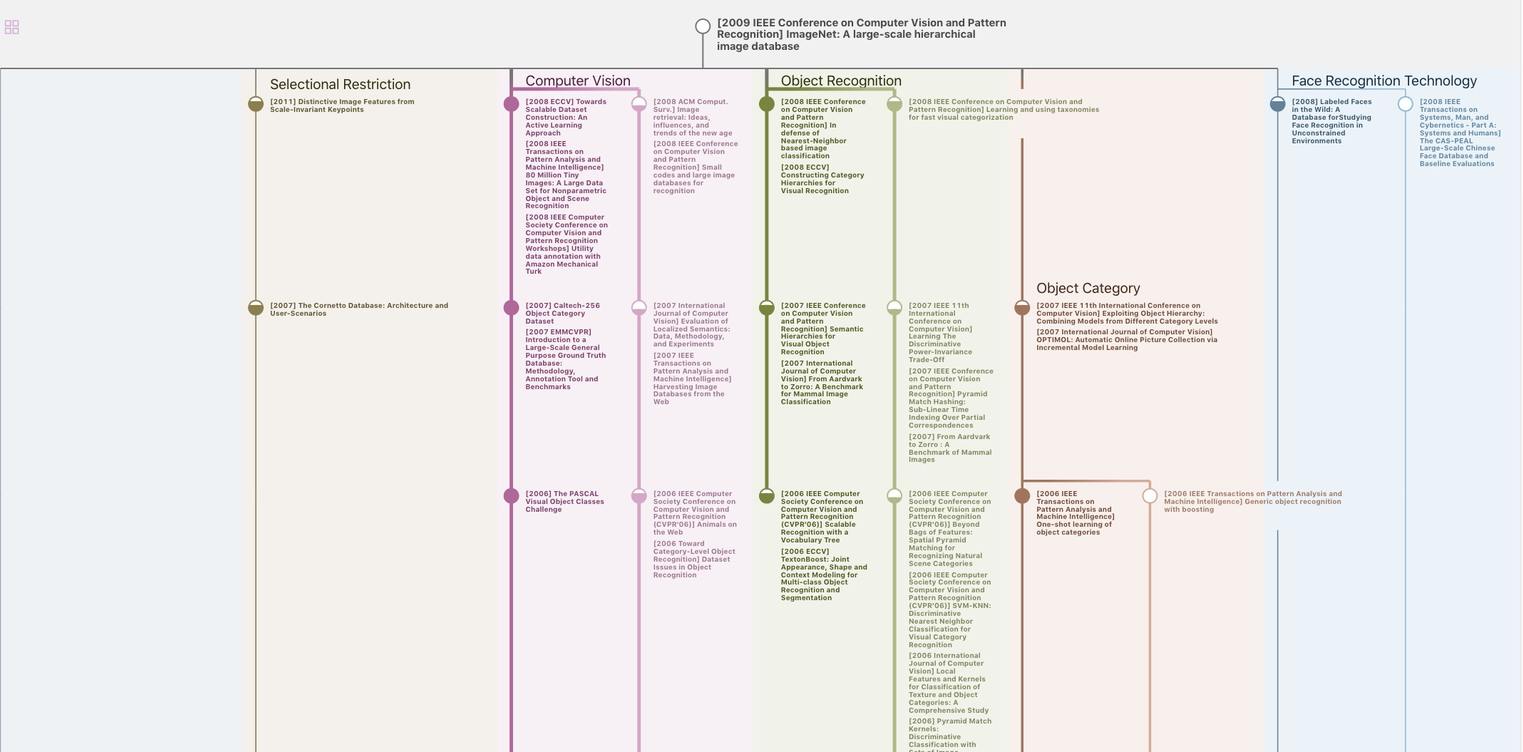
生成溯源树,研究论文发展脉络
Chat Paper
正在生成论文摘要