Fine-Grained Traffic Prediction of Communication-and-Collaboration Apps via Deep-Learning: a First Look at Explainability
ICC 2023 - IEEE INTERNATIONAL CONFERENCE ON COMMUNICATIONS(2023)
摘要
The lifestyle change originated from the COVID-19 pandemic has caused a measurable impact on Internet traffic in terms of volume and application mix, with a sudden increase in usage of communication-and-collaboration apps. In this work, we focus on four of these apps (Skype, Teams, Webex, and Zoom), whose traffic we collect, reliably label at fine (i.e. peractivity) granularity, and analyze from the viewpoint of traffic prediction. The outcome of this analysis is informative for a number of network management tasks, including monitoring, planning, resource provisioning, and (security) policy enforcement. To this aim, we employ state-of-the-art multitask deep learning approaches to assess to which degree the traffic generated by these apps and their different use cases (i.e. activities: audio-call, video-call, and chat) can be forecast at packet level. The experimental analysis investigates the performance of the considered deep learning architectures, in terms of both traffic-prediction accuracy and complexity, and the related trade-off. Equally important, our work is a first attempt at interpreting the results obtained by these predictors via eXplainable Artificial Intelligence (XAI).
更多查看译文
关键词
communication apps,collaboration apps,COVID-19,deep learning,encrypted traffic,multitask approaches,traffic prediction,XAI
AI 理解论文
溯源树
样例
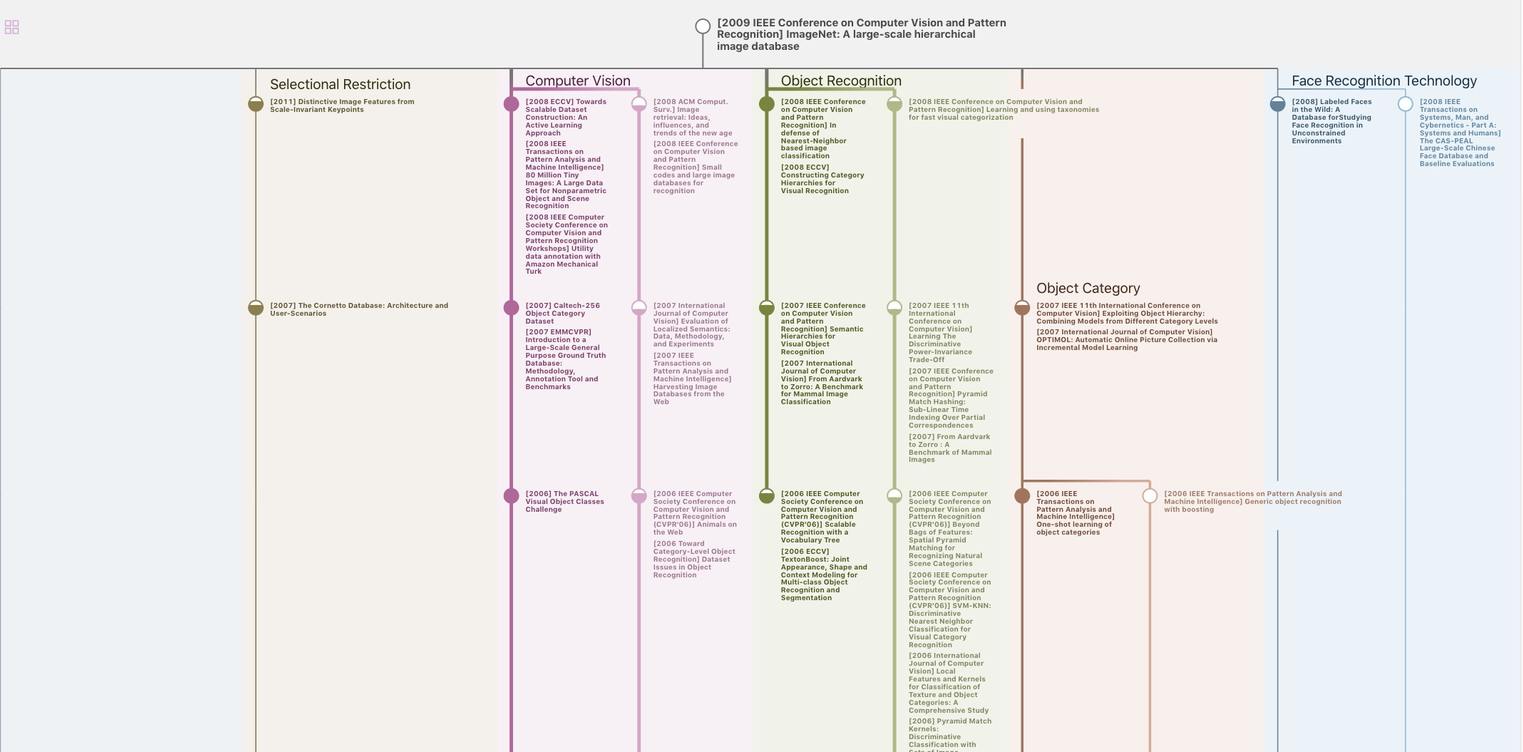
生成溯源树,研究论文发展脉络
Chat Paper
正在生成论文摘要