Difference Convex (DC) Programming Approach as an Alternative Optimizer for Neural Networks
ICC 2023 - IEEE INTERNATIONAL CONFERENCE ON COMMUNICATIONS(2023)
摘要
Artificial neural networks (NNs) are widely used in many modern applications, including signal processing and communication systems. Conventionally, NNs are trained by different forms of stochastic gradient descent (GD) algorithm. However, since NN optimization cost functions are non-convex, training NNs with GD has fundamental common shortcoming of all non-convex problems. To address this issue, in this paper, we take advantage of the difference of convex (DC) programming as an innovative approach for smooth/non-smooth non-convex optimizations. We model the training of NN as a DC problem and propose DC programming as an alternative optimization technique to find NN parameters. Furthermore, we obtains the convex components of the DC function. In particular, we efficiently compute convex components of regression and binary classification cost functions by means of convex analysis tools. We verify our proposed model by comparing its result with the conventional gradient descent optimizer. Simulation results confirm that the superiority of the proposed DC programming approach over GD.
更多查看译文
关键词
Deep neural network,convex analysis,difference convex optimization
AI 理解论文
溯源树
样例
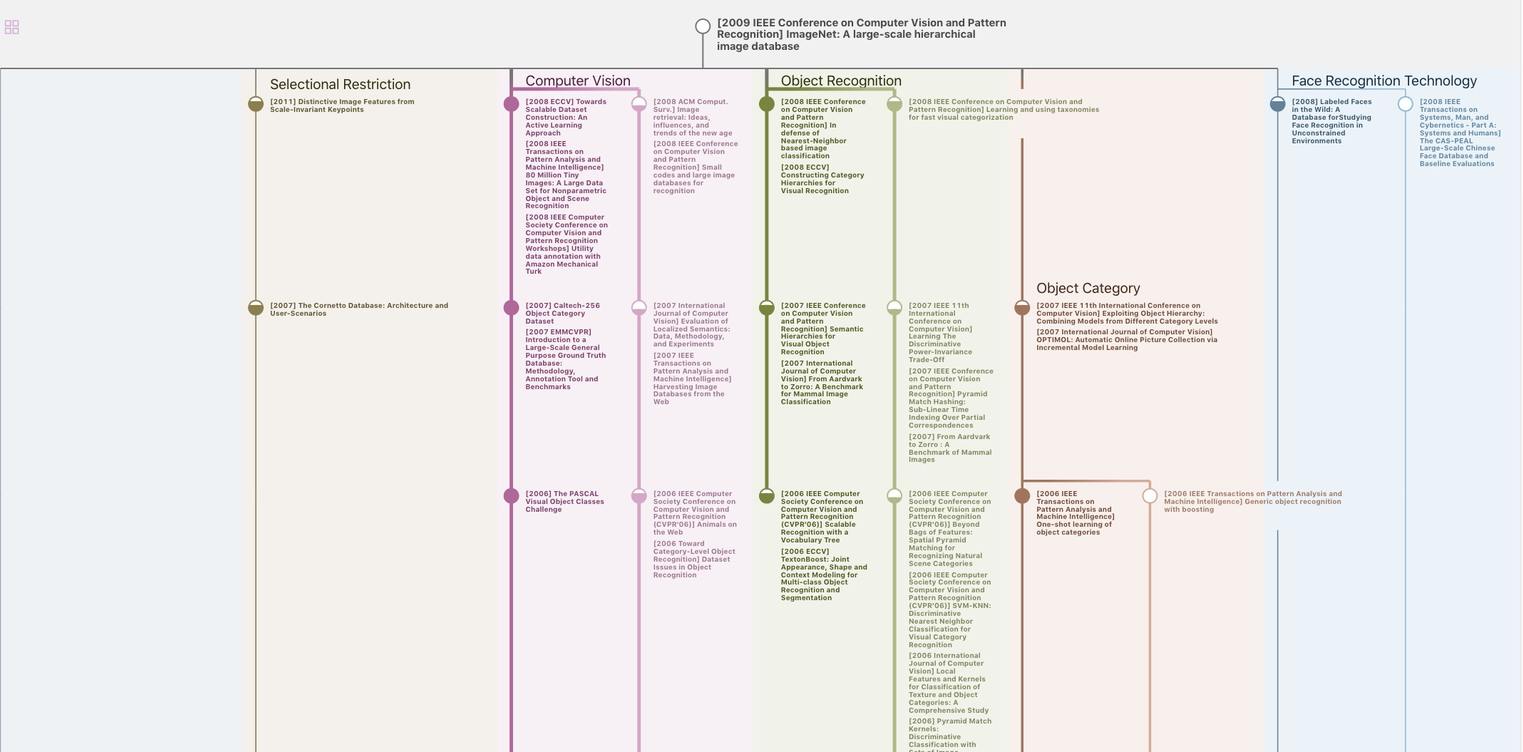
生成溯源树,研究论文发展脉络
Chat Paper
正在生成论文摘要