Cache Policy Design via Reinforcement Learning for Cellular Networks in Non-Stationary Environment
2023 IEEE INTERNATIONAL CONFERENCE ON COMMUNICATIONS WORKSHOPS, ICC WORKSHOPS(2023)
摘要
We consider wireless caching both at the network edge and at User Equipment (UE) to alleviate traffic congestion, aiming to find a joint cache placement and delivery policy by maximizing the Quality of Service (QoS) while minimizing backhaul load and User Equipment (UE) power consumption. We assume unknown and time-variant file popularities which are affected by the UE cache content, leading to a non-stationary Partial Observable Markov Decision Process (POMDP). We address this problem in a deep reinforcement learning framework, employing Feed Forward Neural Network (FFNN) and Long Short Term Memory (LSTM) networks in conjunction with Advantageous Actor Critic (A2C) algorithm. LSTM exploits the correlation of the file popularity distribution across time slots to learn information of the dynamics of the environment and A2C algorithm is used due to its ability of handling continuous and high dimensional spaces. We leverage LSTM and A2C tools based on its virtue to find an optimal solution for the POMDP environment. Simulation results show that using LSTMbased A2C outperforms a FFNN-based A2C in terms of sample efficiency and optimality. An LSTM-based A2C gives a superior performance under the non-stationary POMDP paradigm.
更多查看译文
关键词
Wireless caching,Deep Reinforcement Learning,Advantageous Actor Critic,Long Short Term Memory,NonStationary POMDP
AI 理解论文
溯源树
样例
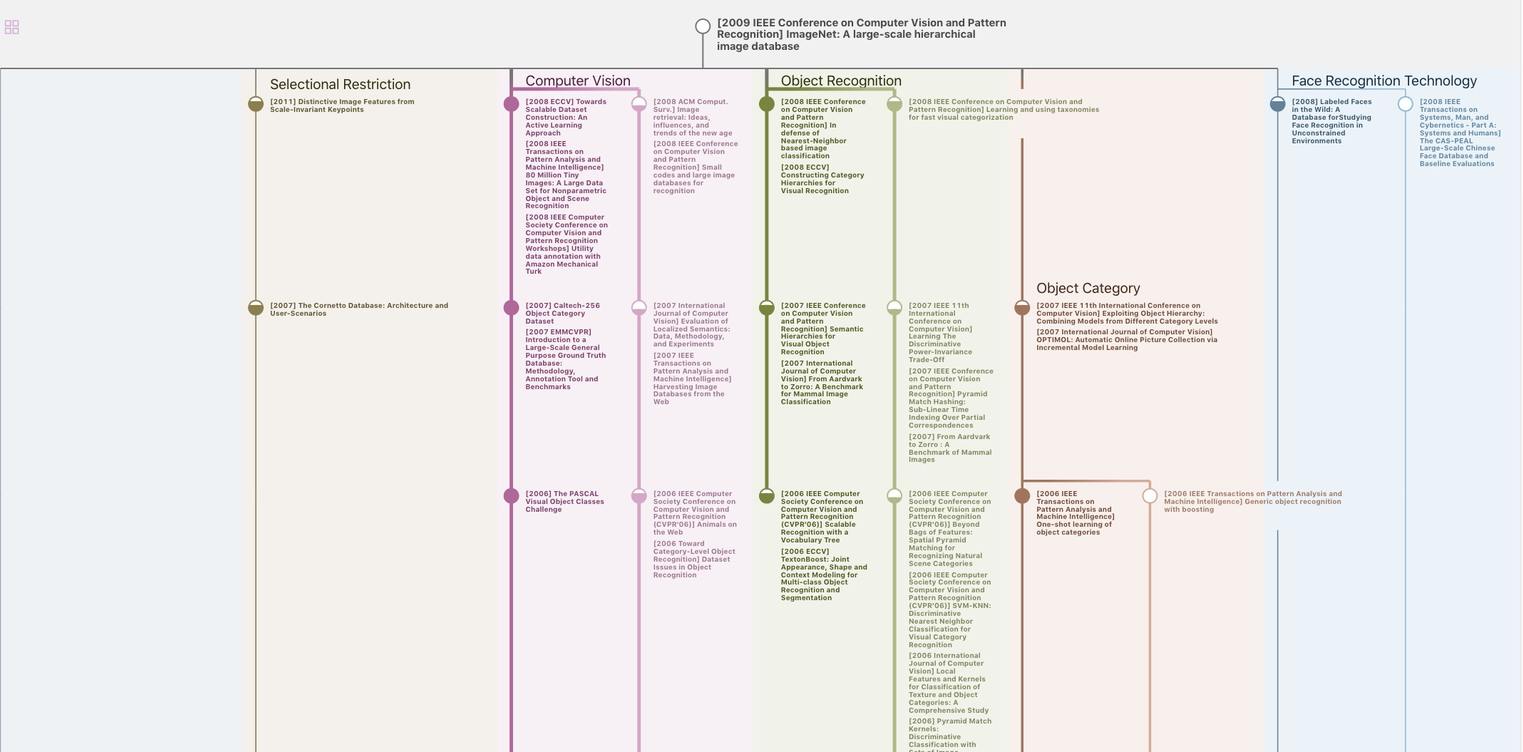
生成溯源树,研究论文发展脉络
Chat Paper
正在生成论文摘要