Graph Attention LSTM for Load Prediction of Fine-grained VNFs in SFC
ICC 2023 - IEEE INTERNATIONAL CONFERENCE ON COMMUNICATIONS(2023)
摘要
Service Function Chain (SFC) is composed of an ordered set of service functions, which are also noted as virtual network function (VNF) instances, to adaptively form a composite network service with increased flexibility and agility. One fundamental challenge in SFC run-time management is the accurate prediction of the resource load of SFC VNFs for proactive VNF resource provisioning. In the literature, existing works largely neglect the application level relationship for the fine-grained atom-VNFs in microservice architecture. In this work, we propose an end-to-end deep-learning-based prediction model, namely granularity-captured Graph Attention LSTM Network (GGAL), for accurate load prediction at the fine-grained atom-VNF level. By integrating granularity-captured graph attention layers, LSTM and MLP, the model is able to extract the application level spatio-temporal relationship among atom-VNFs and perform accurate load prediction. The effectiveness of the proposed GGAL model is demonstrated through intensive simulations by comparing to several baselines.
更多查看译文
关键词
Service Function Chain,Virtual Network Function,Load Prediction,GNN,LSTM
AI 理解论文
溯源树
样例
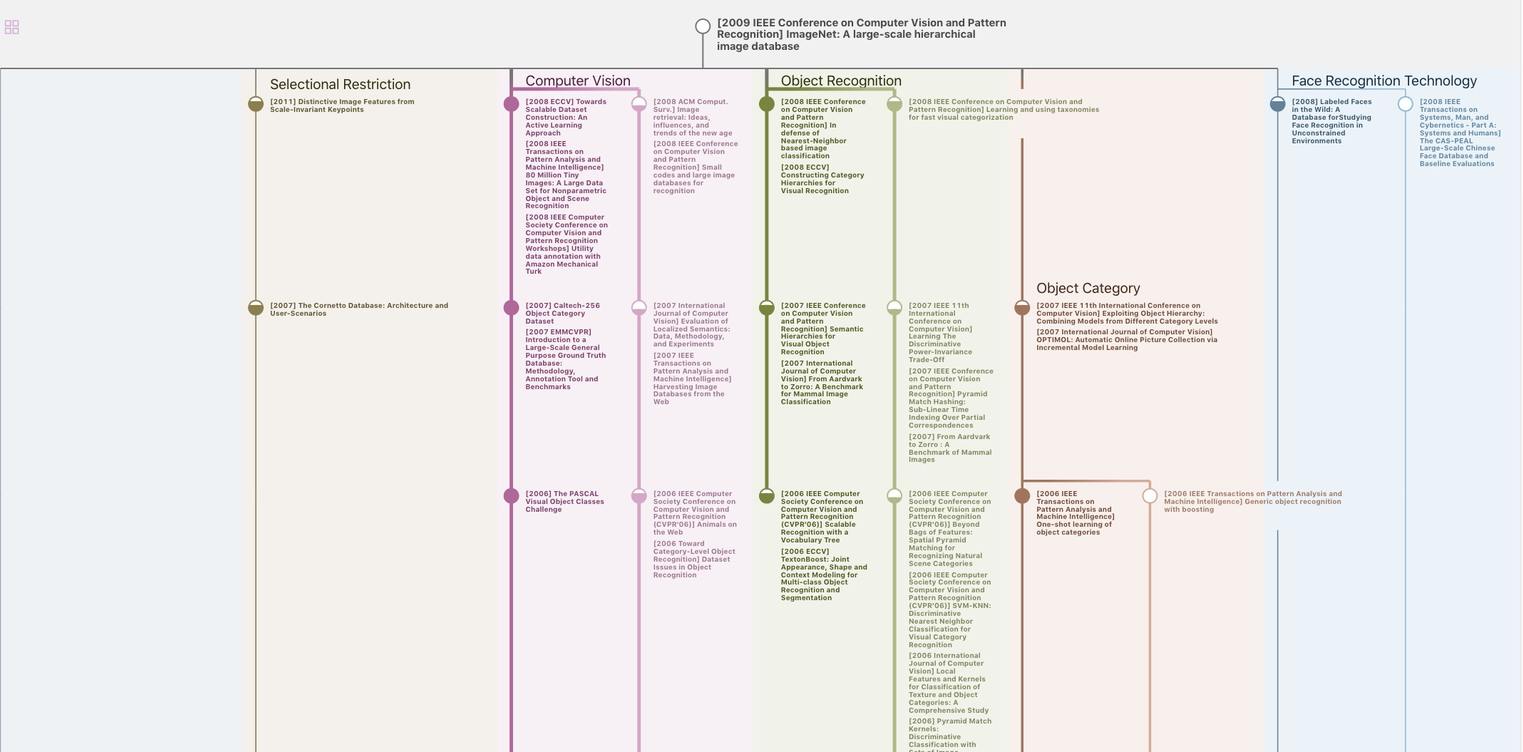
生成溯源树,研究论文发展脉络
Chat Paper
正在生成论文摘要