Communication-Efficient Privacy-Preserving Federated Learning via Knowledge Distillation for Human Activity Recognition Systems
ICC 2023 - IEEE INTERNATIONAL CONFERENCE ON COMMUNICATIONS(2023)
摘要
Emerging Internet of Things (IoT) applications, such as sensor-based Human Activity Recognition (HAR) systems, require efficient machine learning solutions due to their resource-constrained nature which raises the need to design heterogeneous model architectures. Federated Learning (FL) has been used to train distributed deep learning models. However, standard federated learning (fedAvg) does not allow the training of heterogeneous models.Our work addresses the model and statistical heterogeneities of distributed HAR systems. We propose a Federated Learning via Augmented Knowledge Distillation (FedAKD) algorithm for heterogeneous HAR systems and evaluate it on a self-collected sensor-based HAR dataset. Then, Kullback-Leibler (KL) divergence loss is compared with Mean Squared Error (MSE) loss for the Knowledge Distillation (KD) mechanism. Our experiments demonstrate that MSE contributes to a better KD loss than KL. Experiments show that FedAKD is communication-efficient compared with model-dependent FL algorithms and outperforms other KD-based FL methods under the i.i.d. and non-i.i.d. scenarios.
更多查看译文
关键词
Deep Learning,Federated Learning,Knowledge Distillation,Human Activity Recognition (HAR),Kullback-Leibler divergence,privacy-preserving AI
AI 理解论文
溯源树
样例
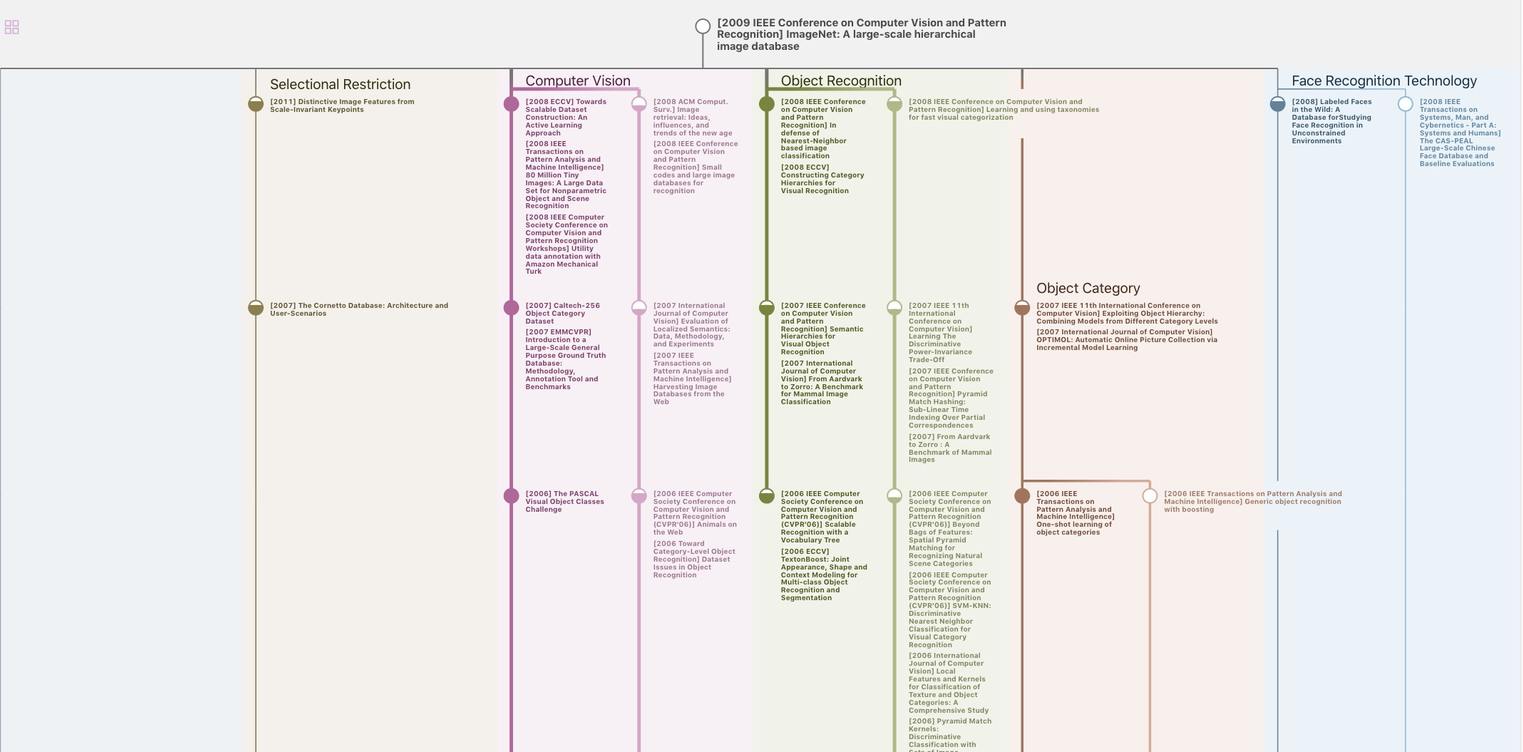
生成溯源树,研究论文发展脉络
Chat Paper
正在生成论文摘要