GBMIA: Gradient-based Membership Inference Attack in Federated Learning
ICC 2023 - IEEE INTERNATIONAL CONFERENCE ON COMMUNICATIONS(2023)
摘要
Membership inference attack (MIA) has been proved to pose a serious threat to federated learning (FL). However, most of the existing membership inference attacks against FL rely on the specific attack models built from the target model behaviors, which make the attacks costly and complicated. In addition, directly adopting the inference attacks that are originally designed for machine learning models into the federated scenarios can lead to poor performance. We propose GBMIA, an attack model-free membership inference method based on gradient. We take full advantage of the federated learning process by observing the target model's behaviors after gradient ascent tuning. And we combine prediction correctness and the gradient norm-based metric for membership inference. The proposed GBMIA can be conducted by both global and local attackers. We conduct experimental evaluations on three real-world datasets to demonstrate that GBMIA can achieve a high attack accuracy. We further apply the arbitration mechanism to increase the effectiveness of GBMIA which can lead to an attack accuracy close to 1 on all three datasets. We also conduct experiments to substantiate that clients going offline and the overlap of clients' training sets have great effect on the membership leakage in FL.
更多查看译文
关键词
Membership Inference Attack,Federated Learning,Membership Privacy,Privacy Leakage
AI 理解论文
溯源树
样例
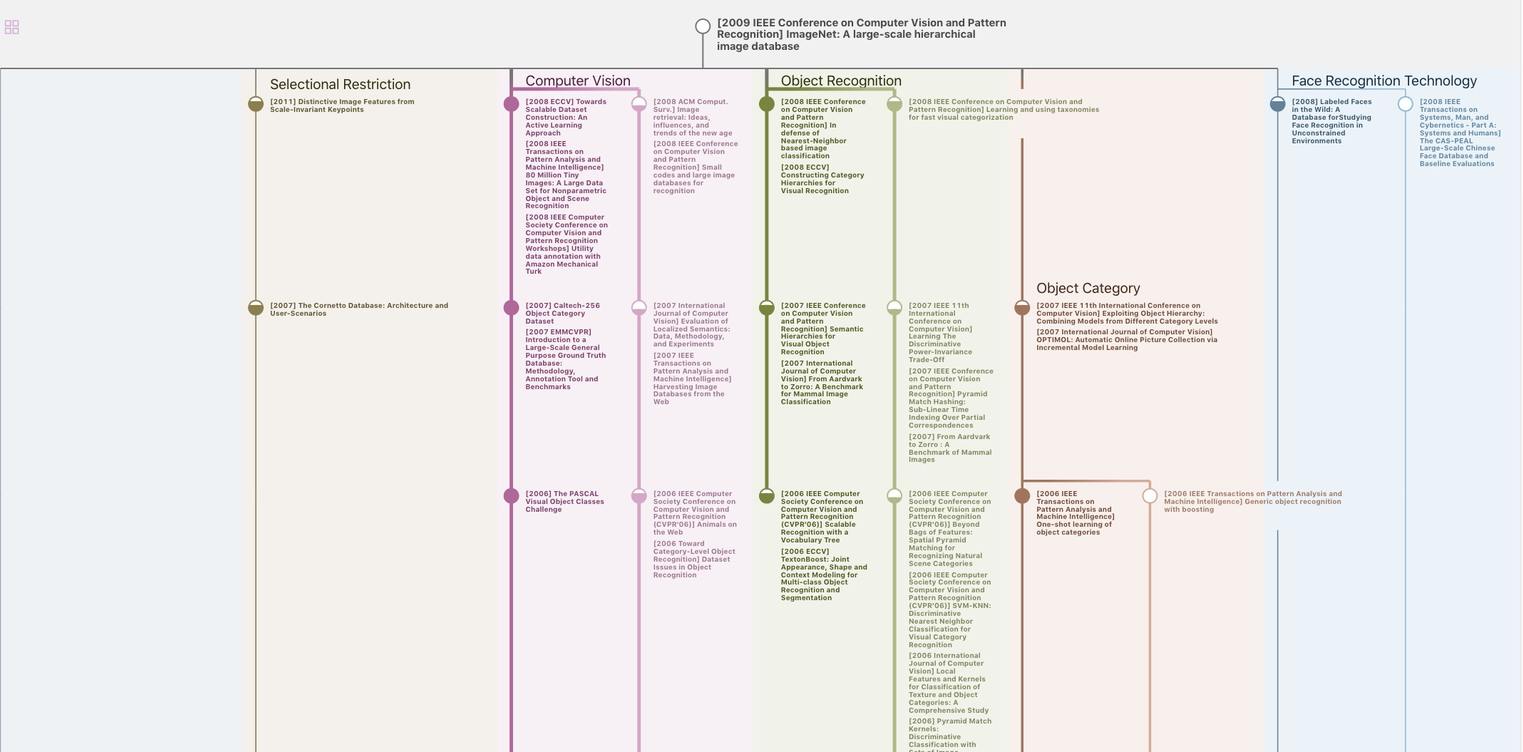
生成溯源树,研究论文发展脉络
Chat Paper
正在生成论文摘要